DSSGNN-PPI: A Protein-Protein Interactions prediction model based on Double Structure and Sequence graph neural networks
Computers in Biology and Medicine(2024)
摘要
The process of experimentally confirming complex interaction networks among proteins is time-consuming and laborious. This study aims to address Protein-Protein Interactions (PPIs) prediction based on graph neural networks (GNN). A novel multilevel prediction model for PPIs named DSSGNN-PPI (Double Structure and Sequence GNN for PPIs) is designed. Initially, a distance graph between amino acid residues is constructed. Subsequently, the distance graph is fed into an underlying graph attention network module. This enables us to efficiently learn vector representations that encode the three-dimensional structure of proteins and simultaneously aggregate key local patterns and overall topological information to obtain graph embedding that adequately represent local and global structural features. In addition, the embedding representations that reflect sequence properties are obtained. Two features are fused to construct high-level protein complex networks, which are fed into the designed gated graph attention network to extract complex topological patterns. By combining heterogeneous multi-source information from downstream structure graph and upstream sequence models, the understanding of PPIs is comprehensively enhanced. A series of evaluation results validate the remarkable effectiveness of DSSGNN-PPI framework in enhancing the prediction of multi-type interactions among proteins. The multilevel representation learning and information fusion strategies provide a new effective solution paradigm for structural biology problems. The source code for DSSGNN-PPI has been hosted on GitHub and is available at https://github.com/cstudy1/DSSGNN-PPI.
更多查看译文
关键词
Protein-Protein Interactions,Prediction,Graph neural networks,Dual-level architecture,DSSGNN-PPI
AI 理解论文
溯源树
样例
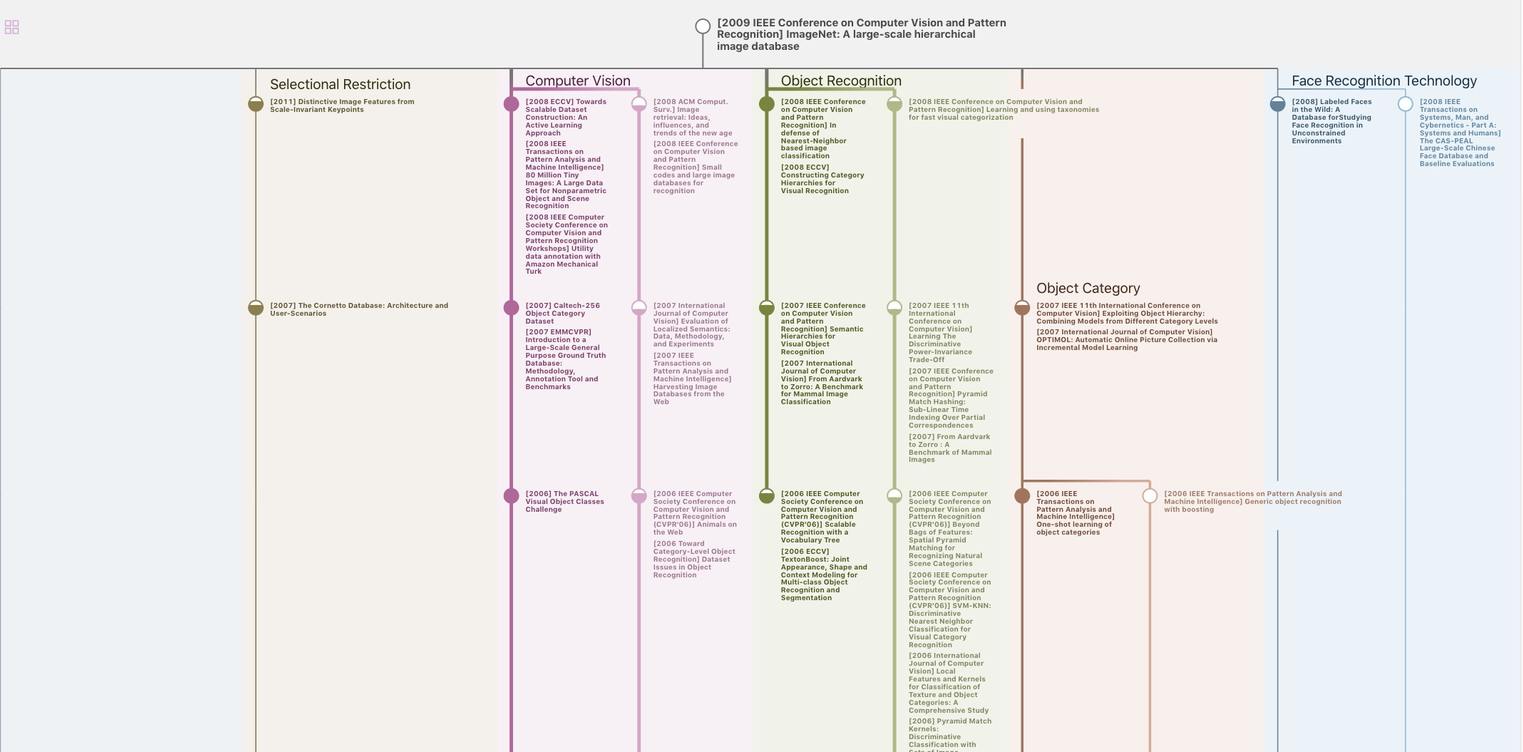
生成溯源树,研究论文发展脉络
Chat Paper
正在生成论文摘要