ULG-SLAM: A Novel Unsupervised Learning and Geometric Feature-Based Visual SLAM Algorithm for Robot Localizability Estimation
Remote Sensing(2024)
摘要
Indoor localization has long been a challenging task due to the complexity and dynamism of indoor environments. This paper proposes ULG-SLAM, a novel unsupervised learning and geometric-based visual SLAM algorithm for robot localizability estimation to improve the accuracy and robustness of visual SLAM. Firstly, a dynamic feature filtering based on unsupervised learning and moving consistency checks is developed to eliminate the features of dynamic objects. Secondly, an improved line feature extraction algorithm based on LSD is proposed to optimize the effect of geometric feature extraction. Thirdly, geometric features are used to optimize localizability estimation, and an adaptive weight model and attention mechanism are built using the method of region delimitation and region growth. Finally, to verify the effectiveness and robustness of localizability estimation, multiple indoor experiments using the EuRoC dataset and TUM RGB-D dataset are conducted. Compared with ORBSLAM2, the experimental results demonstrate that absolute trajectory accuracy can be improved by 95% for equivalent processing speed in walking sequences. In fr3/walking_xyz and fr3/walking_half, ULG-SLAM tracks more trajectories than DS-SLAM, and the ATE RMSE is improved by 36% and 6%, respectively. Furthermore, the improvement in robot localizability over DynaSLAM is noteworthy, coming in at about 11% and 3%, respectively.
更多查看译文
关键词
visual SLAM,unsupervised learning,geometric feature,robot localizability estimation
AI 理解论文
溯源树
样例
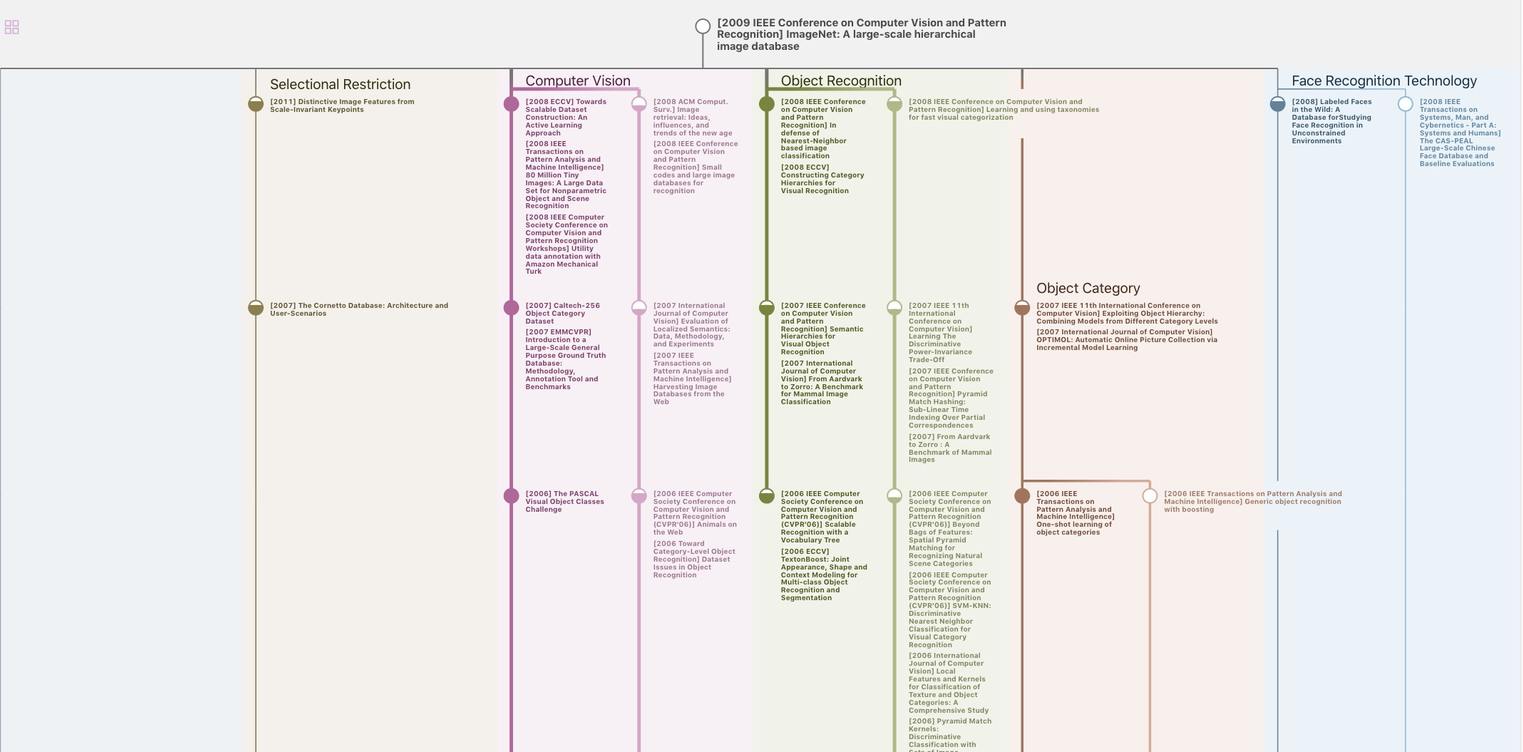
生成溯源树,研究论文发展脉络
Chat Paper
正在生成论文摘要