Data Summarization for Federated Learning.
International Conference on Machine Learning for Networking(2023)
摘要
We explore data summarization techniques as a mean to reduce the energy footprint of Federated Learning (FL). We formulate the problem of selecting a small subset of data points that best represent the gradient of each local dataset as a submodular maximization problem and provide sufficient conditions under which the FL training is guaranteed to converge to the same global model as if the whole local datasets have been used on each client. Experimental results on IID and non-IID datasets show that this approach yields a similar accuracy as training on the full local datasets, but with a significant reduction of runtimes. There is however no clear advantage of data summarization over random sampling.
更多查看译文
AI 理解论文
溯源树
样例
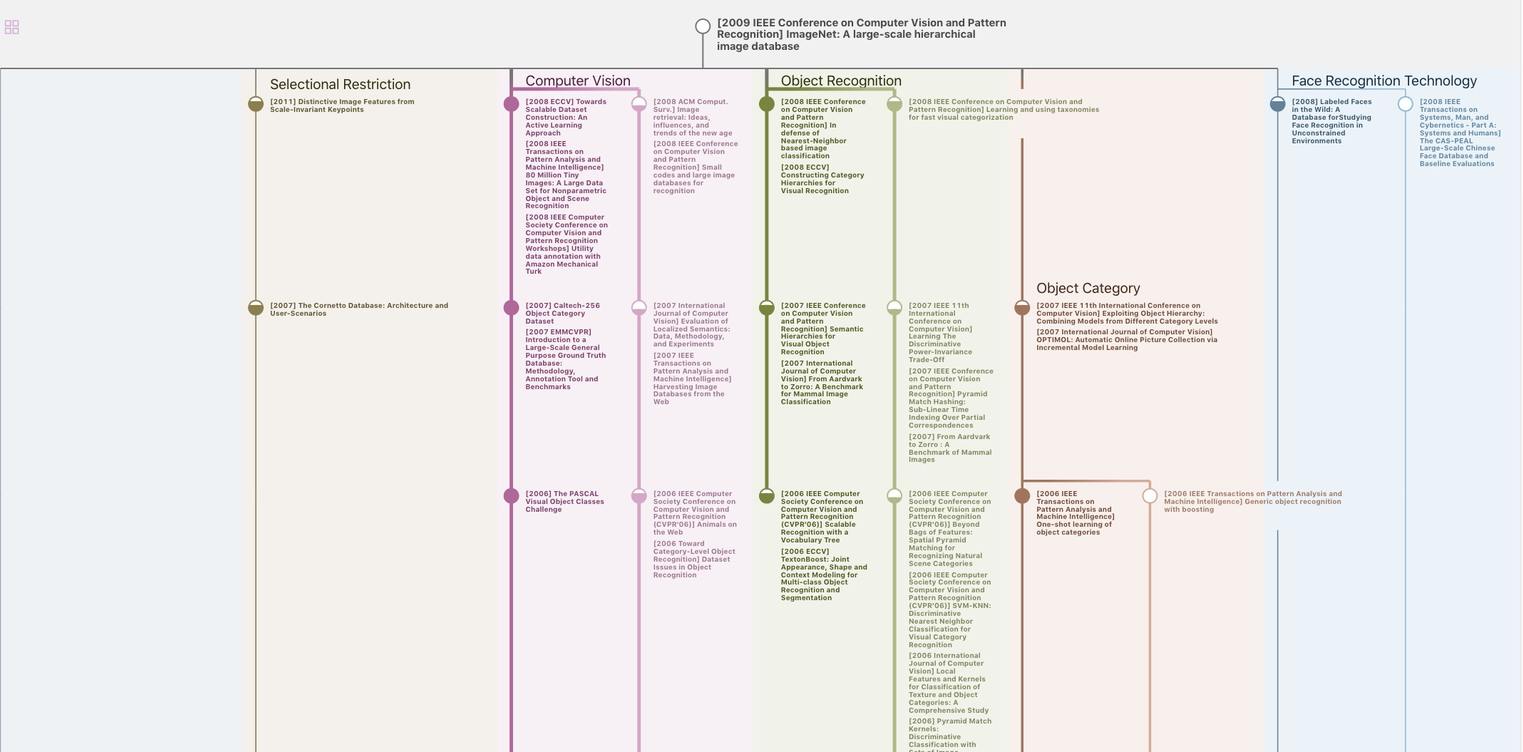
生成溯源树,研究论文发展脉络
Chat Paper
正在生成论文摘要