Identification of anoikis-related genes classification patterns and immune infiltration characterization in Chronic rhinosinusitis with nasal polyps based on machine learning
crossref(2024)
摘要
Introduction Chronic rhinosinusitis with nasal polyps (CRSwNP) is a subtype of chronic rhinosinusitis characterized by notable stromal edema, deposition of albumin, and formation of pseudocysts. Anoikis, a process in which cells detach from the correct extracellular matrix, disrupts integrin junctions, thereby inhibiting improperly proliferating cells from growing or adhering to an inappropriate matrix. Despite increasing evidence indicating that anoikis plays a role in regulating the immune response and significantly contributes to the development of CRSwNP, its specific role in the pathogenesis of CRSwNP remains largely unexplored. Methods At the outset, the GSE136825 and GSE179625 datasets were acquired from the NCBI GEO database, followed by extraction of 338 anoikis-related genes (ARGs) sourced from literature and databases. The CIBERSORT algorithm was employed to estimate the proportions of 22 infiltrating immune cell types. Subsequently, a consensus clustering approach was utilized to categorize CRSwNP samples. Key ARGs in CRSwNP were identified through the least absolute shrinkage and selection operator (LASSO), support vector machine-recursive feature elimination (SVM-RFE), and random forest (RF) algorithms. Receiver operating characteristics (ROC) analysis was conducted to evaluate the diagnostic accuracy of each identified gene. A diagnostic nomogram incorporating trait genes for CRSwNP was formulated. The association between gene expression and immune cell infiltration was analyzed in the combined dataset. Additionally, gene ontology (GO) and Kyoto Encyclopedia of Genes and Genomes (KEGG) analyses were performed to investigate differential signaling pathways and potential functions. Regulatory networks were constructed and depicted using NetworkAnalyst and Cytoscape. Finally, the findings of the bioinformatics analysis were validated preliminarily through the quantitative real-time reverse-transcription PCR (qRT-PCR), immunohistochemistry (IHC), and immunofluorescence (IF) at the human tissue level. Results Our study provides a comprehensive analysis of the role of ARGs in CRSwNP, representing a pioneering investigation in this field. We assessed the expression profile of ARGs in CRSwNP and their correlation with infiltrating immune cells. The results of our consensus clustering analysis revealed a potential classification of CRSwNP patients into two distinct clusters. Through machine learning analysis, we identified four key genes: CDH3, PTHLH, PDCD4, and AR. Additionally, we developed nomogram models based on these key genes and conducted assessments on the correlations of immune infiltration. The use of these key genes by Nomotu enabled the relatively accurate prediction of the likelihood of CRSwNP. Our findings indicated that 862 drugs or molecular compounds targeted AR. Lastly, our qRT-PCR, IHC, and IF analyses provided preliminary validation of our bioinformatics results, demonstrating a basic consistency between the two sets of findings. Conclusions We identified two anoikis-related clusters in CRSwNP and discovered four key genes associated with CRSwNP, in which AR was highly expressed in cluster 1 and PTHLH was highly expressed in cluster 2. These findings may provide new insights for drug screening, personalized therapy, and immunotherapy strategies for CRSwNP.
更多查看译文
AI 理解论文
溯源树
样例
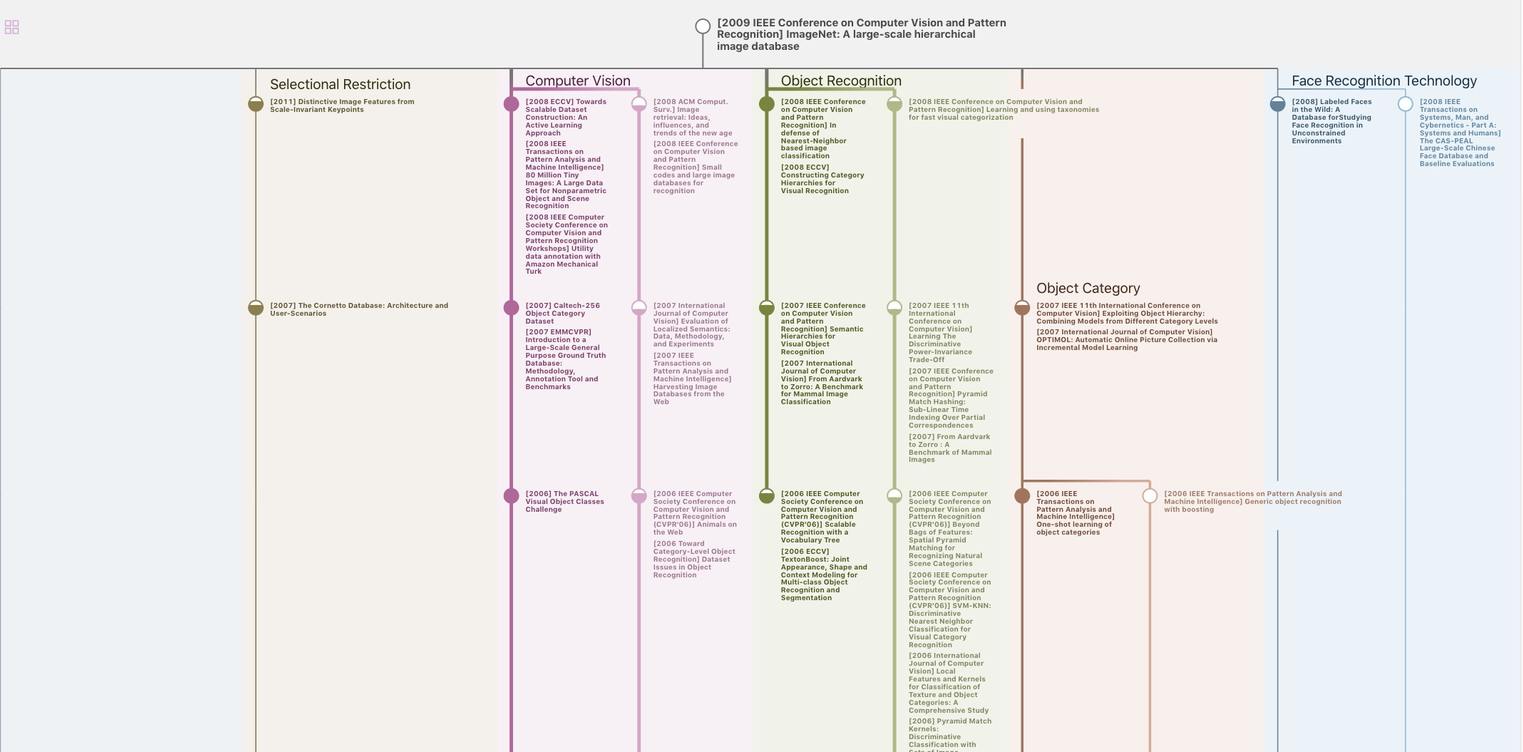
生成溯源树,研究论文发展脉络
Chat Paper
正在生成论文摘要