Remaining useful life estimation based on selective ensemble of deep neural networks with diversity
Advanced Engineering Informatics(2024)
摘要
Combining ensemble learning with deep neural networks is an effective method for accurately predicting the remaining useful life (RUL). However, most related works fail to balance accuracy and independence of models, leading to poor enhancement results and lacking robustness. To address this issue, a two-stage selective deep neural network ensemble method was developed to enhance prediction accuracy and convergence efficiency. The first stage aimed at generating deep learning models with high diversity. This was achieved by training the base models through multi-perturbation, including heterogeneous network structures, multi-time scales, and randomization of algorithm parameters. In the second stage, a selection criterion was designed that incorporated model structural diversity, behavior diversity, and base model accuracy, balancing model variety and equitability. The criterion was used as fitness function, and genetic algorithm was used to effectively prune redundant models and obtain the optimal subset, with the output based on average ensemble. Experimental results show that the proposed method significantly improves prediction accuracy and generalization capabilities.
更多查看译文
关键词
Remaining useful life,Ensemble diversity,Selective ensemble learning,Heterogeneous deep neural network
AI 理解论文
溯源树
样例
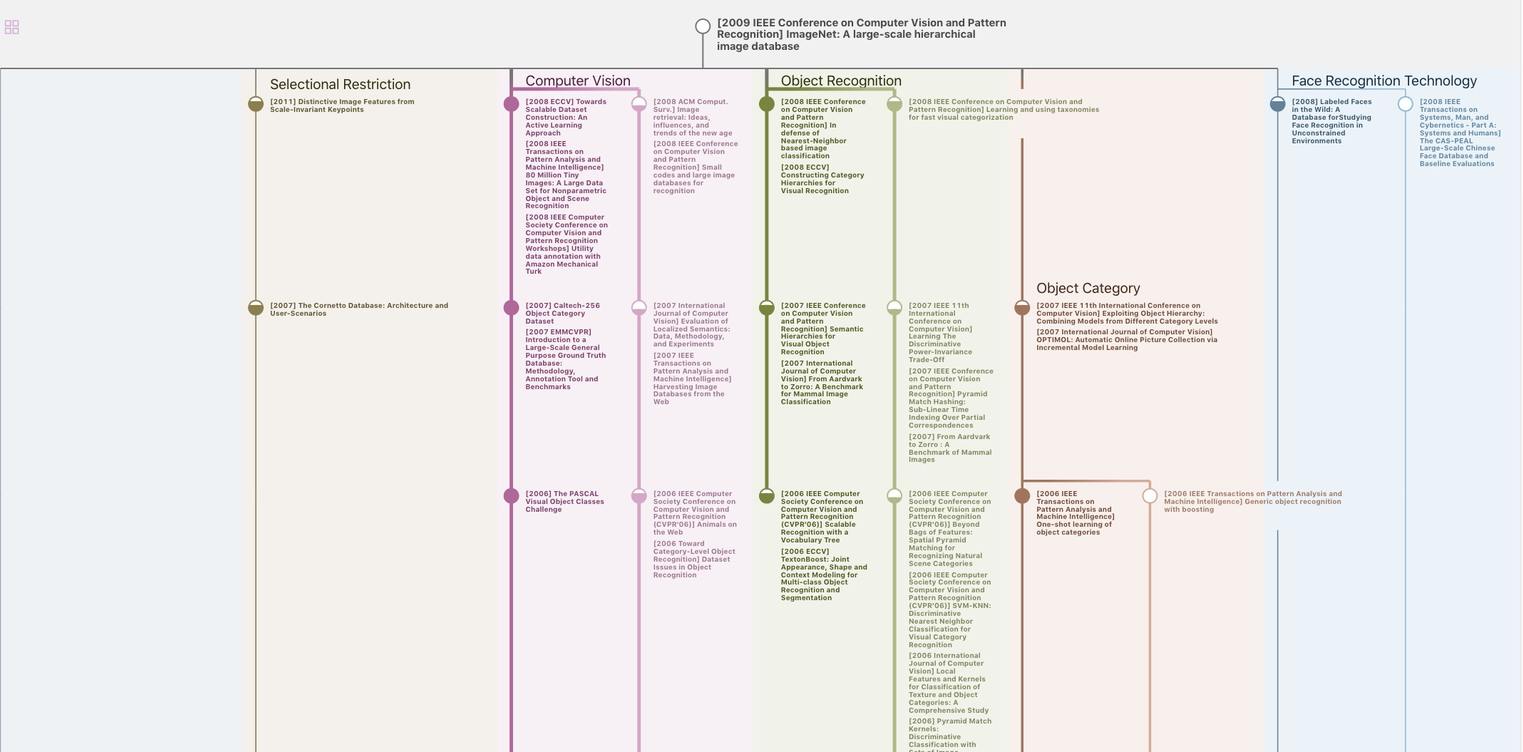
生成溯源树,研究论文发展脉络
Chat Paper
正在生成论文摘要