Make Safe Decisions in Power System: Safe Reinforcement Learning Based Pre-decision Making for Voltage Stability Emergency Control
CoRR(2024)
摘要
The high penetration of renewable energy and power electronic equipment bring
significant challenges to the efficient construction of adaptive emergency
control strategies against various presumed contingencies in today's power
systems. Traditional model-based emergency control methods have difficulty in
adapt well to various complicated operating conditions in practice. Fr emerging
artificial intelligence-based approaches, i.e., reinforcement learning-enabled
solutions, they are yet to provide solid safety assurances under strict
constraints in practical power systems. To address these research gaps, this
paper develops a safe reinforcement learning (SRL)-based pre-decision making
framework against short-term voltage collapse. Our proposed framework employs
neural networks for pre-decision formulation, security margin estimation, and
corrective action implementation, without reliance on precise system
parameters. Leveraging the gradient projection, we propose a security
projecting correction algorithm that offers theoretical security assurances to
amend risky actions. The applicability of the algorithm is further enhanced
through the incorporation of active learning, which expedites the training
process and improves security estimation accuracy. Extensive numerical tests on
the New England 39-bus system and the realistic Guangdong Provincal Power Grid
demonstrate the effectiveness of the proposed framework.
更多查看译文
AI 理解论文
溯源树
样例
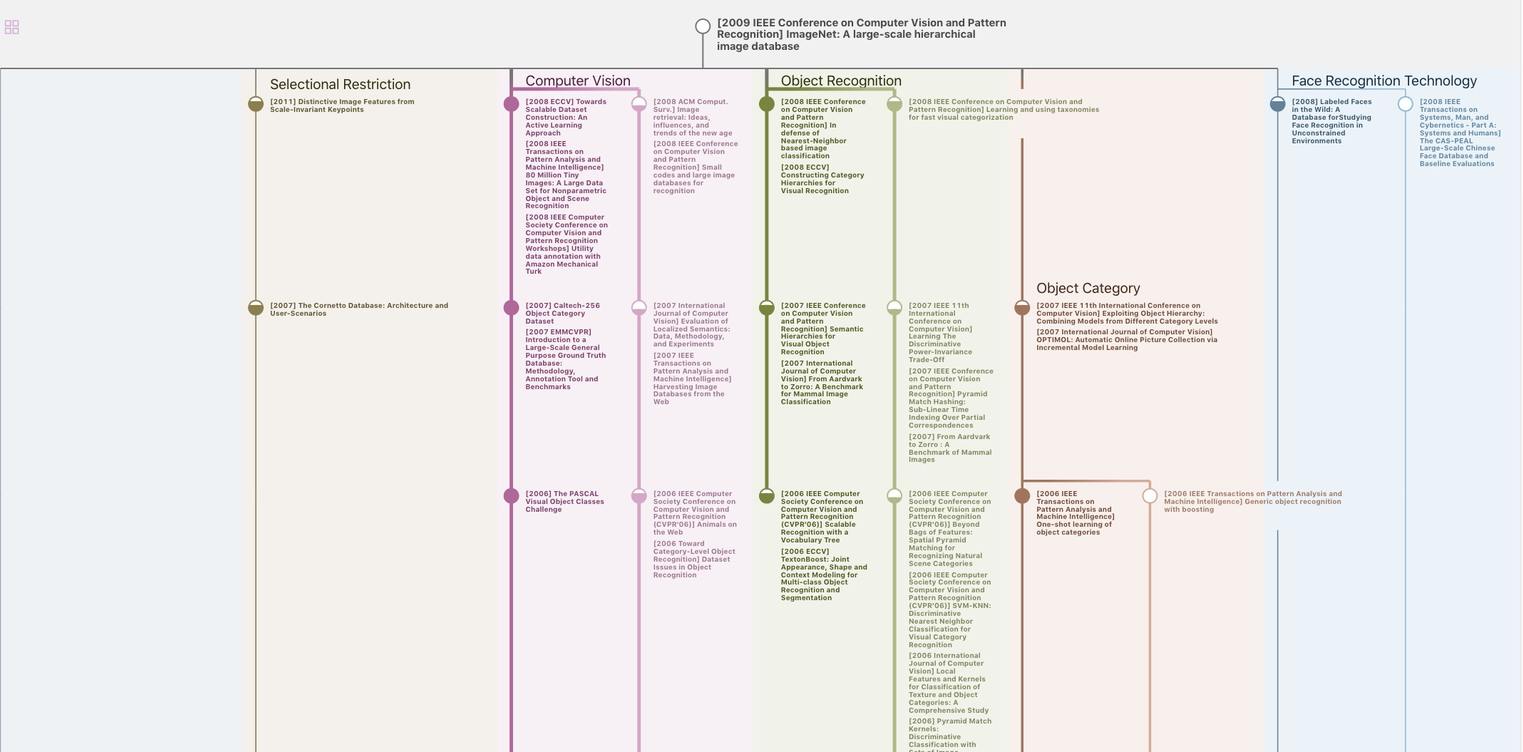
生成溯源树,研究论文发展脉络
Chat Paper
正在生成论文摘要