Distributed Deep Learning for Big Remote Sensing Data Processing on Apache Spark: Geological Remote Sensing Interpretation As a Case Study
WEB AND BIG DATA, PT I, APWEB-WAIM 2023(2024)
关键词
Remote sensing,Distributed deep learning,Apache spark,Distributed geological remote sensing interpretation
AI 理解论文
溯源树
样例
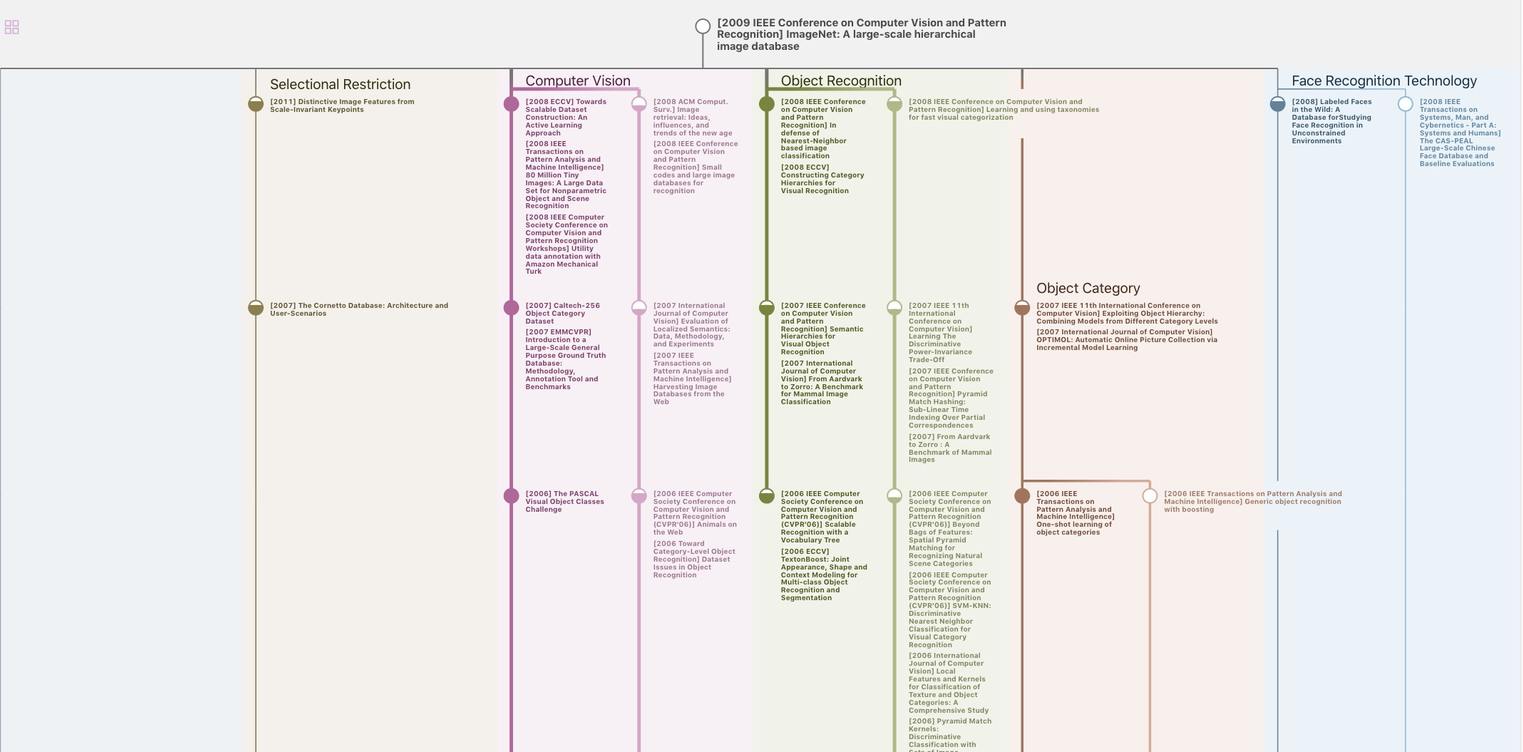
生成溯源树,研究论文发展脉络
Chat Paper
正在生成论文摘要