A Hybrid Deep Learning-Based Framework for Chip Packaging Fault Diagnostics in X-Ray Images
IEEE Transactions on Industrial Informatics(2024)
摘要
In the testing of chips, defect diagnostics in X-ray images of packaging chips is mainly performed by humans, which is time-consuming and inefficient. To overcome the abovementioned problems, a novel intelligent defect diagnostics system based on hybrid deep learning for chip X-ray images was proposed. The system consists of four successive stages: image segmentation and normalization, image reconstruction and defect detection, contour matching, and qualification diagnosis. The first stage is used to localize the external contours of the target chip and remove extraneous backgrounds through the improved UNet. Then, considering the variety of defects and the complexity of labeling, an unsupervised learning model is designed to reconstruct defect-free images to detect defects, which requires only normal samples for training. Third, the multicomponent template matching based on structural prior is used to localize the internal contours of the chip. In the final stage, the qualification is diagnosed based on the previous results through the Floyd–Warshall algorithm. The effectiveness and robustness of the proposed methods are verified by experiments on real-world inspection lines. The experimental results demonstrate that the developed system can successfully perform fault diagnostics tasks, achieving a judgment accuracy of 92.5%.
更多查看译文
关键词
Deep learning,defect diagnostics,unsupervised learning,X-ray images
AI 理解论文
溯源树
样例
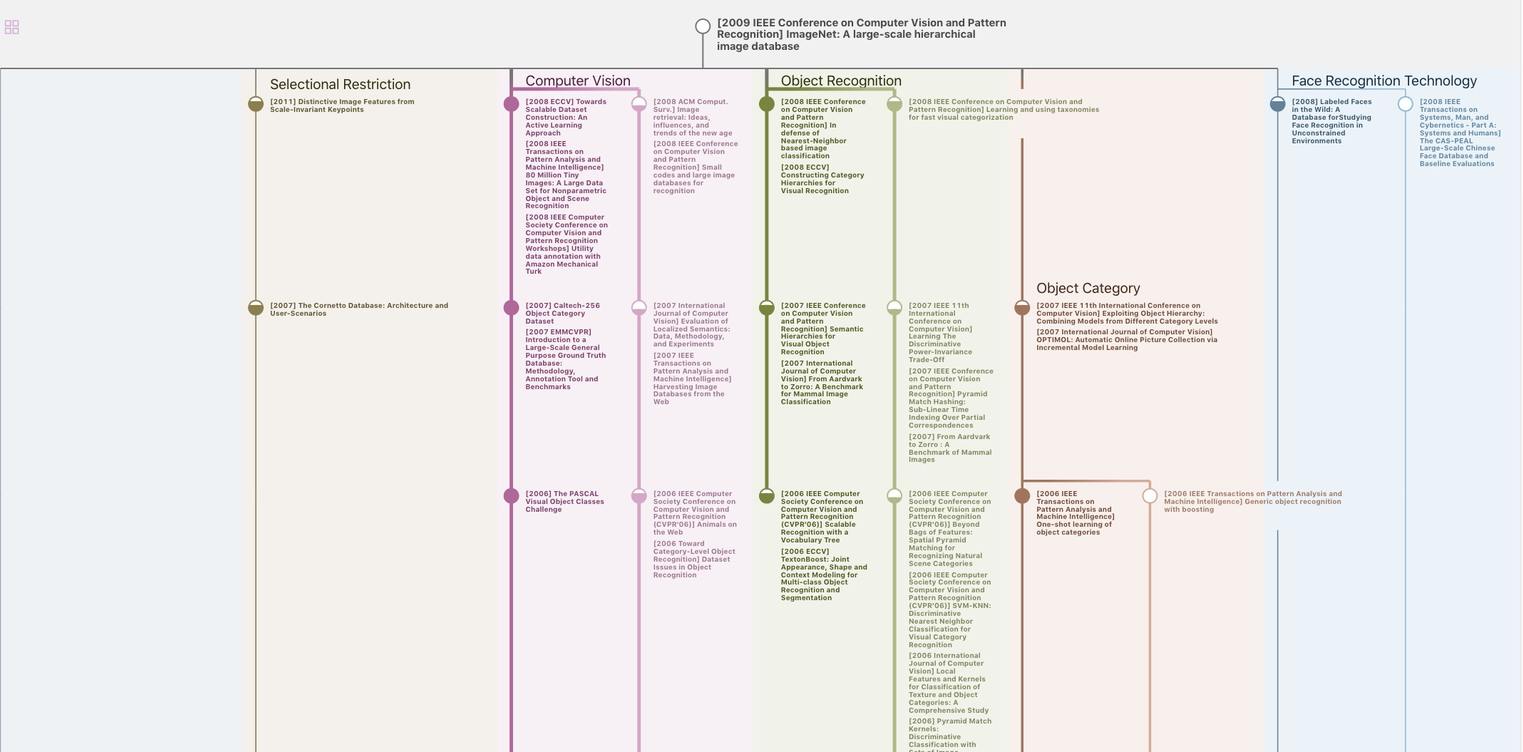
生成溯源树,研究论文发展脉络
Chat Paper
正在生成论文摘要