Accurate Whole-Brain Image Enhancement for Low-Dose Integrated PET/MR Imaging Through Spatial Brain Transformation
IEEE Journal of Biomedical and Health Informatics(2024)
Abstract
Positron emission tomography/magnetic resonance imaging (PET/MRI) systems can provide precise anatomical and functional information with exceptional sensitivity and accuracy for neurological disorder detection. Nevertheless, the radiation exposure risks and economic costs of radiopharmaceuticals may pose significant burdens on patients. To mitigate image quality degradation during low-dose PET imaging, we proposed a novel 3D network equipped with a spatial brain transform (SBF) module for low-dose whole-brain PET and MR images to synthesize high-quality PET images. The FreeSurfer toolkit was applied to derive the spatial brain anatomical alignment information, which was then fused with low-dose PET and MR features through the SBF module. Moreover, several deep learning methods were employed as comparison measures to evaluate the model performance, with the peak signal-to-noise ratio (PSNR), structural similarity (SSIM) and Pearson correlation coefficient (PCC) serving as quantitative metrics. Both the visual results and quantitative results illustrated the effectiveness of our approach. The obtained PSNR and SSIM were
$41.96 \pm 4.91$ dB
(p<0.01) and
$0.9654 \pm 0.0215$
(p<0.01), which achieved a 19% and 20% improvement, respectively, compared to the original low-dose brain PET images. The volume of interest (VOI) analysis of brain regions such as the left thalamus (PCC = 0.959) also showed that the proposed method could achieve a more accurate standardized uptake value (SUV) distribution while preserving the details of brain structures. In future works, we hope to apply our method to other multimodal systems, such as PET/CT, to assist clinical brain disease diagnosis and treatment.
MoreTranslated text
Key words
Adaptive denoising network,low-dose PET imaging,spatial brain transform
AI Read Science
Must-Reading Tree
Example
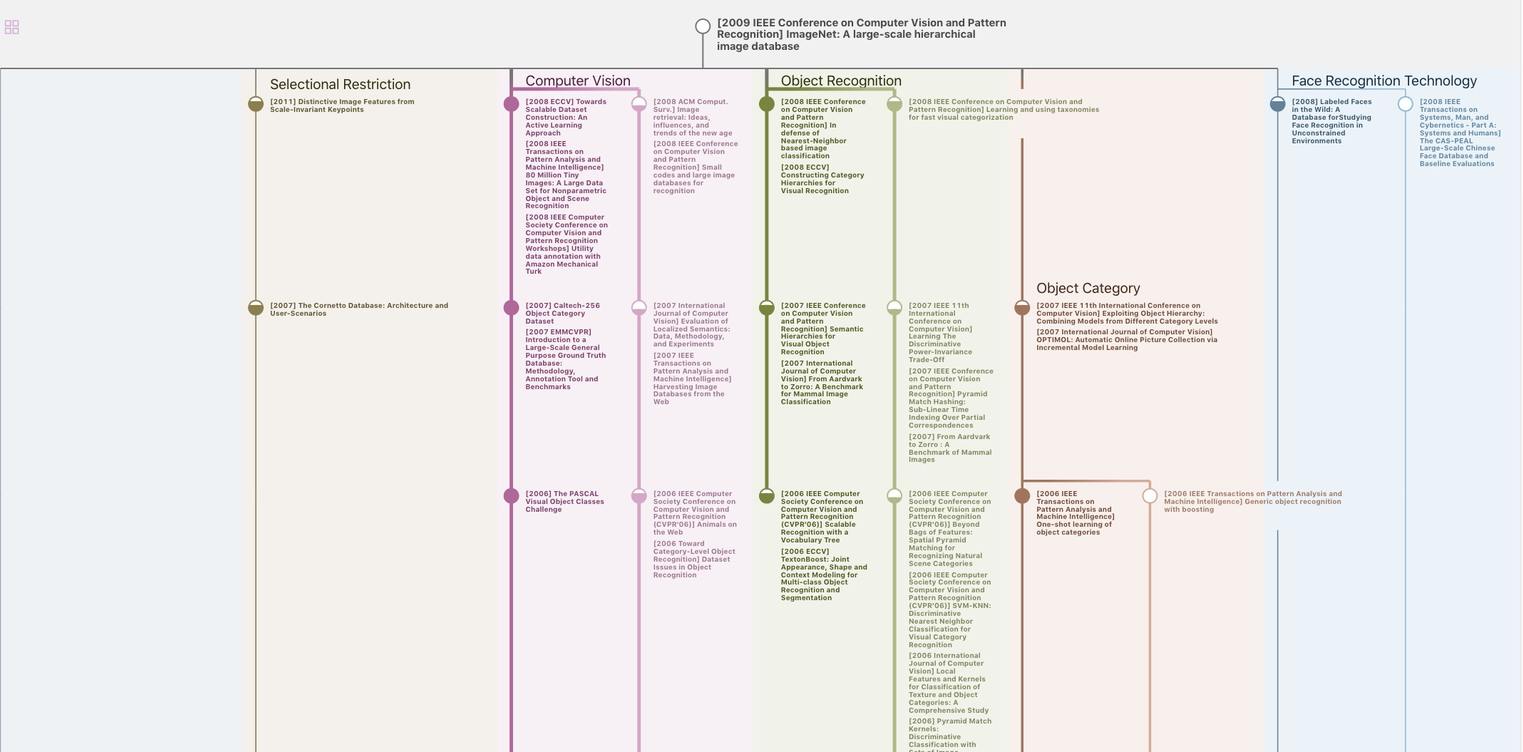
Generate MRT to find the research sequence of this paper
Chat Paper
Summary is being generated by the instructions you defined