Causal dilated Convolution-Based residual DenseNet with channel attention for RUL prediction of rolling bearings
Measurement(2024)
摘要
The remaining useful life (RUL) prediction technology of rolling bearings plays an essential role in preventing major accidents and implementing predictive maintenance. Therefore, a causal dilated convolution-based residual DenseNet with channel attention (CARDenseNet) is investigated for bearings RUL prediction. Firstly, causal dilated convolution is introduced to learn the primary features from two-dimension time–frequency images. Then, the constructed residual DenseNet is applied to further mine the deep features. Meanwhile, the channel attention is embedded to automatically adjust the weights of different features to improve the RUL prediction accuracy. The CARDenseNet model can not only learn the long-term dependencies in time series, but also decrease the computational cost and increase the learning efficiency for complex data. Finally, the ablation analysis of the investigated model is performed using two rolling bearing datasets, and the analysis results reveals that the suggested method can get higher forecasting precision of RUL.
更多查看译文
关键词
RUL prediction,Residual DenseNet,Causal dilated convolution,Attention mechanism,Rolling bearings
AI 理解论文
溯源树
样例
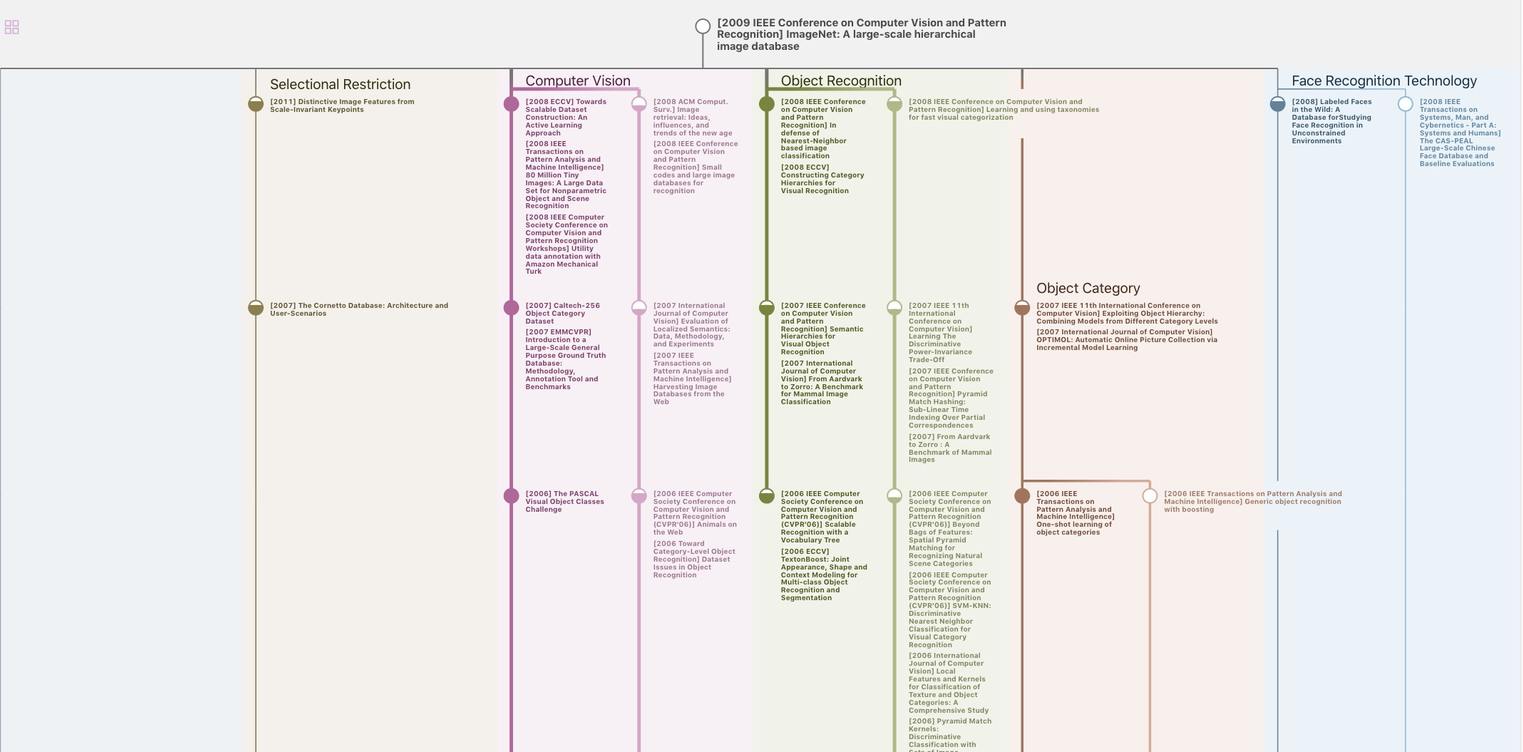
生成溯源树,研究论文发展脉络
Chat Paper
正在生成论文摘要