Efficient tick-shape networks of full-residual point-depth-point blocks for image classification
Neurocomputing(2024)
Abstract
Light-weight convolutional neural networks (CNNs) are crucial for deploying computer vision applications in mobile devices thanks to their compact models, small computational complexity, and energy efficiency. However, such models ordinarily have steady-increased channels in their backbone leading to a sharp increase in the model size; while the deficiency of identity mappings in their residual mechanism can lead to modest performance in feature extraction. To mitigate those issues, we propose light-weight networks based on three novel concepts as follows. Firstly, an efficient perceptron is presented to encapsulate point-depth-point (PDP) features extracted by light-weight convolutions along with a full-residual (FR) mechanism through the architecture of a network. This full-residual connection is proposed to deal with the shortcoming of the existing light-weight models whose architecture has incompletely exploited identity mappings. It is due to the variability of spatial dimension caused by several strides of their convolutional operations. Secondly, a tick-shape backbone is then introduced by designing its structure in accordance with the channel elasticity of the FR-PDP perceptron subject to the shape of a check mark. Thirdly, taking advantage of the channel elasticity concept, three tick-shape networks (TickNets) are constructed in a light-weight architecture by hooking one or more tick-shape backbones. Experimental results for image classification on benchmark datasets have clearly corroborated the efficacy of the proposed methods. The code implementation of tick-shape models is available at https://github.com/nttbdrk25/TickNets.
MoreTranslated text
Key words
Light-weight models,Depthwise/pointwise convolutions,CNN-based networks,Full-residual mechanism,Image classification
AI Read Science
Must-Reading Tree
Example
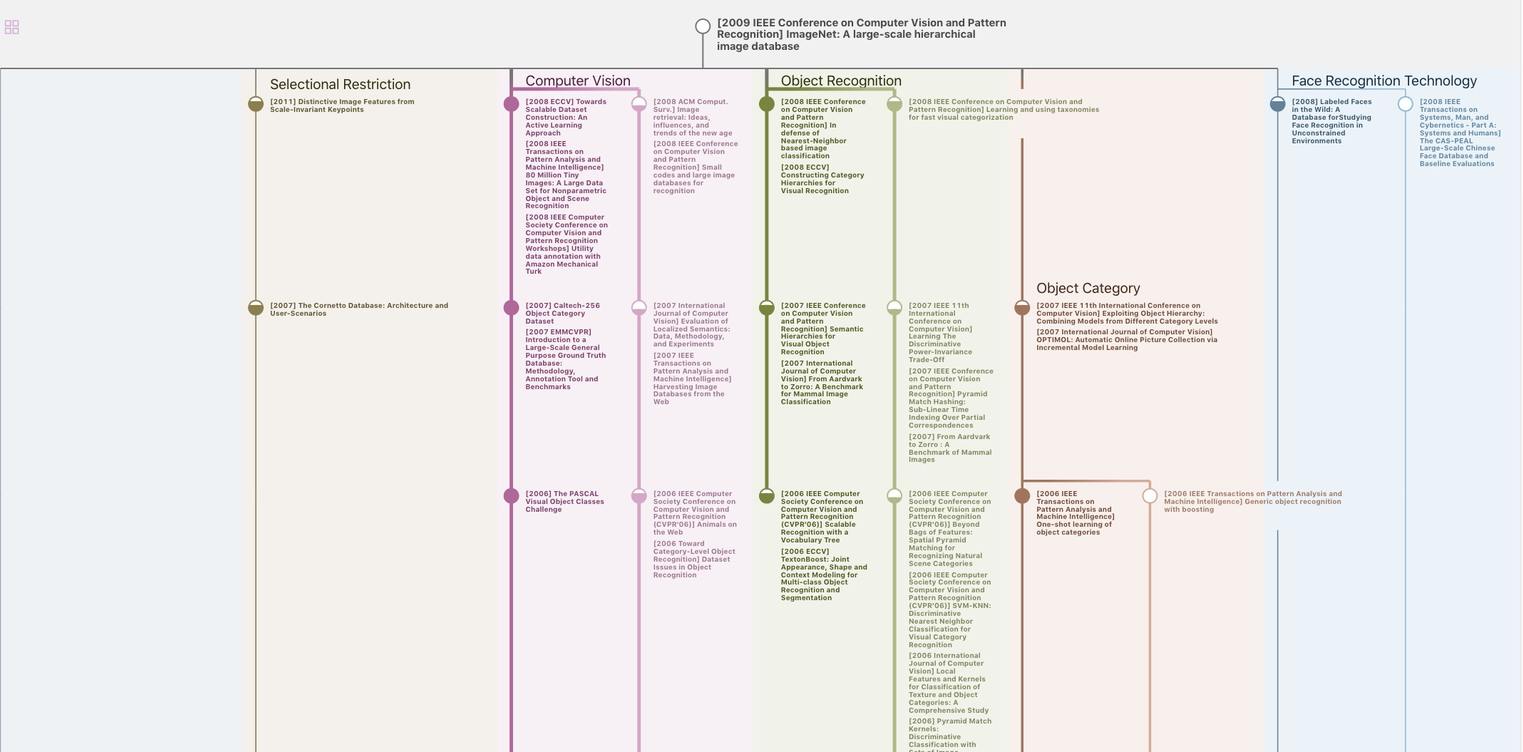
Generate MRT to find the research sequence of this paper
Chat Paper
Summary is being generated by the instructions you defined