A Registration Method of Overlap Aware Point Clouds Based on Transformer-to-Transformer Regression
Remote Sensing(2024)
Abstract
Transformer has recently become widely adopted in point cloud registration. Nevertheless, Transformer is unsuitable for handling dense point clouds due to resource constraints and the sheer volume of data. We propose a method for directly regressing the rigid relative transformation of dense point cloud pairs. Specifically, we divide the dense point clouds into blocks according to the down-sampled superpoints. During training, we randomly select point cloud blocks with varying overlap ratios, and during testing, we introduce the overlap-aware Rotation-Invariant Geometric Transformer Cross-Encoder (RIG-Transformer), which predicts superpoints situated within the common area of the point cloud pairs. The dense points corresponding to the superpoints are inputted into the Transformer Cross-Encoder to estimate their correspondences. Through the fusion of our RIG-Transformer and Transformer Cross-Encoder, we propose Transformer-to-Transformer Regression (TTReg), which leverages dense point clouds from overlapping regions for both training and testing phases, calculating the relative transformation of the dense points by using the predicted correspondences without random sample consensus (RANSAC). We have evaluated our method on challenging benchmark datasets, including 3DMatch, 3DLoMatch, ModelNet, and ModelLoNet, demonstrating up to a 7.2% improvement in registration recall. The improvements are attributed to our RIG-Transformer module and regression mechanism, which makes the features of superpoints more discriminative.
MoreTranslated text
Key words
point cloud registration,Transformer-to-Transformer,dense point cloud
AI Read Science
Must-Reading Tree
Example
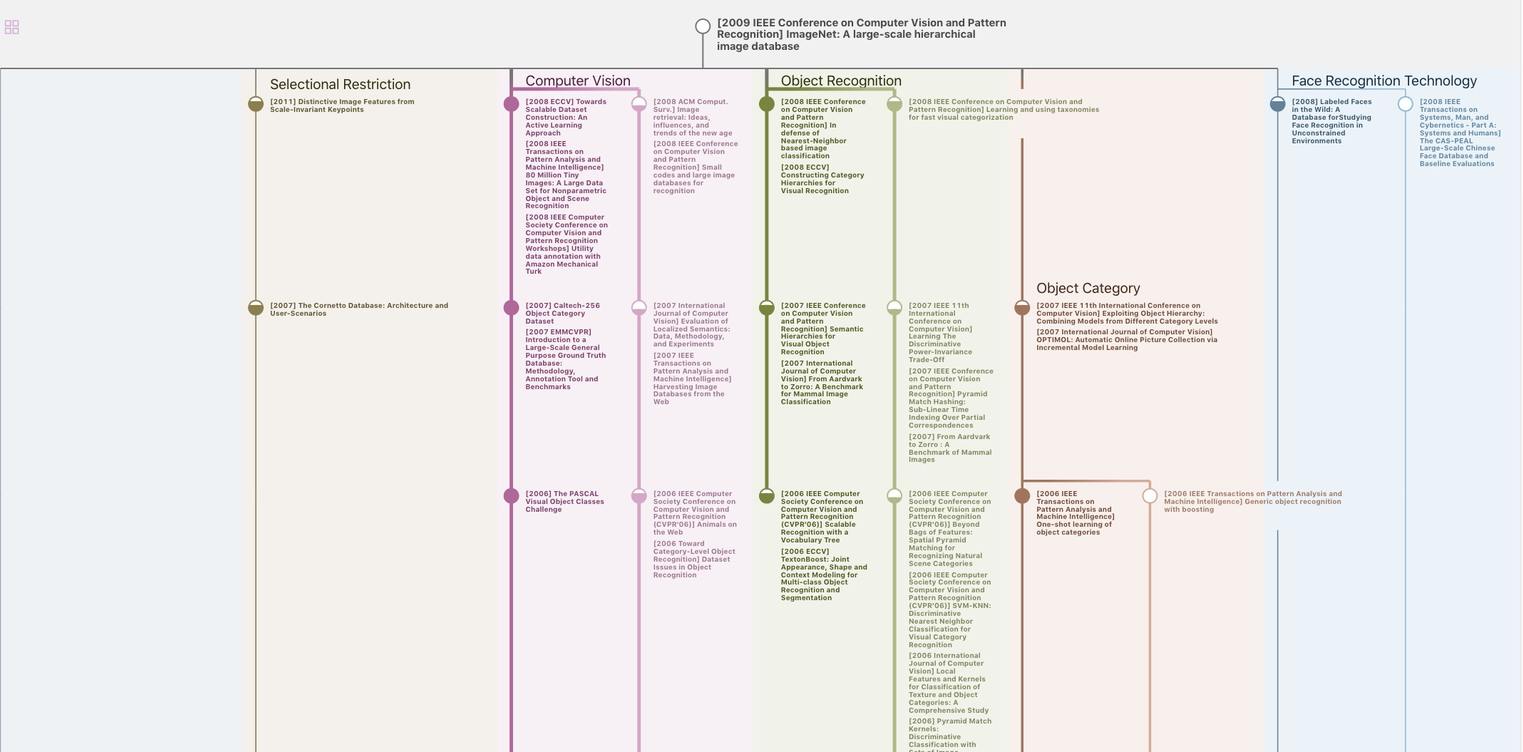
Generate MRT to find the research sequence of this paper
Chat Paper
Summary is being generated by the instructions you defined