Shared Latent Membership Enables Joint Shape Abstraction and Segmentation With Deformable Superquadrics.
IEEE transactions on image processing : a publication of the IEEE Signal Processing Society(2024)
Abstract
Part-level 3D shape representations are crucial to shape reasoning and understanding. Two key sub-tasks are: 1) shape abstraction, creating primitive-based object parts; and 2) shape segmentation, finding partition-based object parts. However, for 3D object point clouds, most advanced methods produce parts relying on task-specific priors, such as similarity metrics and primitive geometries, resulting in misleading parts that deviate from semantics. To address prior limitations, we establish a foundation for joint shape abstraction and shape segmentation as formal linear transformations within a shared latent space, encapsulating essential dual-purpose membership information linking points and object parts for mutual reinforcement. We demonstrate that the transformations are underpinned by a derivation based on k-means, Non-negative Matrix Factorization (NMF), and the attention mechanism. As a result, we introduce Latent Membership Pursuit (LMP) for joint optimization of shape abstraction and segmentation. LMP utilizes a shared latent representation of object part membership to autonomously identify common object parts in both tasks without any supervision and priors. Furthermore, we adapt deformable superquadrics (DSQs) for primitives to capture variable part-level geometric and semantic information. Experiments on benchmark datasets validate that our approach enables mutual learning of shape abstraction and segmentation, and promotes consistent interpretations of 3D object shapes across instances and even categories in a fully unsupervised manner.
MoreTranslated text
Key words
Point cloud,shape abstraction,segmentation,unsupervised learning,joint task
AI Read Science
Must-Reading Tree
Example
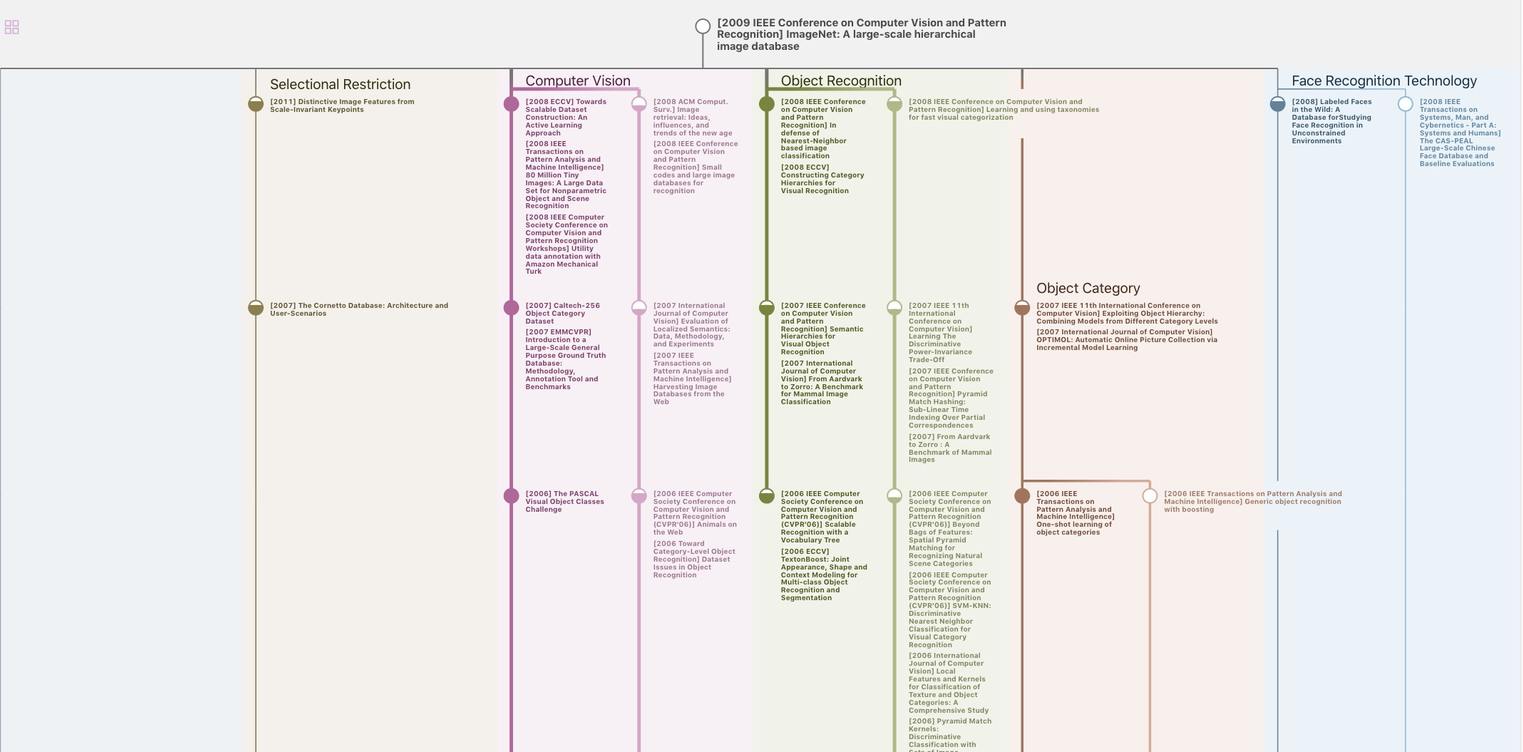
Generate MRT to find the research sequence of this paper
Chat Paper
Summary is being generated by the instructions you defined