EEG-DBNet: A Dual-Branch Network for Temporal-Spectral Decoding in Motor-Imagery Brain-Computer Interfaces
CoRR(2024)
Abstract
Motor imagery electroencephalogram (EEG)-based brain-computer interfaces
(BCIs) offer significant advantages for individuals with restricted limb
mobility. However, challenges such as low signal-to-noise ratio and limited
spatial resolution impede accurate feature extraction from EEG signals, thereby
affecting the classification accuracy of different actions. To address these
challenges, this study proposes an end-to-end dual-branch network (EEG-DBNet)
that decodes the temporal and spectral sequences of EEG signals in parallel
through two distinct network branches. Each branch comprises a local
convolutional block and a global convolutional block. The local convolutional
block transforms the source signal from the temporal-spatial domain to the
temporal-spectral domain. By varying the number of filters and convolution
kernel sizes, the local convolutional blocks in different branches adjust the
length of their respective dimension sequences. Different types of pooling
layers are then employed to emphasize the features of various dimension
sequences, setting the stage for subsequent global feature extraction. The
global convolution block splits and reconstructs the feature of the signal
sequence processed by the local convolution block in the same branch and
further extracts features through the dilated causal convolutional neural
networks. Finally, the outputs from the two branches are concatenated, and
signal classification is completed via a fully connected layer. Our proposed
method achieves classification accuracies of 85.84
Competition 4-2a and BCI Competition 4-2b datasets, respectively, surpassing
existing state-of-the-art models. The source code is available at
https://github.com/xicheng105/EEG-DBNet.
MoreTranslated text
AI Read Science
Must-Reading Tree
Example
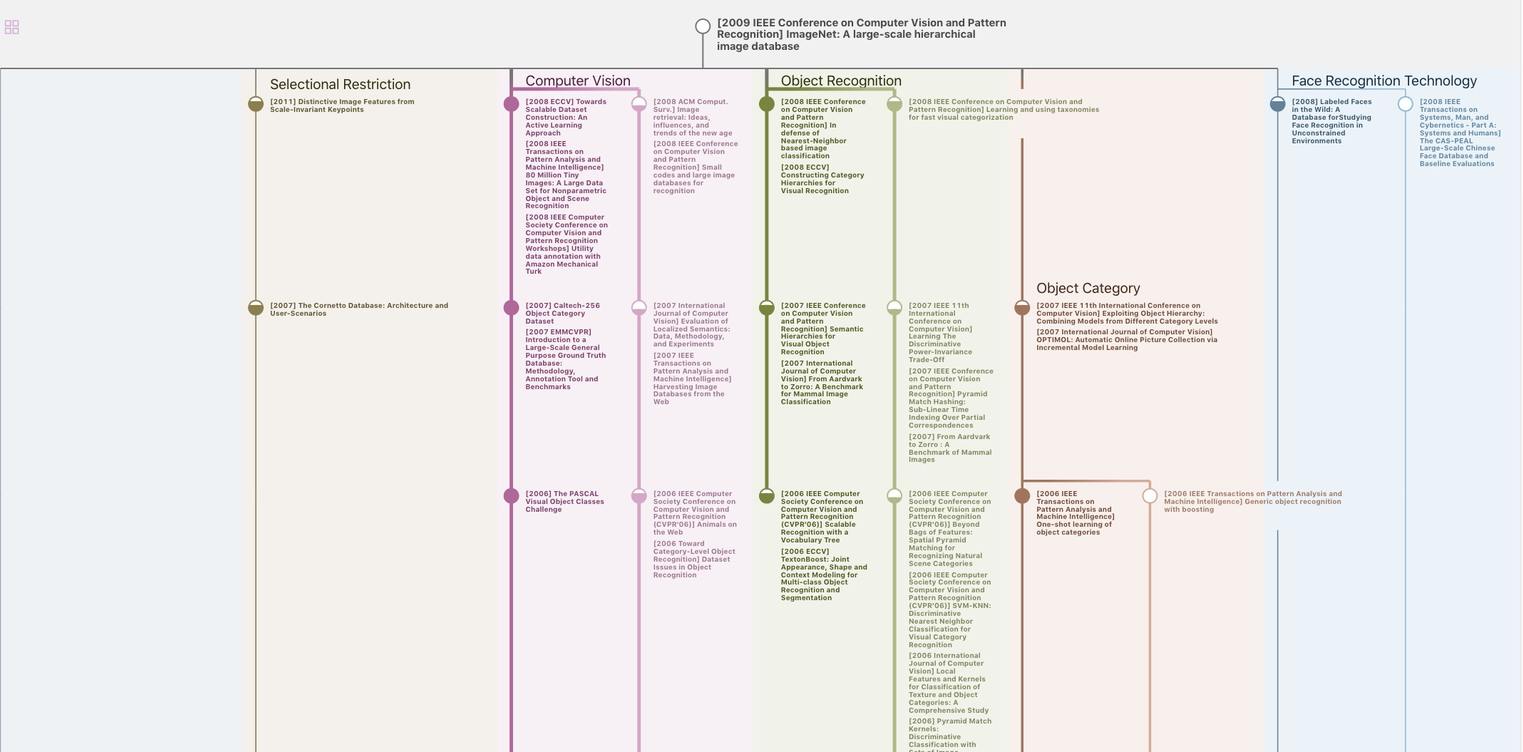
Generate MRT to find the research sequence of this paper
Chat Paper
Summary is being generated by the instructions you defined