HINT: Learning Complete Human Neural Representations from Limited Viewpoints
CoRR(2024)
Abstract
No augmented application is possible without animated humanoid avatars. At
the same time, generating human replicas from real-world monocular hand-held or
robotic sensor setups is challenging due to the limited availability of views.
Previous work showed the feasibility of virtual avatars but required the
presence of 360 degree views of the targeted subject. To address this issue, we
propose HINT, a NeRF-based algorithm able to learn a detailed and complete
human model from limited viewing angles. We achieve this by introducing a
symmetry prior, regularization constraints, and training cues from large human
datasets. In particular, we introduce a sagittal plane symmetry prior to the
appearance of the human, directly supervise the density function of the human
model using explicit 3D body modeling, and leverage a co-learned human
digitization network as additional supervision for the unseen angles. As a
result, our method can reconstruct complete humans even from a few viewing
angles, increasing performance by more than 15
state-of-the-art algorithms.
MoreTranslated text
Key words
Human Model,Viewing Angle,Explicit Model,Regularization Constraint,Model Parameters,Real-world Data,Pedestrian,Realistic Model,Multilayer Perceptron,Color Space,Video Sequences,Robotic Applications,Depth Estimation,Objects In The Scene,Color Appearance,Pre-trained Weights,Limited View,Dynamic Scenes,Canonical Representation,HSV Color,View Synthesis,Loss Of Symmetry
AI Read Science
Must-Reading Tree
Example
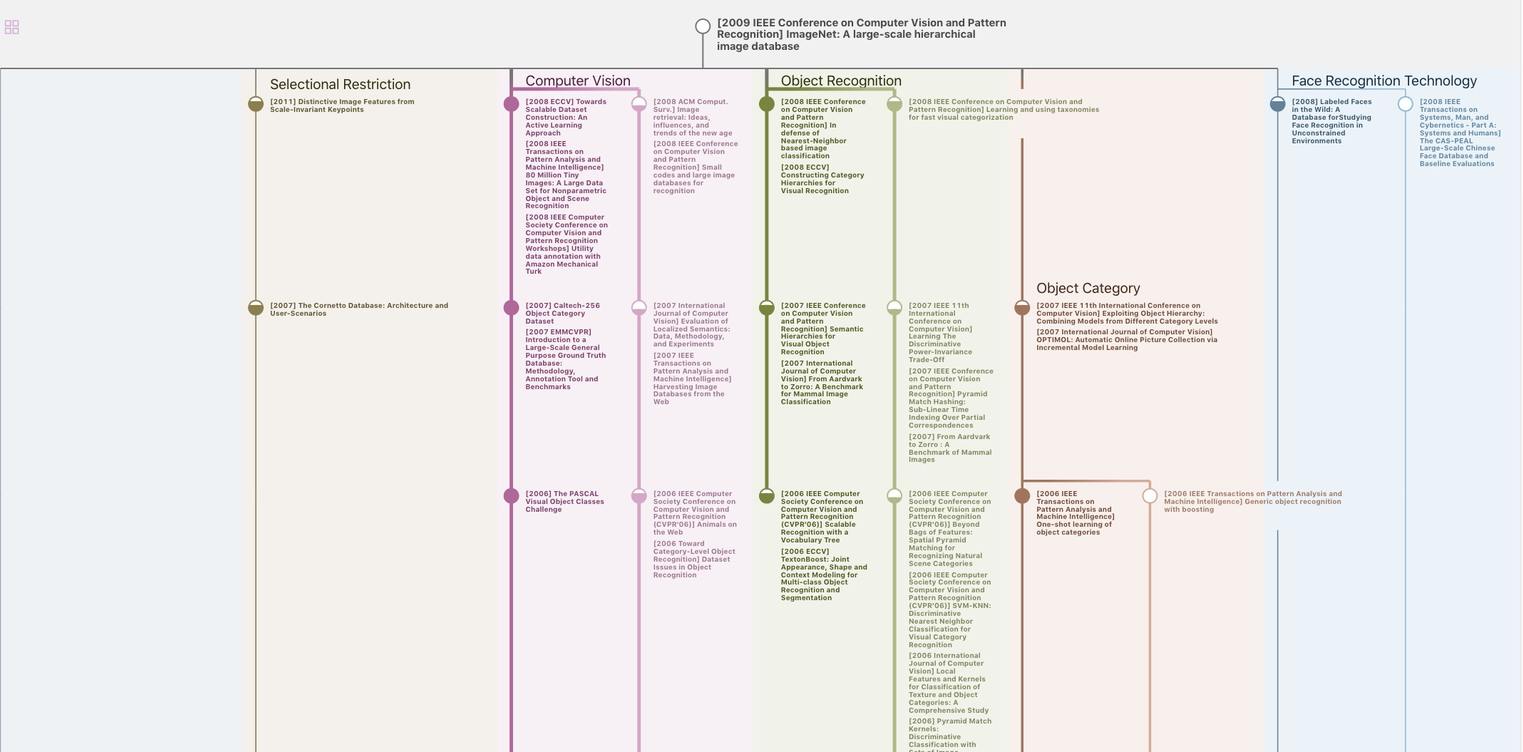
Generate MRT to find the research sequence of this paper
Chat Paper
Summary is being generated by the instructions you defined