Privacy-Aware Visual Language Models
CoRR(2024)
摘要
This paper aims to advance our understanding of how Visual Language Models
(VLMs) handle privacy-sensitive information, a crucial concern as these
technologies become integral to everyday life. To this end, we introduce a new
benchmark PrivBench, which contains images from 8 sensitive categories such as
passports, or fingerprints. We evaluate 10 state-of-the-art VLMs on this
benchmark and observe a generally limited understanding of privacy,
highlighting a significant area for model improvement. Based on this we
introduce PrivTune, a new instruction-tuning dataset aimed at equipping VLMs
with knowledge about visual privacy. By tuning two pretrained VLMs, TinyLLaVa
and MiniGPT-v2, on this small dataset, we achieve strong gains in their ability
to recognize sensitive content, outperforming even GPT4-V. At the same time, we
show that privacy-tuning only minimally affects the VLMs performance on
standard benchmarks such as VQA. Overall, this paper lays out a crucial
challenge for making VLMs effective in handling real-world data safely and
provides a simple recipe that takes the first step towards building
privacy-aware VLMs.
更多查看译文
AI 理解论文
溯源树
样例
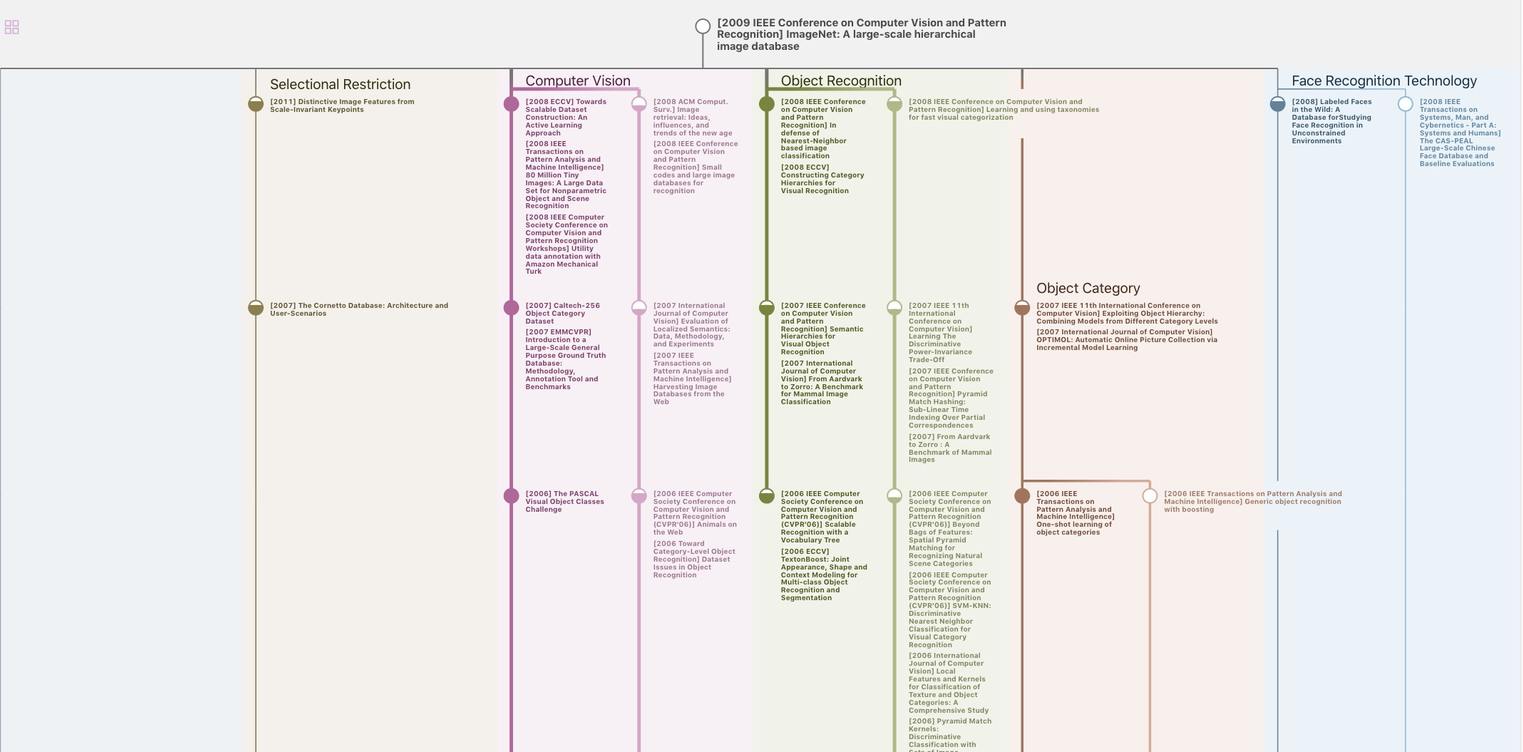
生成溯源树,研究论文发展脉络
Chat Paper
正在生成论文摘要