SFNet: A Scaling-down Fusion Network for Infrared and Visible Images with Texture Attention.
Asia Conference on Algorithms, Computing and Machine Learning(2024)
摘要
The goal of infrared and visible image fusion is to generate a fused image that possesses the prominent attributes of infrared image and the rich textures of visible image. Current deep learning-based methods typically involve three main steps: feature extraction, feature fusion, and reconstruction. Extracting multi-scale features during the feature extraction stage is beneficial for fully utilizing deep features. Shallow image features exhibit higher resolution and contain more textures, while deep image features have lower resolution but stronger semantic information after more pooling and convolution. Existing methods use the same network to process features of different scales, while ignoring their differences. Moreover, they lack carefully designed texture preservation modules, leading to insufficient preservation of texture in the fused images. To overcome these issues, we propose a novel end-to-end fusion network for infrared and visible images where we develop a scaling-down network and texture attention. Based on the characteristics of features at different scales, we design a scaling-down fusion network that use deeper and more complex network to process shallow features, while using more streamlined network to process deep features. In order to better preserve image textures, we design texture attention in a relatively gentle way that focus on feature channels have rich textures to achieve the goal. We conducted experiments on publicly available datasets, and the results demonstrate that our method surpasses eleven state-of-the-art methods in terms of fusion performance. This conclusion has been verified through both subjective evaluation and objective evaluation.
更多查看译文
AI 理解论文
溯源树
样例
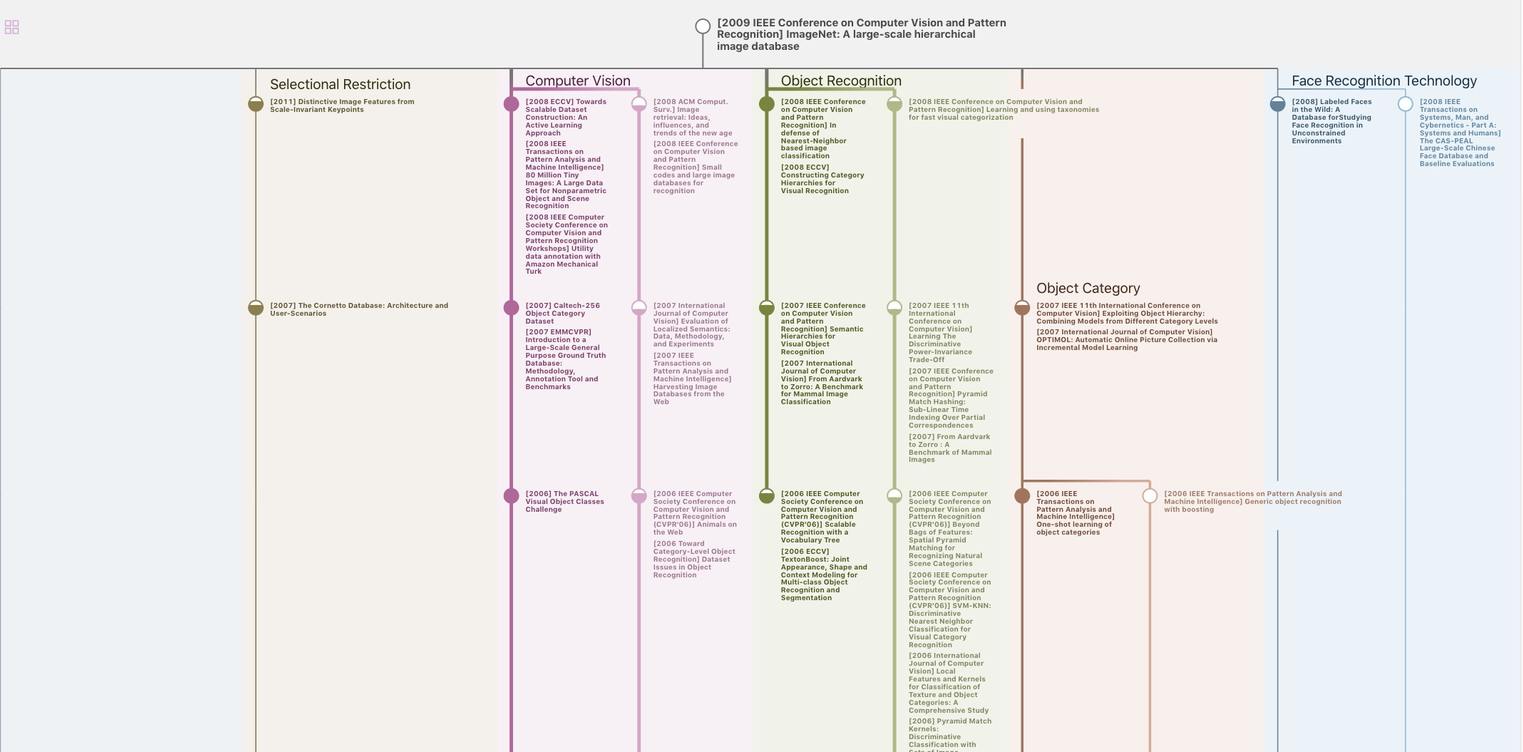
生成溯源树,研究论文发展脉络
Chat Paper
正在生成论文摘要