Dimension reduction-based adaptive-to-model semi-supervised classification
Statistical Papers(2024)
Abstract
This paper introduces a novel Dimension Reduction-based Adaptive-to-model Semi-supervised Classification method, specifically designed for scenarios where the number of unlabeled samples significantly exceeds that of labeled samples. Leveraging the strengths of sufficient dimension reduction and non-parametric interpolation, the method significantly amplifies the value derived from unlabeled samples, thus enhancing the precision of the classification model. An iterative version is also presented to extract further insights from the interpolated unlabeled samples. Theoretical analyses and numerical studies demonstrate substantial improvements in classifier accuracy, particularly in the context of model misspecified. The effectiveness of the proposed method in enhancing classification accuracy is further substantiated through two empirical analyses: credit card application evaluations and coronary heart disease diagnostic assessments.
MoreTranslated text
Key words
Sufficient dimension reduction,Model misspecified,Semi-supervised learning
AI Read Science
Must-Reading Tree
Example
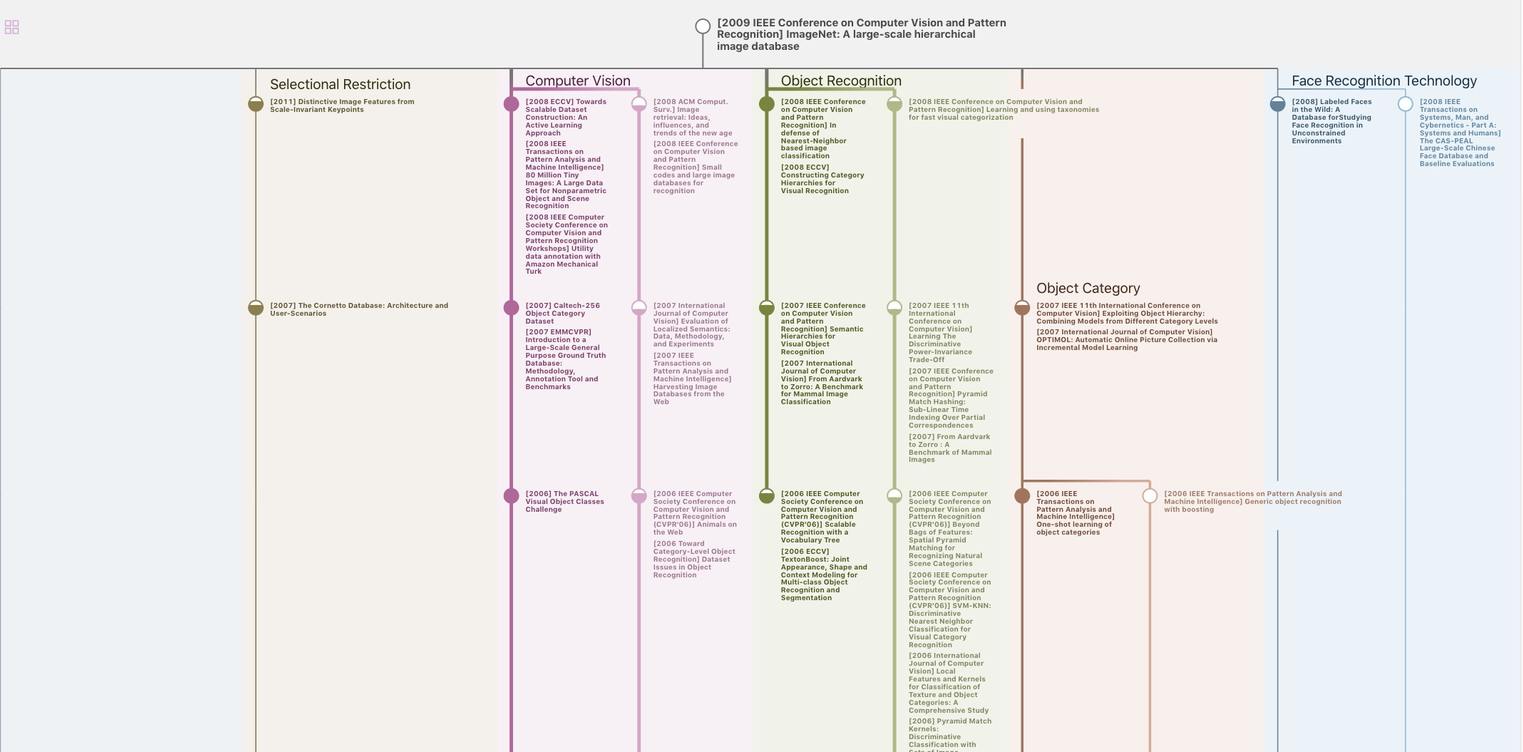
Generate MRT to find the research sequence of this paper
Chat Paper
Summary is being generated by the instructions you defined