Transport of Algebraic Structure to Latent Embeddings
ICML 2024(2024)
Abstract
Machine learning often aims to produce latent embeddings of inputs which lie in a larger, abstract mathematical space. For example, in the field of 3D modeling, subsets of Euclidean space can be embedded as vectors using implicit neural representations. Such subsets also have a natural algebraic structure including operations (e.g., union) and corresponding laws (e.g., associativity). How can we learn to "union" two sets using only their latent embeddings while respecting associativity? We propose a general procedure for parameterizing latent space operations that are provably consistent with the laws on the input space. This is achieved by learning a bijection from the latent space to a carefully designedmirrored algebrawhich is constructed on Euclidean space in accordance with desired laws. We evaluate thesestructural transport netsfor a range of mirrored algebras against baselines that operate directly on the latent space. Our experiments provide strong evidence that respecting the underlying algebraic structure of the input space is key for learning accurate and self-consistent operations.
MoreTranslated text
AI Read Science
Must-Reading Tree
Example
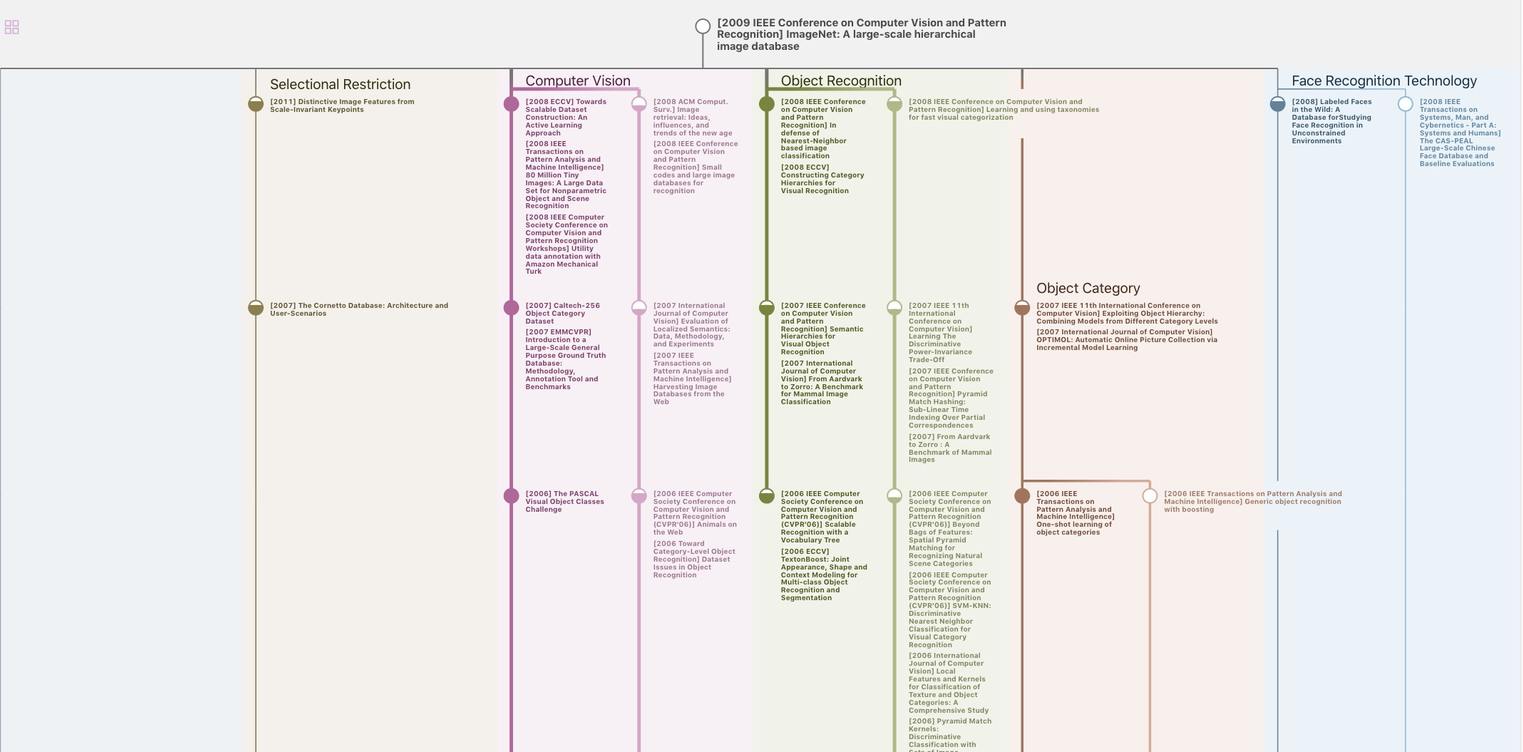
Generate MRT to find the research sequence of this paper
Chat Paper
Summary is being generated by the instructions you defined