Rapport Prediction Using Pairwise Learning in Dyadic Conversations Among Strangers and Among Friends.
International Conference on Human-Computer Interaction(2024)
Abstract
Automatic rapport prediction is a key component in the creation of socially aware conversational agents. In our study, we aim to automatically predict a speakers’ subjective rapport using their nonverbal (acoustic and facial) cues during conversations. While cues indicating rapport vary according to social relations between speakers, few studies have investigated an effective modality or combination of modalities for predicting subjective rapport. To fill this research gap, we collected both first-meeting (FM) and friend (FR) conversations from the same participants. Then, we addressed predicting subjective rapport using a common framework in both FM and FR conversations. Predicting subjective rapport is often formulated as a regression task that directly predicts rapport ratings. However, regression is not a suitable approach because it does not consider individual differences and ambiguity in subjective ratings. Thus, we adopted pairwise learning (PL). PL overcomes individual differences and ambiguity in subjective ratings because PL does not directly use rapport ratings. Our experimental results showed that PL is a more appropriate approach than regression for predicting conversations in both FM and FR conversations. We also reported an effective modality or combination of modalities for predicting subjective rapport in FM and FR conversations, respectively.
MoreTranslated text
AI Read Science
Must-Reading Tree
Example
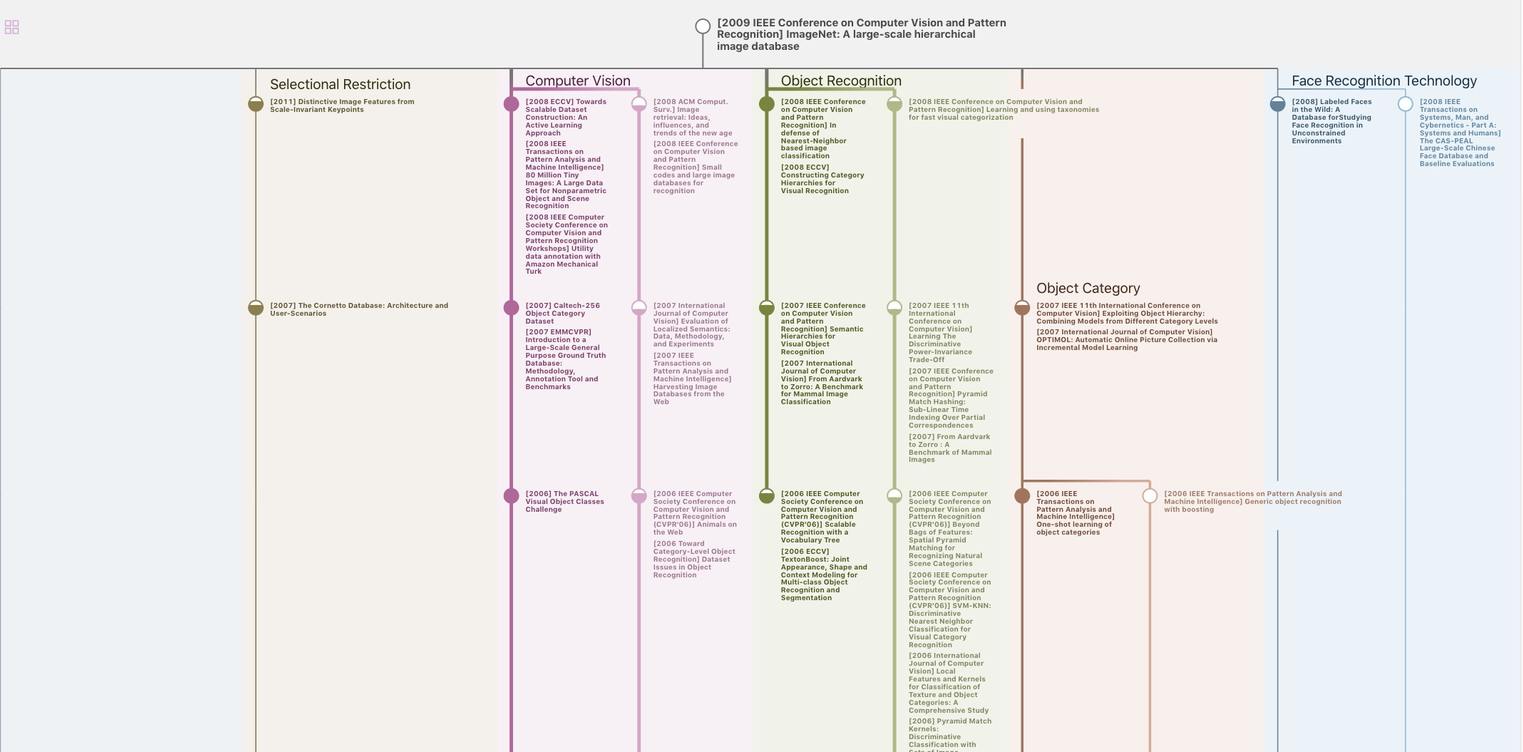
Generate MRT to find the research sequence of this paper
Chat Paper
Summary is being generated by the instructions you defined