Defending against Adversarial Attacks in Federated Learning on Metric Learning Model.
International Conference on Trust, Security and Privacy in Computing and Communications(2023)
Abstract
The industry has widely deployed federated learning (FL) due to its promise to protect clients’ privacy. However, FL is vulnerable to adversarial attacks when the participants are compromised. The defense against adversarial attacks is a challenging problem in FL. Moreover, existing defense methods optimize the dimensionality reduction and anomaly detection models separately, leading to a disappointing projection space and low detection accuracy. We propose a deep metric learning-based anomaly detection to project the model gradients into a metric space where the malicious gradients are separated from benign ones. Meanwhile, while existing methods require an auxiliary dataset to train the defense model, the auxiliary dataset is usually unavailable to the server in the FL setting. We propose a self-supervised method to distill the data between the training epochs of our defense model. To handle radical changes in malicious model gradients, we utilize a median-based aggregated gradient filter to discard improper aggregated gradients. We show experimentally that our algorithm has a competitive performance over existing methods under Byzantine attacks and backdoor attacks with various triggers.
MoreTranslated text
Key words
Federated learning,Adversarial attack,Anomaly detection
AI Read Science
Must-Reading Tree
Example
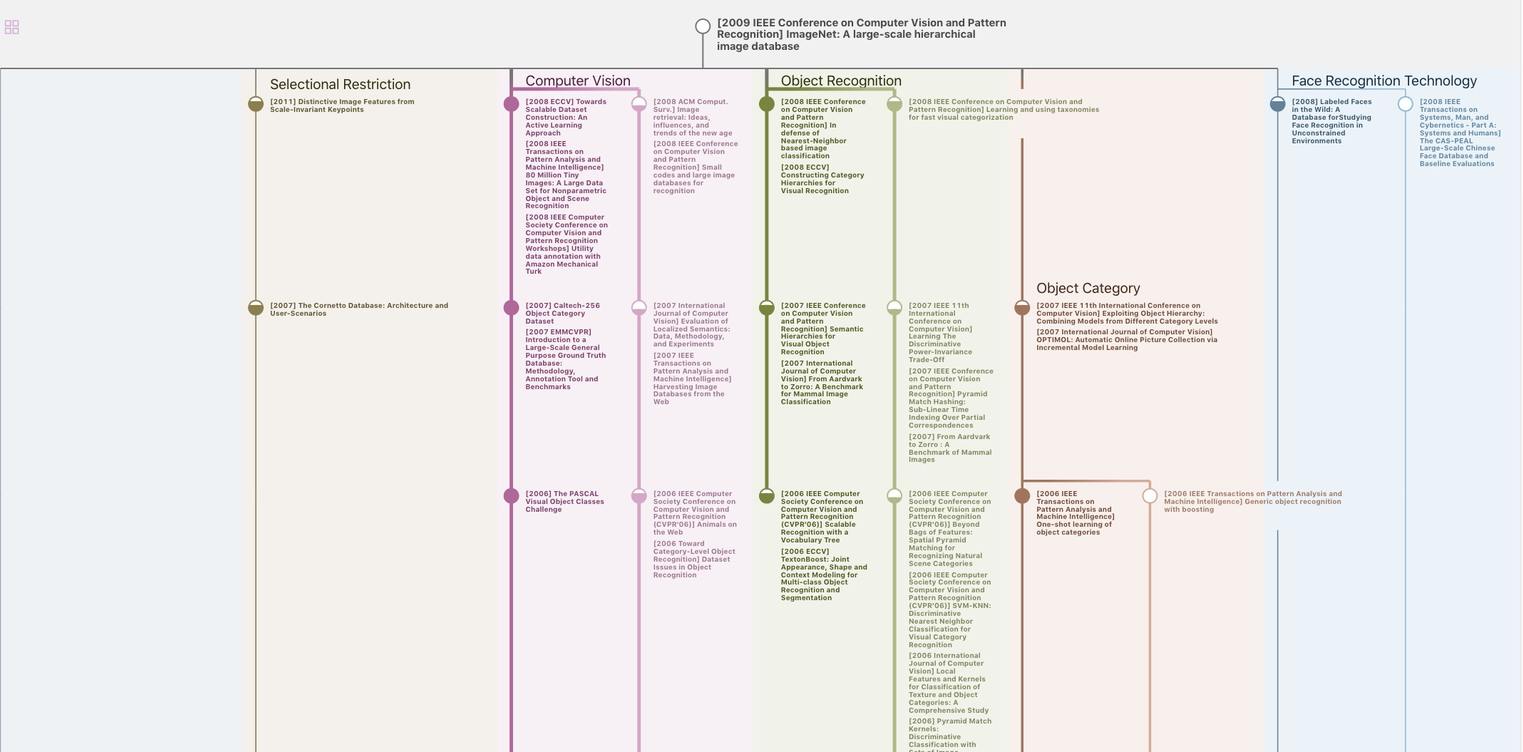
Generate MRT to find the research sequence of this paper
Chat Paper
Summary is being generated by the instructions you defined