IFKMHC: Implicit Fuzzy K-Means Model for High-Dimensional Data Clustering
IEEE TRANSACTIONS ON CYBERNETICS(2024)
摘要
The graph-information-based fuzzy clustering has shown promising results in various datasets. However, its performance is hindered when dealing with high-dimensional data due to challenges related to redundant information and sensitivity to the similarity matrix design. To address these limitations, this article proposes an implicit fuzzy k-means (FKMs) model that enhances graph-based fuzzy clustering for high-dimensional data. Instead of explicitly designing a similarity matrix, our approach leverages the fuzzy partition result obtained from the implicit FKMs model to generate an effective similarity matrix. We employ a projection-based technique to handle redundant information, eliminating the need for specific feature extraction methods. By formulating the fuzzy clustering model solely based on the similarity matrix derived from the membership matrix, we mitigate issues, such as dependence on initial values and random fluctuations in clustering results. This innovative approach significantly improves the competitiveness of graph-enhanced fuzzy clustering for high-dimensional data. We present an efficient iterative optimization algorithm for our model and demonstrate its effectiveness through theoretical analysis and experimental comparisons with other state-of-the-art methods, showcasing its superior performance.
更多查看译文
关键词
Feature extraction,High-dimensional data,Data models,Optimization,Manifolds,Image segmentation,Computational modeling,Fuzzy clustering,graph clustering,high-dimensional data,implicit model
AI 理解论文
溯源树
样例
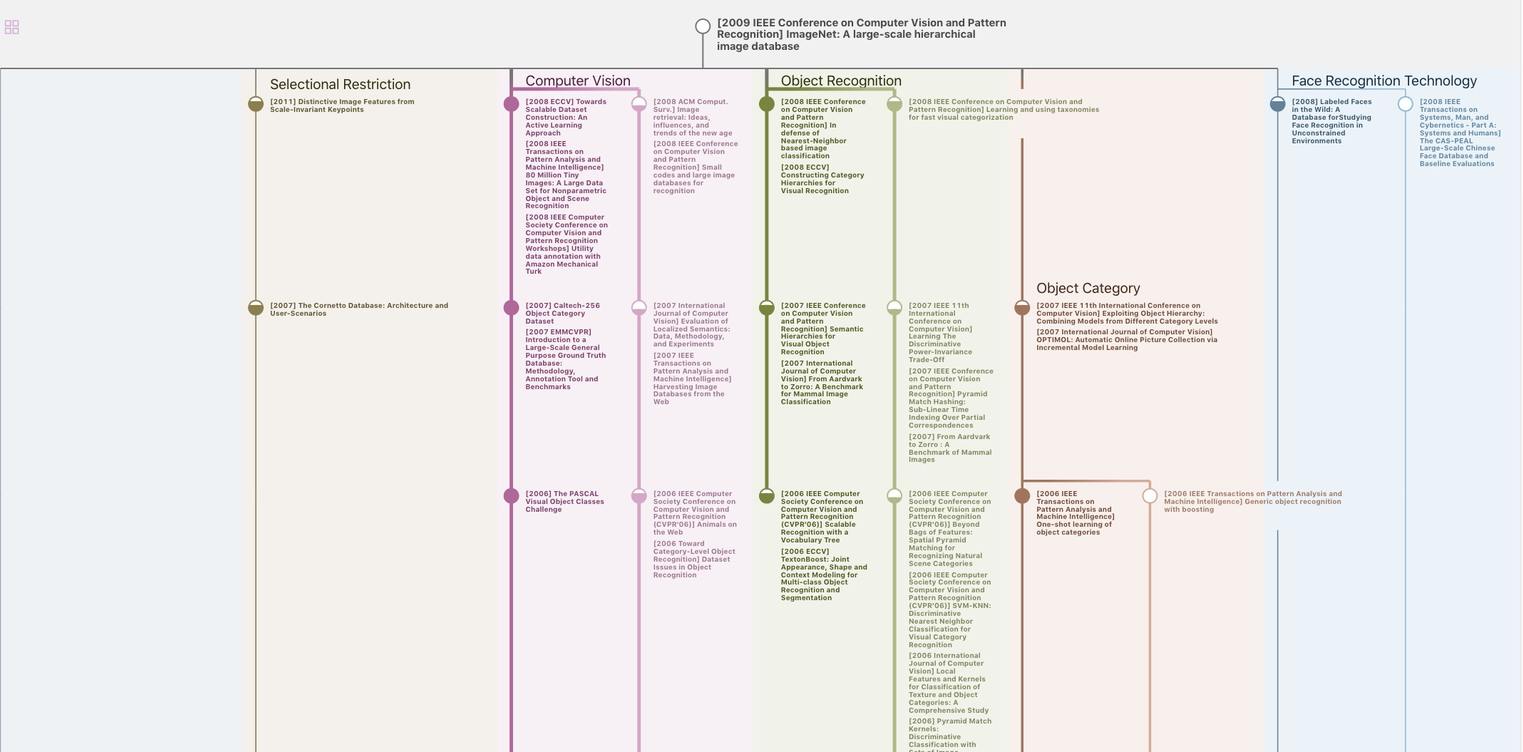
生成溯源树,研究论文发展脉络
Chat Paper
正在生成论文摘要