Causal inference in the closed-loop: marginal structural models for sequential excursion effects.
ArXiv(2024)
Abstract
Optogenetics is widely used to study the effects of neural circuit manipulation on behavior. However, the paucity of causal inference methodological work on this topic has resulted in analysis conventions that discard information, and constrain the scientific questions that can be posed. To fill this gap, we introduce a nonparametric causal inference framework for analyzing "closed-loop" designs, which use dynamic policies that assign treatment based on covariates. In this setting, standard methods can introduce bias and occlude causal effects. Building on the sequentially randomized experiments literature in causal inference, our approach extends history-restricted marginal structural models for dynamic regimes. In practice, our framework can identify a wide range of causal effects of optogenetics on trial-by-trial behavior, such as, fast/slow-acting, dose-response, additive/antagonistic, and floor/ceiling. Importantly, it does so without requiring negative controls, and can estimate how causal effect magnitudes evolve across time points. From another view, our work extends "excursion effect" methods--popular in the mobile health literature--to enable estimation of causal contrasts for treatment sequences greater than length one, in the presence of positivity violations. We derive rigorous statistical guarantees, enabling hypothesis testing of these causal effects. We demonstrate our approach on data from a recent study of dopaminergic activity on learning, and show how our method reveals relevant effects obscured in standard analyses.
MoreTranslated text
AI Read Science
Must-Reading Tree
Example
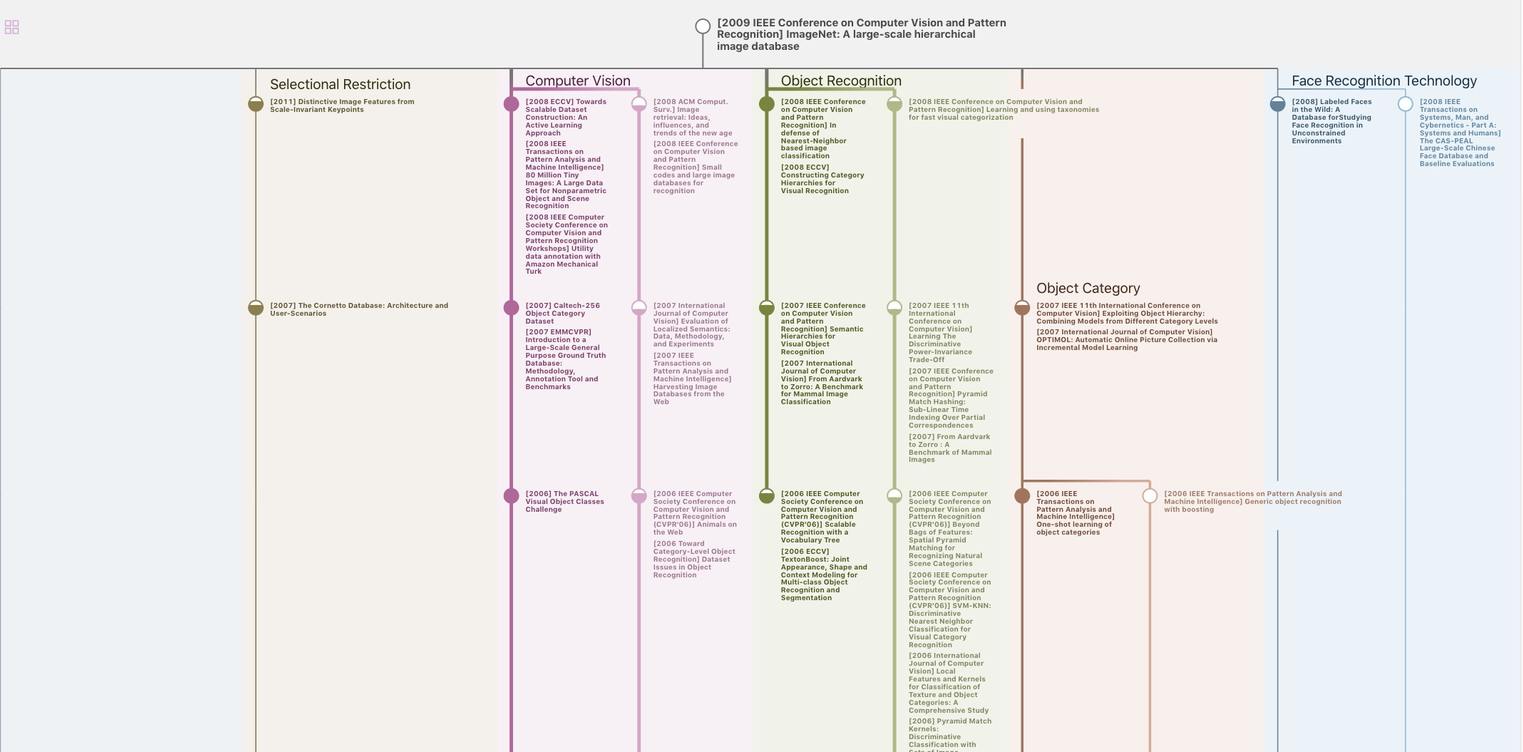
Generate MRT to find the research sequence of this paper
Chat Paper
Summary is being generated by the instructions you defined