Critical Learning Periods: Leveraging Early Training Dynamics for Efficient Data Pruning
CoRR(2024)
Abstract
Neural Machine Translation models are extremely data and compute-hungry.
However, not all data points contribute equally to model training and
generalization. Data pruning to remove the low-value data points has the
benefit of drastically reducing the compute budget without significant drop in
model performance. In this paper, we propose a new data pruning technique:
Checkpoints Across Time (CAT), that leverages early model training dynamics to
identify the most relevant data points for model performance. We benchmark CAT
against several data pruning techniques including COMET-QE, LASER and LaBSE. We
find that CAT outperforms the benchmarks on Indo-European languages on multiple
test sets. When applied to English-German, English-French and English-Swahili
translation tasks, CAT achieves comparable performance to using the full
dataset, while pruning up to 50
that CAT selects and find that it tends to favour longer sentences and
sentences with unique or rare words.
MoreTranslated text
AI Read Science
Must-Reading Tree
Example
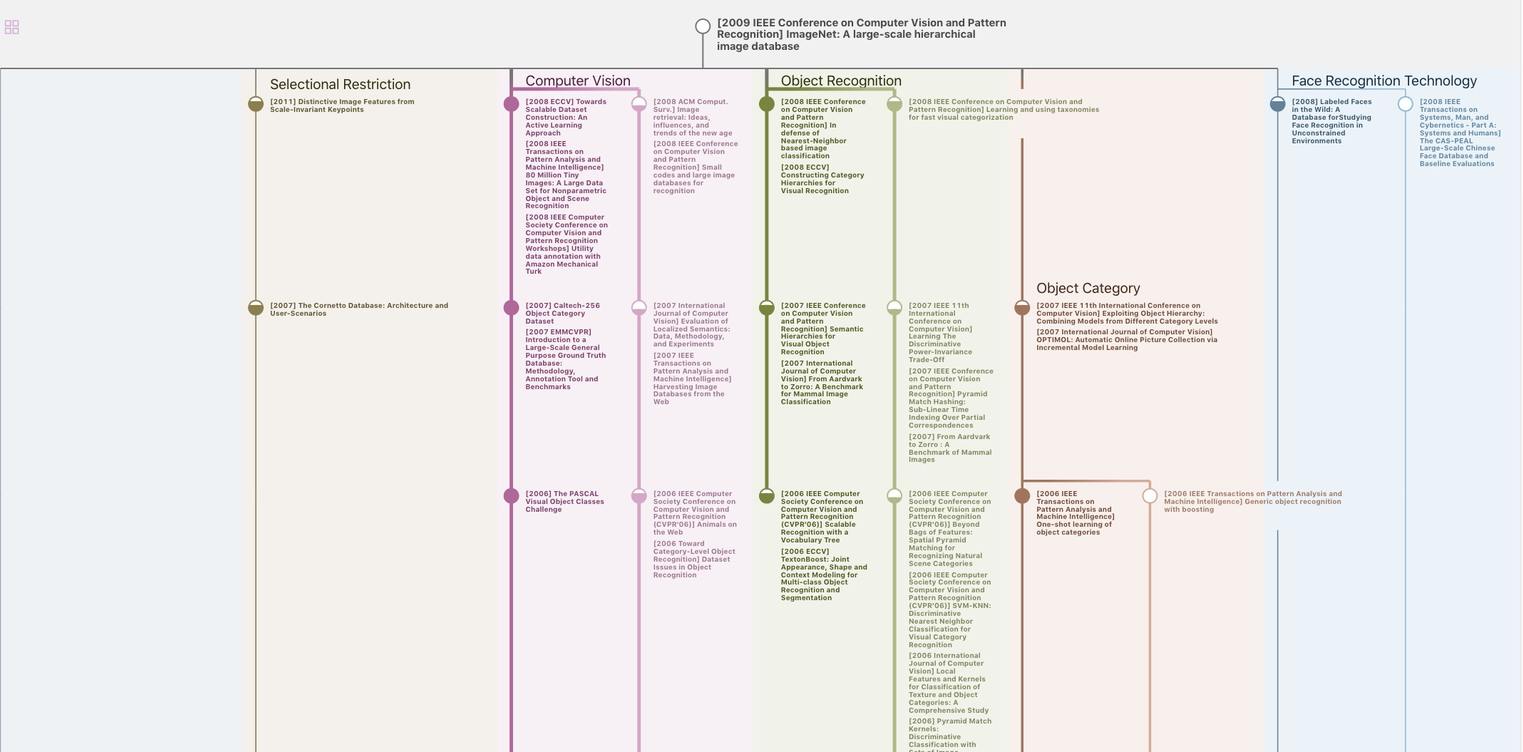
Generate MRT to find the research sequence of this paper
Chat Paper
Summary is being generated by the instructions you defined