LabObf: A Label Protection Scheme for Vertical Federated Learning Through Label Obfuscation
CoRR(2024)
Abstract
Split learning, as one of the most common architectures in vertical federated
learning, has gained widespread use in industry due to its privacy-preserving
characteristics. In this architecture, the party holding the labels seeks
cooperation from other parties to improve model performance due to insufficient
feature data. Each of these participants has a self-defined bottom model to
learn hidden representations from its own feature data and uploads the
embedding vectors to the top model held by the label holder for final
predictions. This design allows participants to conduct joint training without
directly exchanging data. However, existing research points out that malicious
participants may still infer label information from the uploaded embeddings,
leading to privacy leakage. In this paper, we first propose an embedding
extension attack that manually modifies embeddings to undermine existing
defense strategies, which rely on constraining the correlation between the
embeddings uploaded by participants and the labels. Subsequently, we propose a
new label obfuscation defense strategy, called `LabObf', which randomly maps
each original one-hot vector label to multiple numerical soft labels with
values intertwined, significantly increasing the difficulty for attackers to
infer the labels. We conduct experiments on four different types of datasets,
and the results show that LabObf can reduce the attacker's success rate to near
random guessing while maintaining an acceptable model accuracy.
MoreTranslated text
AI Read Science
Must-Reading Tree
Example
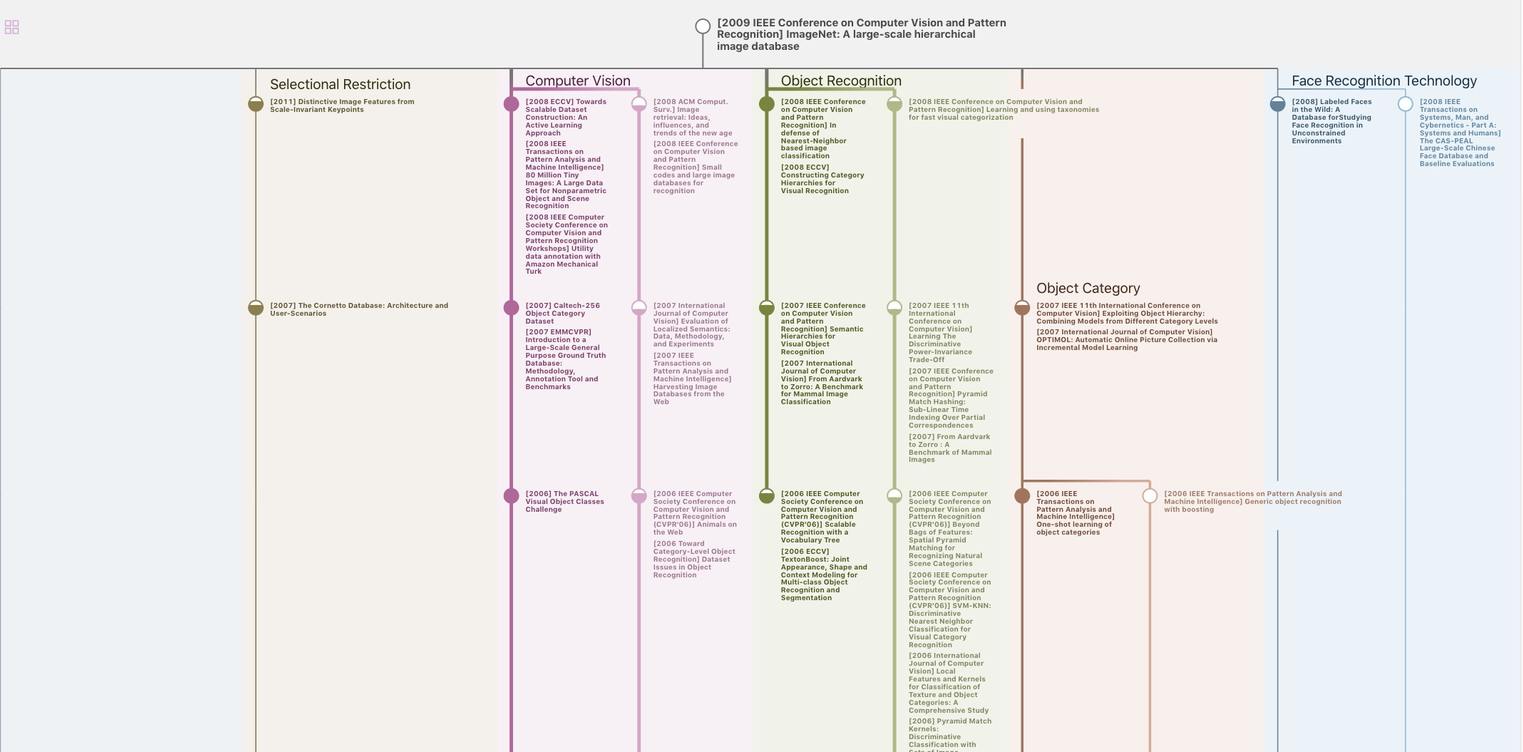
Generate MRT to find the research sequence of this paper
Chat Paper
Summary is being generated by the instructions you defined