LAM-Depth: Laplace-Attention Module-Based Self-Supervised Monocular Depth Estimation
IEEE Transactions on Intelligent Transportation Systems(2024)
Abstract
Depth estimation is extremely important in the world of driverless vehicles, which can provide key distance information for 3D scene perception and local path planning. Using convolutional neural network (CNN) to completely recover depth information from monocular images becomes a hot research trend. Supervised monocular depth estimation needs large numbers of per-pixel ground-truth depth data collected from LiDAR to train the model. This leads to high resource consumption and poor generalization ability. For the above reasons, the self-supervised learning method seems to be a promising alternative to monocular depth estimation. However, the up-sampling operations in existing encoder-decoder based architecture may lose some critical image information, which leads to boundary blurring and depth artifact in depth maps. In this paper, the Laplace-Attention module based self-supervised monocular depth estimation network (LAM-Depth) is designed to resolve this problem. Specifically, multi-scale Laplacian features are introduced into the corresponding streams in the decoder to fuse the low-level and skip-connection features. The concatenated features are then re-calibrated with a channel-wise attention unit for emphasizing the Laplacian features. Based on the above operations, the image information is preserved to the greatest extent in feature processing. The experiment results show that the proposed model LAM-Depth achieves a high ranking among the existing unsupervised methods and outperforms several supervised models trained with LiDAR data. Furthermore, we conduct experiments in real scenes to evaluate the generalization ability of LAM-Depth and obtain high-quality depth maps.
MoreTranslated text
Key words
Scene perception,monocular depth estimation,self-supervised,attention unit
AI Read Science
Must-Reading Tree
Example
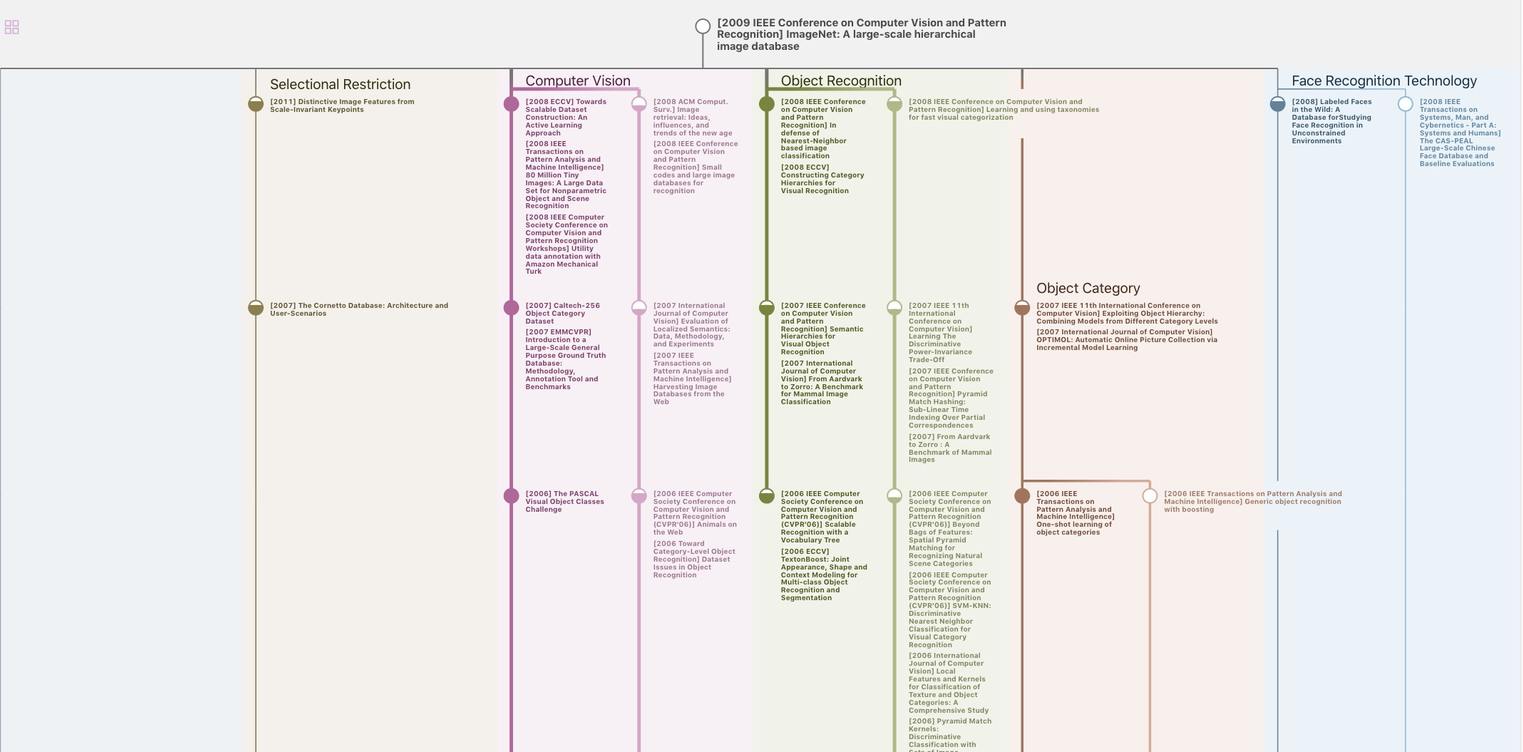
Generate MRT to find the research sequence of this paper
Chat Paper
Summary is being generated by the instructions you defined