Diagnosis of Parkinson Disease from EEG Signals Using a CNN-LSTM Model and Explainable AI.
International Work-Conference on the Interplay Between Natural and Artificial Computation(2024)
摘要
Parkinson’s disease (PD), a complex and debilitating neurological disorder, often leads to progressive cognitive decline, including mild cognitive impairment (MCI) and dementia. Over the years, various methods have been developed to diagnose PD, with neuroimaging modalities, particularly electroencephalogram (EEG) recording, gaining significant traction among specialist doctors. This article presents a novel PD detection method employing deep learning (DL) techniques to analyze EEG signals. The proposed method utilizes the UC San Diego (UCSD) resting-state EEG dataset and involves a meticulous preprocessing phase encompassing filtering, channel selection, and EEG signal windowing. Subsequently, a novel 1D CNN-LSTM architecture is introduced for extracting salient features from EEG signals. In the classification stage, three algorithms, namely Softmax, support vector machine (SVM), and decision tree (DT), are employed and their performances compared. To assess the robustness of the classification models, k-fold cross-validation with k=10 is implemented. The results demonstrate that the SVM algorithm exhibits superior performance, achieving an impressive 99.51 % accuracy for binary classification and 99.75 % accuracy for multi-class classification tasks. To gain insights into the model’s decision-making process and enhance interpretability, t-distributed Stochastic Neighbor Embedding (t-SNE) is utilized as an explainable artificial intelligence (XAI) method in the post-processing stage.
更多查看译文
AI 理解论文
溯源树
样例
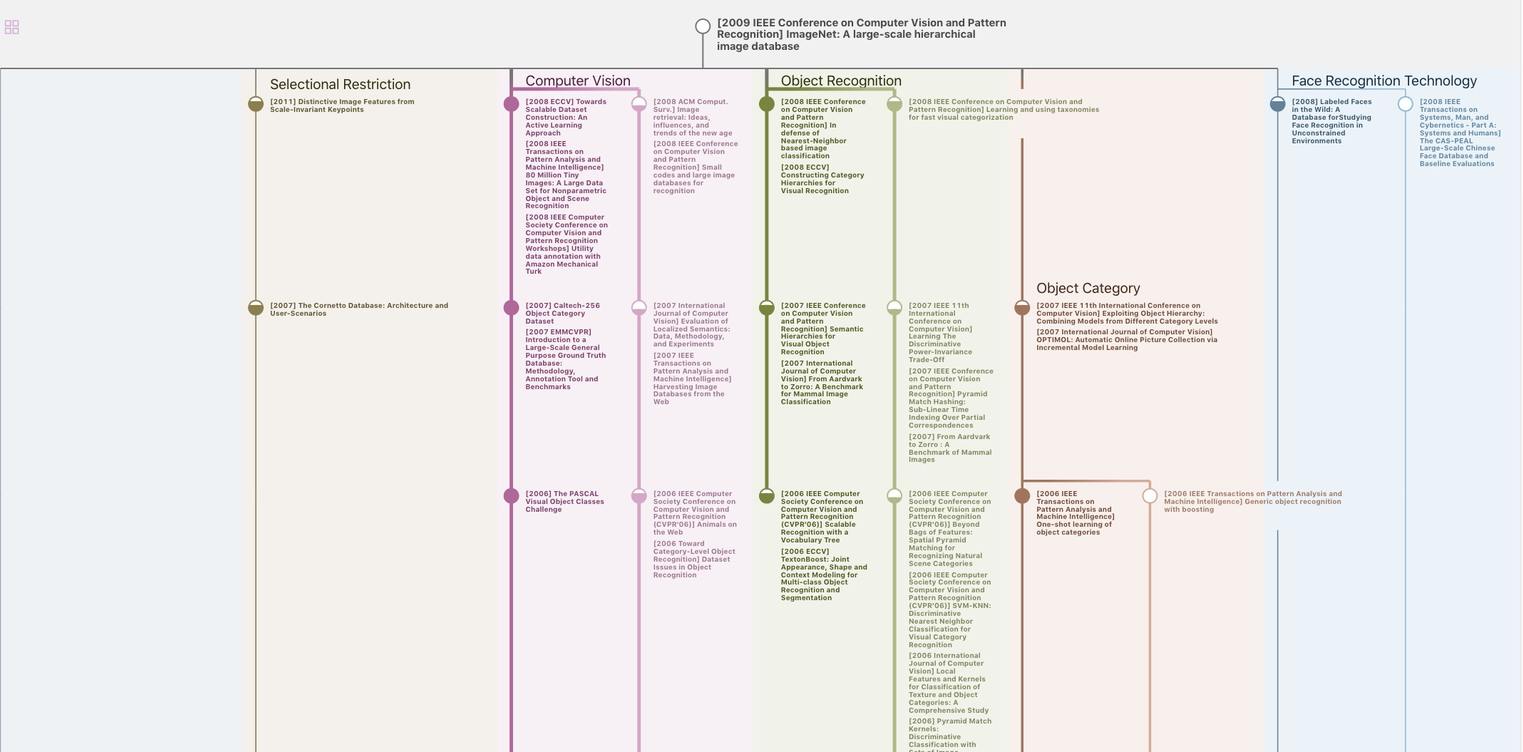
生成溯源树,研究论文发展脉络
Chat Paper
正在生成论文摘要