VADER: Visual Affordance Detection and Error Recovery for Multi Robot Human Collaboration
CoRR(2024)
摘要
Robots today can exploit the rich world knowledge of large language models to
chain simple behavioral skills into long-horizon tasks. However, robots often
get interrupted during long-horizon tasks due to primitive skill failures and
dynamic environments. We propose VADER, a plan, execute, detect framework with
seeking help as a new skill that enables robots to recover and complete
long-horizon tasks with the help of humans or other robots. VADER leverages
visual question answering (VQA) modules to detect visual affordances and
recognize execution errors. It then generates prompts for a language model
planner (LMP) which decides when to seek help from another robot or human to
recover from errors in long-horizon task execution. We show the effectiveness
of VADER with two long-horizon robotic tasks. Our pilot study showed that VADER
is capable of performing complex long-horizon tasks by asking for help from
another robot to clear a table. Our user study showed that VADER is capable of
performing complex long-horizon tasks by asking for help from a human to clear
a path. We gathered feedback from people (N=19) about the performance of the
VADER performance vs. a robot that did not ask for help.
https://google-vader.github.io/
更多查看译文
AI 理解论文
溯源树
样例
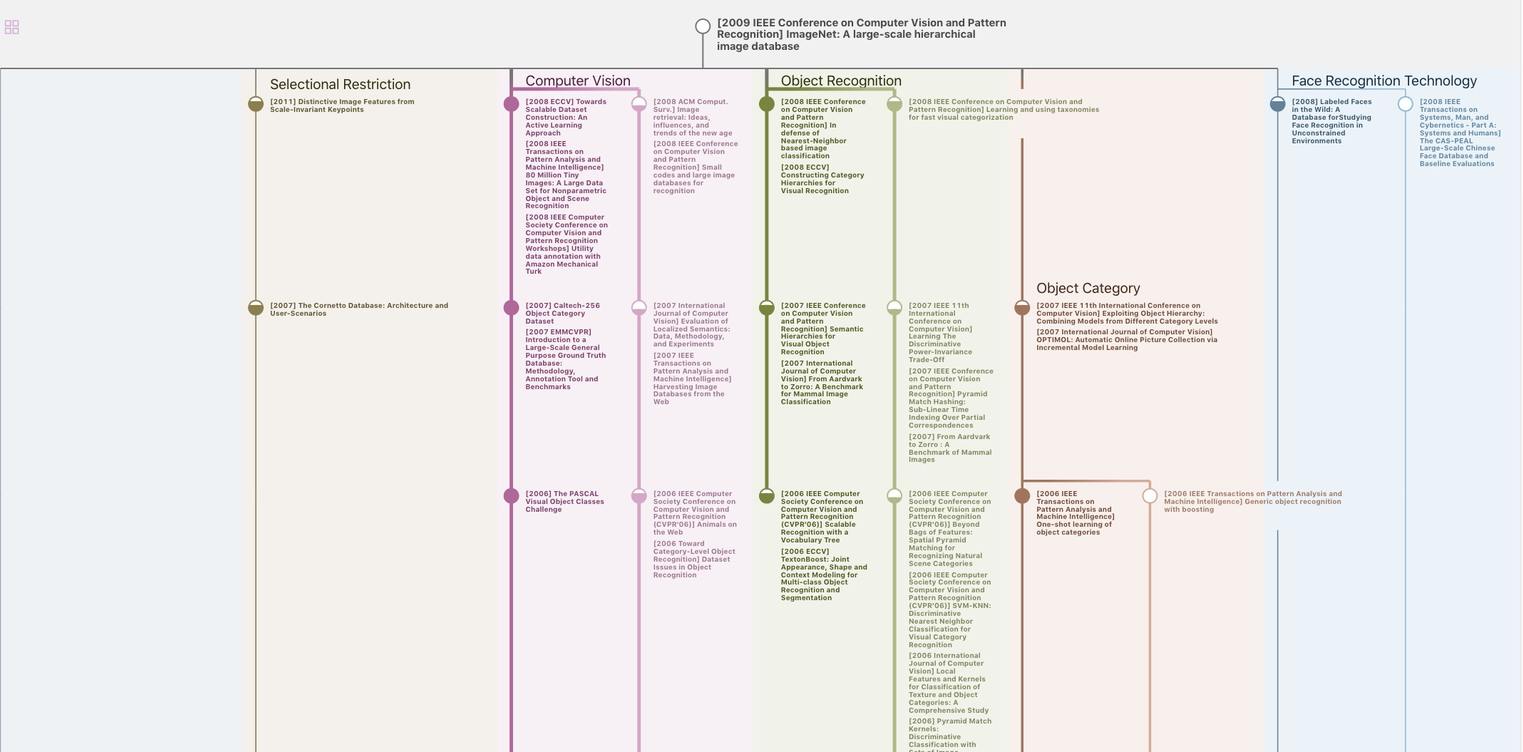
生成溯源树,研究论文发展脉络
Chat Paper
正在生成论文摘要