Predictive monitoring of built thermal environment using limited sensor data: A deep learning-based spatiotemporal method
Sustainable Energy Technologies and Assessments(2024)
Abstract
Spatiotemporal monitoring of the built thermal environment plays an important role in promoting building thermal and energy management for development of sustainable buildings. However, the limited number of sensors and the uncertain airflow dynamics increase the difficulty for monitoring the nonuniformly distributed temperature states in the built thermal environment. In this study, a deep learning based predictive monitoring framework is proposed for spatiotemporal reconstruction and uncertainty quantification of the high-dimensional airflow fields in built environment based on limited sensors. The monitoring framework (iCVAE-BiLSTM) is developed by combining improved Conditional Variational Auto-Encoder (iCVAE), Bi-directional Long Short-Term Memory Network (BiLSTM) and Monte Carlo Simulation (MCS). Specifically, iCVAE is used in combination with BiLSTM model to model the spatiotemporal variations of the distributed indoor temperature states, while MCS is applied to quantify the uncertainties in temperature variations. A case study is carried out in a confined space under mixing convection for validation of the iCVAE-BiLSTM based monitoring framework. Moreover, the effects of sensor numbers and sensor placement on monitoring accuracy are quantitatively investigated. Our study demonstrates that the proposed iCVAE-BiLSTM model has significant application potentials for monitoring built environment and for promoting the development of energy-efficient buildings.
MoreTranslated text
Key words
Built environment,Predictive monitoring,Sensors,Spatiotemporal reconstruction,Deep learning
AI Read Science
Must-Reading Tree
Example
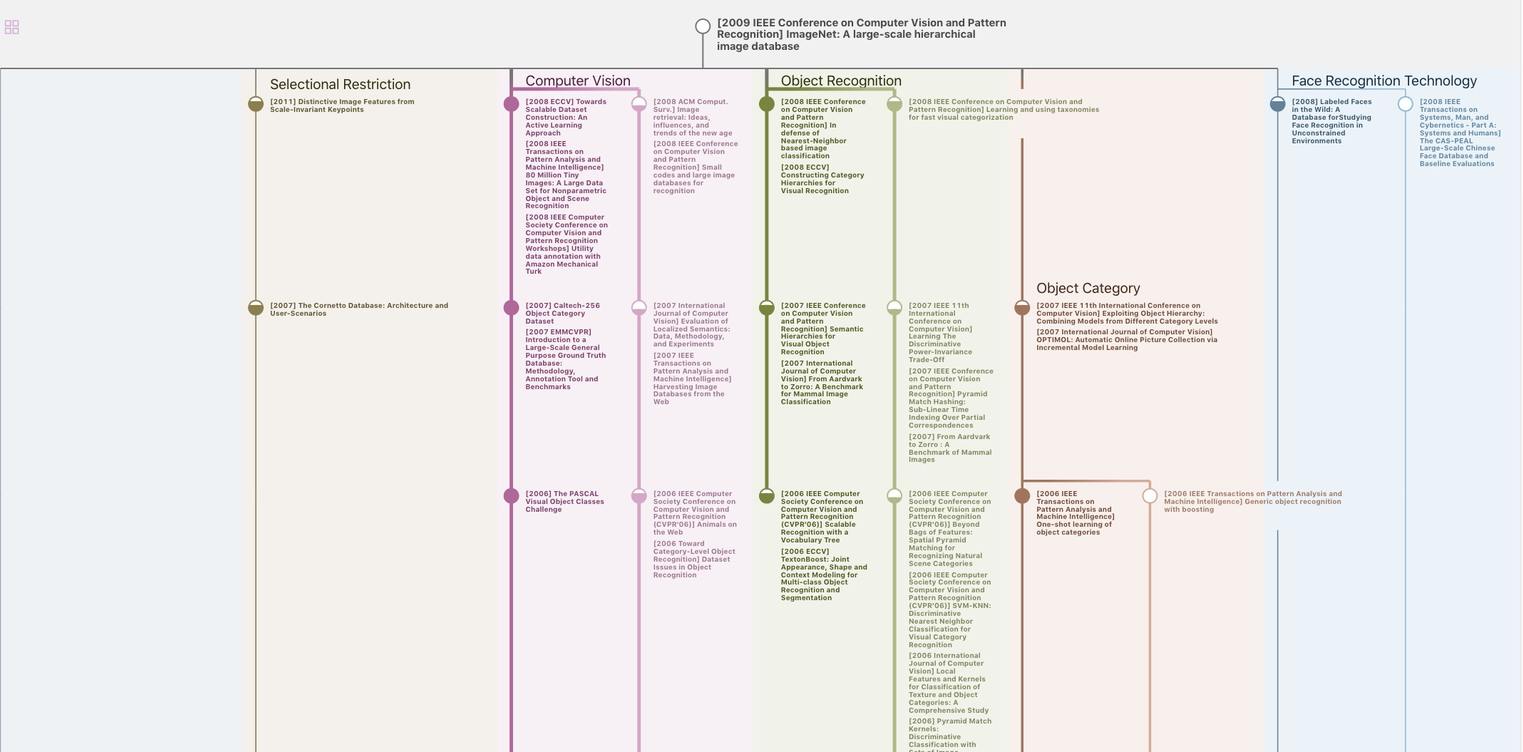
Generate MRT to find the research sequence of this paper
Chat Paper
Summary is being generated by the instructions you defined