GaussianPrediction: Dynamic 3D Gaussian Prediction for Motion Extrapolation and Free View Synthesis
SIGGRAPH '24 ACM SIGGRAPH 2024 Conference Papers(2024)
摘要
Forecasting future scenarios in dynamic environments is essential forintelligent decision-making and navigation, a challenge yet to be fullyrealized in computer vision and robotics. Traditional approaches like videoprediction and novel-view synthesis either lack the ability to forecast fromarbitrary viewpoints or to predict temporal dynamics. In this paper, weintroduce GaussianPrediction, a novel framework that empowers 3D Gaussianrepresentations with dynamic scene modeling and future scenario synthesis indynamic environments. GaussianPrediction can forecast future states from anyviewpoint, using video observations of dynamic scenes. To this end, we firstpropose a 3D Gaussian canonical space with deformation modeling to capture theappearance and geometry of dynamic scenes, and integrate the lifecycle propertyinto Gaussians for irreversible deformations. To make the prediction feasibleand efficient, a concentric motion distillation approach is developed bydistilling the scene motion with key points. Finally, a Graph ConvolutionalNetwork is employed to predict the motions of key points, enabling therendering of photorealistic images of future scenarios. Our framework showsoutstanding performance on both synthetic and real-world datasets,demonstrating its efficacy in predicting and rendering future environments.
更多查看译文
AI 理解论文
溯源树
样例
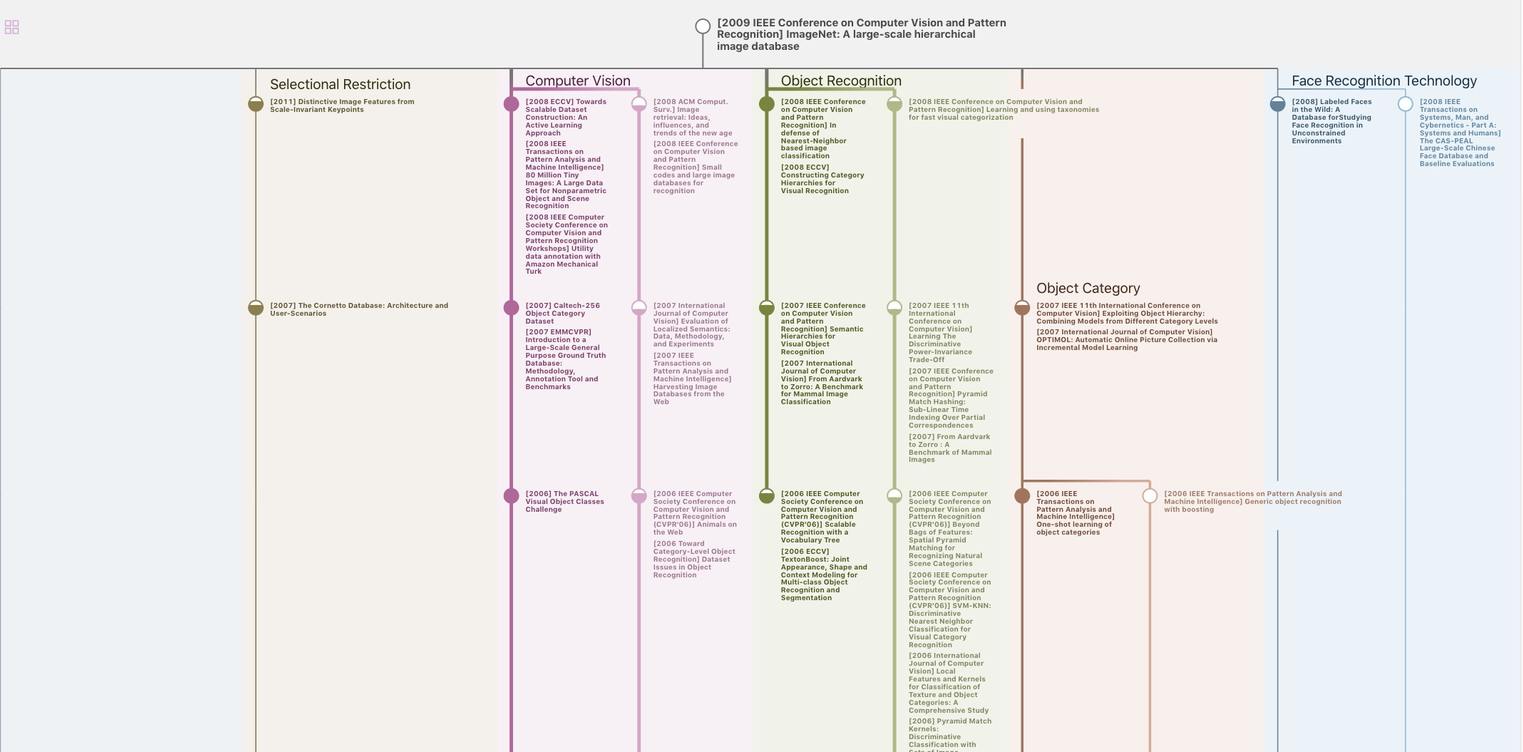
生成溯源树,研究论文发展脉络
Chat Paper
正在生成论文摘要