Representations of lipid nanoparticles using large language models for transfection efficiency prediction.
Bioinformatics (Oxford, England)(2024)
摘要
MOTIVATION:Lipid nanoparticles (LNPs) are the most widely used vehicles for mRNA vaccine delivery. The structure of the lipids composing the LNPs can have a major impact on the effectiveness of the mRNA payload. Several properties should be optimized to improve delivery and expression including biodegradability, synthetic accessibility, and transfection efficiency.
RESULTS:To optimize LNPs, we developed and tested models that enable the virtual screening of LNPs with high transfection efficiency. Our best method uses the lipid Simplified Molecular-Input Line-Entry System (SMILES) as inputs to a large language model. Large language model-generated embeddings are then used by a downstream gradient-boosting classifier. As we show, our method can more accurately predict lipid properties, which could lead to higher efficiency and reduced experimental time and costs.
AVAILABILITY AND IMPLEMENTATION:Code and data links available at: https://github.com/Sanofi-Public/LipoBART.
更多查看译文
AI 理解论文
溯源树
样例
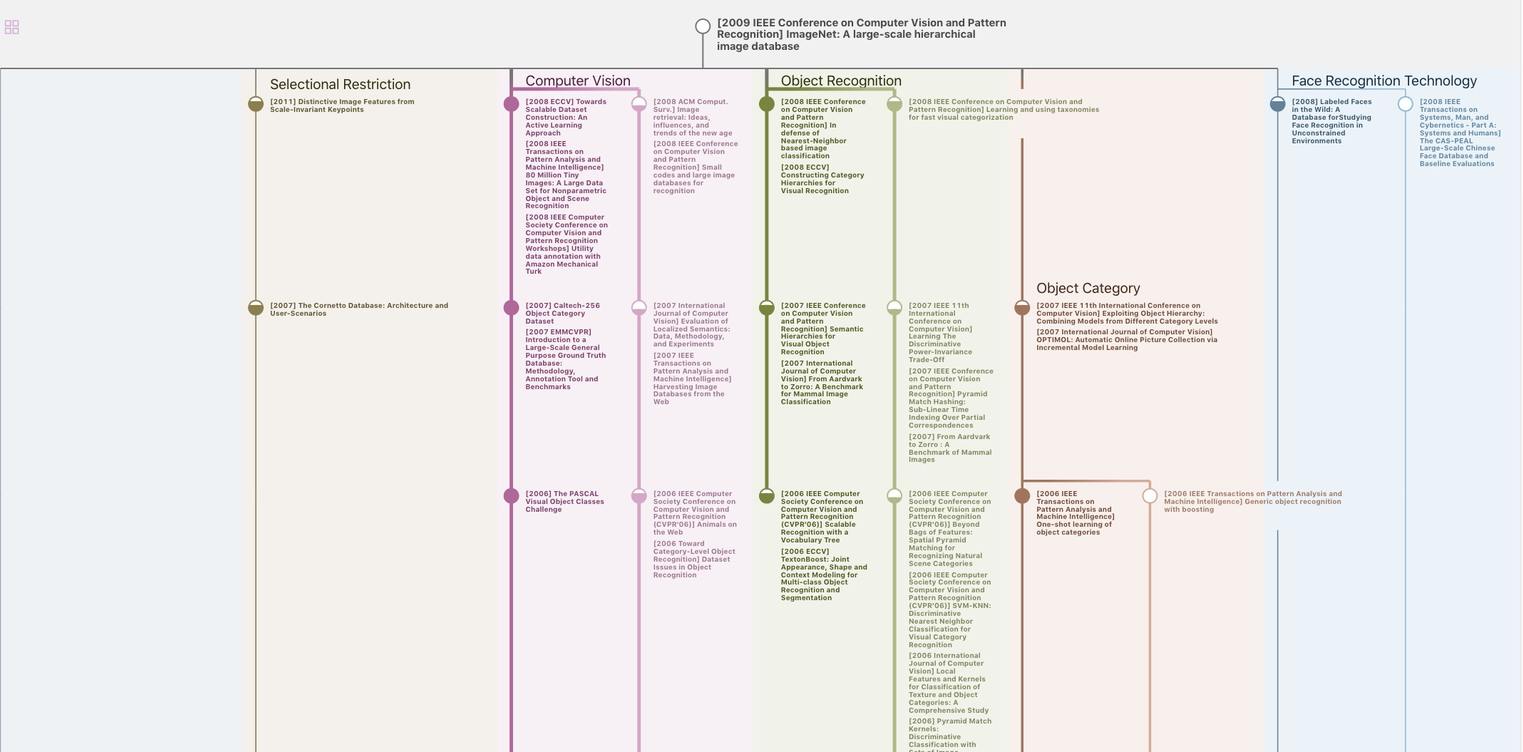
生成溯源树,研究论文发展脉络
Chat Paper
正在生成论文摘要