Railway Track Multi-component Segmentation Based on Residual Contextual Transformer
IEEE Sensors Journal(2024)
摘要
Regular inspection of track component conditions is crucial for maintaining railway safety, and accurate segmentation of track multi-component is fundamental to the detection and severity evaluation of track diseases. The U-shaped architecture has achieved tremendous success in segmentation task. However, most of existing U-shaped architecture-based methods are not suitable for track multi-component segmentation since the limitations in explicitly modeling long-range dependency. In order to address this problem, this paper proposes an innovative track multi-component segmentation network based on the Residual Contextual Transformer and adopts the encoder-decoder framework. In encoder stage, the long-range rich context information can be obtained by adopting designed Residual Contextual Transformer blocks and cascading transformer layers. In decoder stage, a multi-scale feature fusion module is used to aggregate the decoding features of different scales to predict segmentation results. Specially, the decoding features of each scale are obtained by integrating the coding features of the corresponding scale. Numerous tests have been carried out on constructed track datasets. The experimental results demonstrate that our proposed network exhibits strong segmentation capabilities for track multi-component and surpasses the benchmarks in metrics of mIoU and mDice.
更多查看译文
关键词
Track multi-component segmentation,Residual Contextual Transformer,Transformer,multi-scale feature fusion
AI 理解论文
溯源树
样例
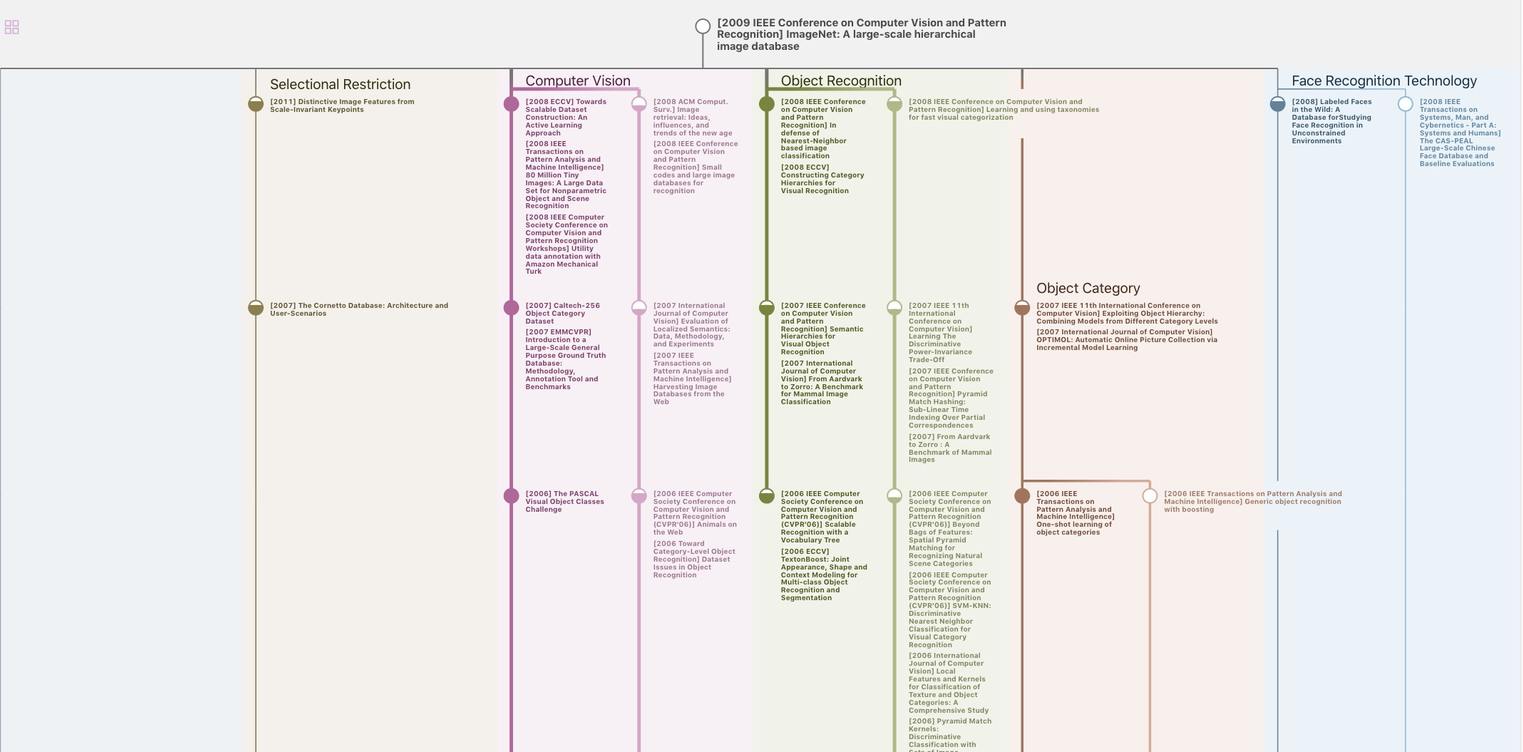
生成溯源树,研究论文发展脉络
Chat Paper
正在生成论文摘要