An explainable dual-mode convolutional neural network for multivariate time series classification
Knowledge-Based Systems(2024)
摘要
Multivariate time series classification (MTSC) is a crucial machine learning problem prevalent across various real-life domains. Traditional deep learning approaches with high accuracy in MTSC are often criticized for their “black box” nature, offering no insight into their operational mechanisms or decision-making processes. Explainable artificial intelligence (XAI) becomes a key idea for dealing with such limitations in decision-sensitive areas. While some researchers have explored the interpretability of MTSC, the majority have focused on elucidating the relationship between variables over time, neglecting the intricate connections among different variables. To address the interpretability issue in MTSC, we introduce an explainable dual-mode convolutional neural network (XDM-CNN) designed specifically for MTSC. The proposed XDM-CNN framework comprises two modules: a classification module and an explanation module. The classification module can ensure exceptional classification performance by combining both one-dimensional and two-dimensional convolutional neural networks, while the explanation module can mine the underlying logical relationships among variables during the classification process by utilizing a synergy of visualization and quantification techniques. We validate our approach using 26 datasets sourced from the University of East Anglia (UEA) archive. The experimental results demonstrate that XDM-CNN not only exhibits excellent performance in classification accuracy, but also has strong explainability. By combining visual and numerical explanation, the hidden logical relationships among multivariate time series are explored and interpreted, providing human users with a decidable basis for classification decisions.
更多查看译文
关键词
Explainable convolutional neural network,Multivariate time series classification,Dual-mode,Time-frequency domain,Visual and numerical explanation
AI 理解论文
溯源树
样例
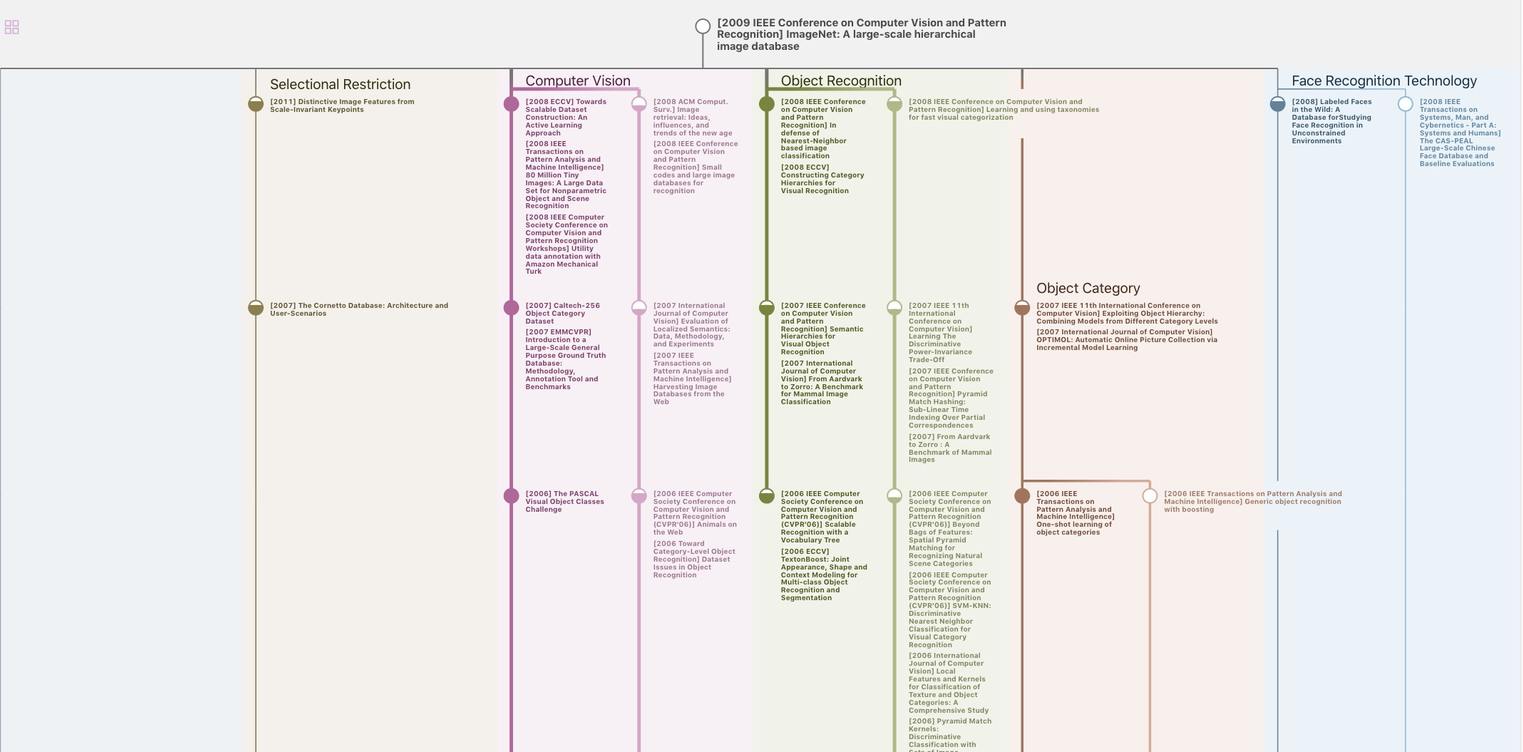
生成溯源树,研究论文发展脉络
Chat Paper
正在生成论文摘要