Machine learning assisted improved desalination pilot system design and experimentation for the circular economy
Journal of Water Process Engineering(2024)
摘要
Desalination is among the most feasible solutions to supply sustainable and clean drinking water in water scarcity areas. In this regard, Multi-Effect Desalination (MED) systems are particularly preferred for harsh feeds (high temperature and salinity) because of their robust mode of operation for water production. However, maintaining the efficient operation of the MED systems is challenging because of the large system design and variables' interdependencies that are sensitive to the distillate production. Therefore, this research leverages the power of machine learning and optimization to estimate the optimal operating conditions for the maximum distillate production from the MED system. In the first step, detailed experimentation is conducted for distillate production against hot water temperature (HWT) varying from 38 to 70 °C, and feed water temperature (FWT) is changed from 34 to 42 °C. Whereas, the feed flow rate (FFR) is investigated to be varied nearly from 3.6 to 8.7 LPM in the three stages, i.e., FFR-S1, FFR-S2 and FFR-S3. The compiled dataset is used to make the process models of the MED system by three ML-based algorithms, i.e., Artificial Neural Network (ANN), Support Vector Machine (SVM), and Gaussian Process Regression (GPR) under rigorous hyperparameters optimization. GPR exhibited superior predictive performance than those of ANN and SVM on R2 value of 0.99 and RMSE of 0.026 LPM. Monte Carlo technique-based variable significance analysis revealed that the HWT has the highest effect on distillate production with a percentage significance of 95.6 %. Then Genetic Algorithm is used to maximize the distillate production with the GPR model embedded in the optimization problem. The GPR-GA driven maximum distillate production is estimated on HWT = 70 ± 0.5 °C, FWT = 40 ± 2.5 °C, FFR-S1 = 6 ± 2.6 LPM, FFR-S2 = 7 ± 1 LPM and FFR-S3 = 7 ± 1. The ML-GA-based system analysis and optimization of the MED system can boost the distillate production that promotes operation excellence and circular economy from the desalination sector.
更多查看译文
关键词
Water circular economy,Distillate production,Machine learning,Sustainable development goal,Desalination
AI 理解论文
溯源树
样例
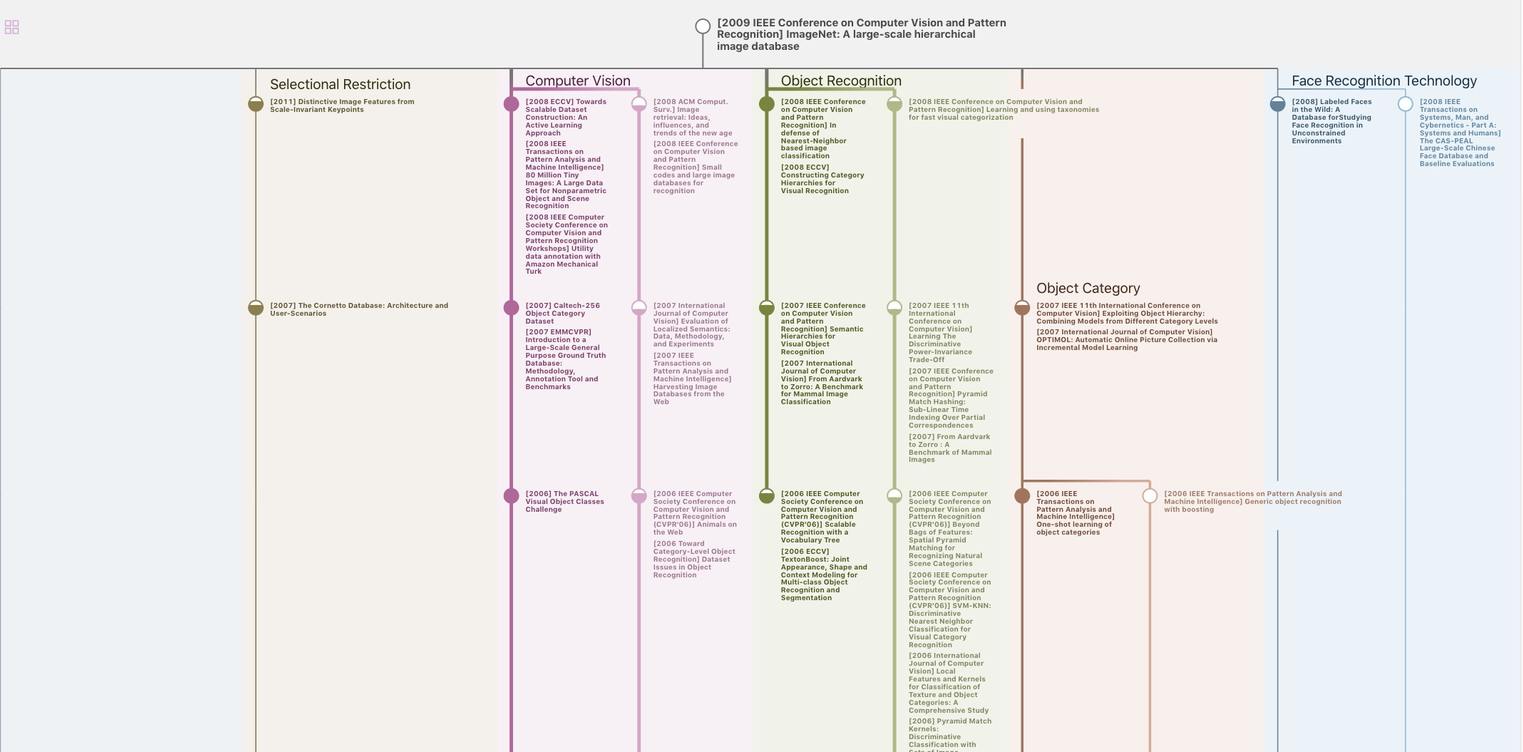
生成溯源树,研究论文发展脉络
Chat Paper
正在生成论文摘要