MECFNet: Reconstruct Sharp Image for UAV-Based Crack Detection
IEEE TRANSACTIONS ON INTELLIGENT TRANSPORTATION SYSTEMS(2024)
Abstract
Crack detection, as one of the structural health monitoring tasks, plays a crucial role in ensuring the reliability of transportation infrastructure. And the convenience of Unmanned Aerial Vehicles (UAVs) has led to their widespread utilization as carriers for crack detection task. However, the motion and vibration of the carrier inevitably lead to image blurring, consequently degrading the performance of subsequent crack detection. While existing image deblurring methods exhibit commendable performance in feature-rich real-world scenarios, their efficacy tends to diminish in feature-limited environments, such as the surfaces of concrete structures and pavements. In response to this challenge, we propose a novel deblurring network, named Motion and Event-guided Cross-modal Fusion Network (MECFNet), by incorporating motion information and event information as constraints to guide the image reconstruction process. For one thing, we devise two novel representation methods: motion field and accumulated event to characterize these two types of information. For another, we introduce a cross-modal transformer module to incorporate these two new modalities of information into the image features. Comparison experiments and ablation experiments have demonstrated the effectiveness of the proposed network and its constituent components. Furthermore, the performance of crack detection achieves a significant enhancement after the reconstruction of blurry images.
MoreTranslated text
Key words
Feature extraction,Crack detection,image deblurring,motion field,accumulated event,cross-modal transformer
AI Read Science
Must-Reading Tree
Example
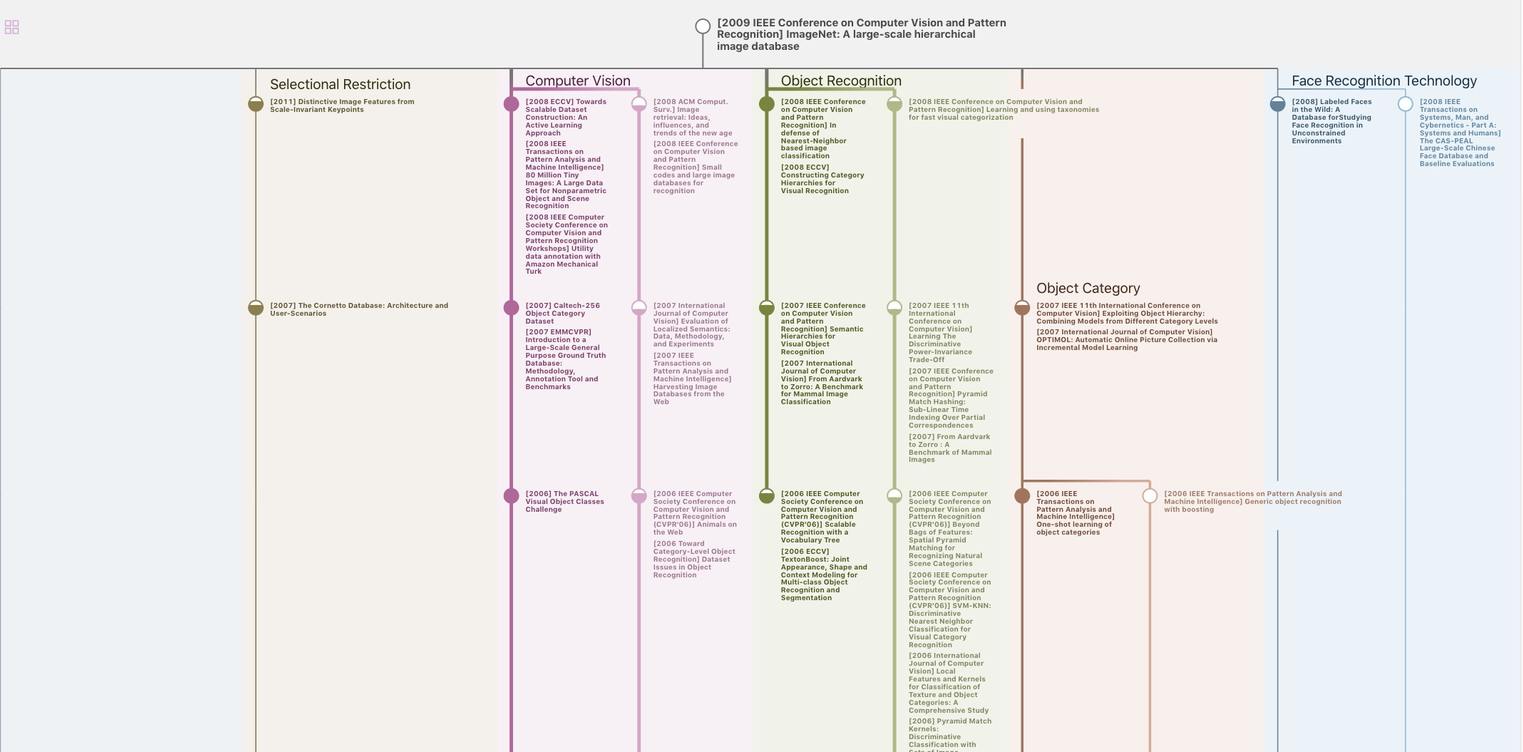
Generate MRT to find the research sequence of this paper
Chat Paper
Summary is being generated by the instructions you defined