Efficient and Robust Sparse Linear Discriminant Analysis for Data Classification
IEEE Transactions on Emerging Topics in Computational Intelligence(2024)
Abstract
Sparse linear discriminant analysis (LDA) is a popular machine learning method that improves the accuracy of data classification by introducing sparsity. However, its performance often degrades seriously when encountering noise. To address this issue, this paper proposes a new method called efficient and robust sparse linear discriminant analysis (ERSLDA). The core idea is to characterize the local pixel corruptions by integrating
$L_{p}$
-norm
$(0< p< 1)$
, and to describe the global structured sparsity by enforcing
$L_{2,p}$
-norm
$(0< p< 1)$
, thereby improving the ability of feature selection. Compared with existing
$L_{1}$
-norm and
$L_{2,1}$
-norm, the involved
$L_{p}$
-norm and
$L_{2,p}$
-norm can bring higher robustness and better accuracy. Moreover, an additional matrix with Frobenius norm is embedded to represent Gaussian noise, which further enhances the robustness in different scenarios. In algorithms, an iterative optimization scheme is developed based on the alternating direction method of multipliers (ADMM) to solve the proposed ERSLDA, and the resulting subproblems can be calculated efficiently. Extensive numerical comparisons are performed with nine state-of-the-art LDA-based methods on seven benchmark image datasets. The experimental results validate that the proposed method is efficient for data classification and robust to noise.
MoreTranslated text
Key words
Data classification,linear discriminant analysis (LDA),$L_{p}$ -norm,optimization algorithm
AI Read Science
Must-Reading Tree
Example
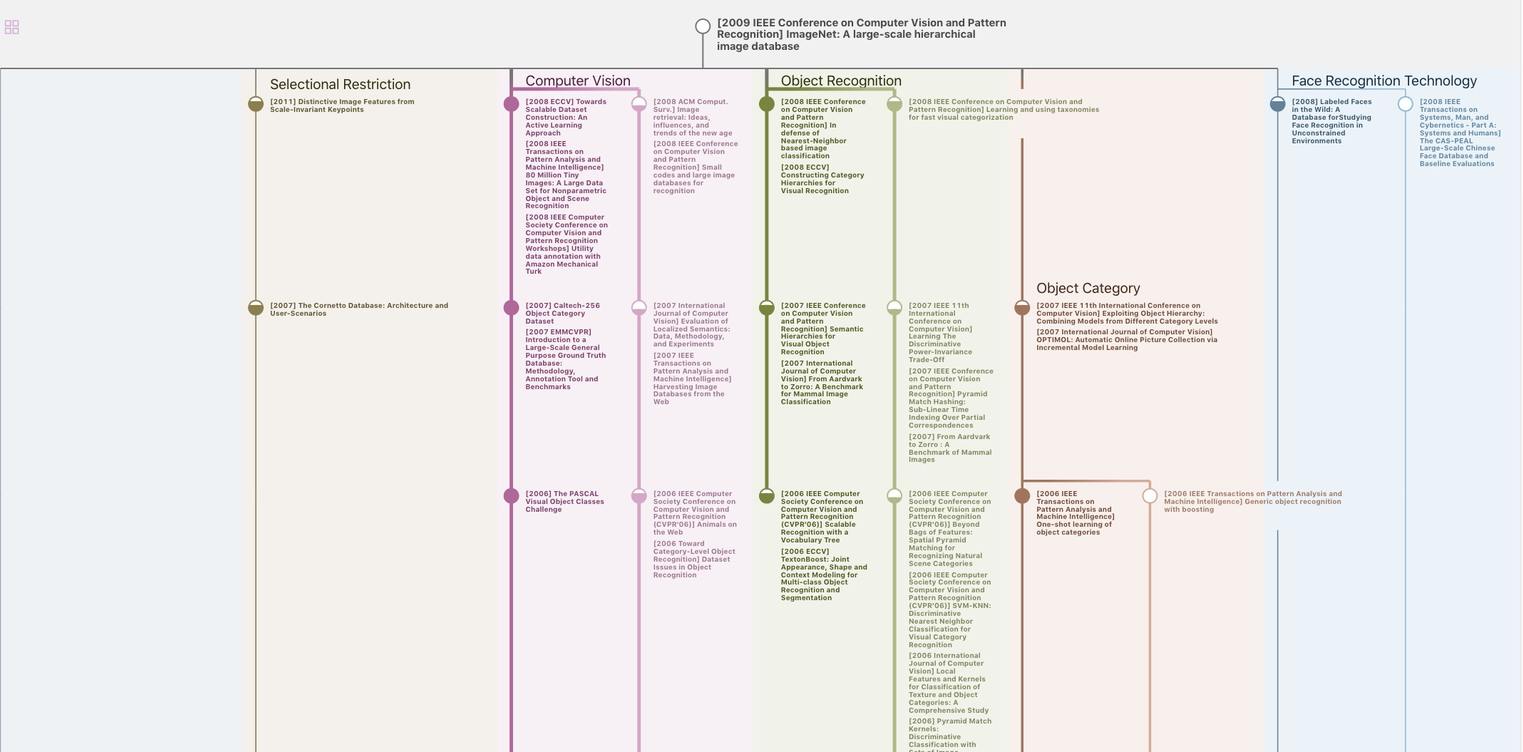
Generate MRT to find the research sequence of this paper
Chat Paper
Summary is being generated by the instructions you defined