A Two-Layer Encoding Learning Swarm Optimizer Based on Frequent Itemsets for Sparse Large-Scale Multi-Objective Optimization
IEEE/CAA Journal of Automatica Sinica(2024)
Abstract
Traditional large-scale multi-objective optimization algorithms(LSMOEAs)encounter difficulties when dealing with sparse large-scale multi-objective optimization problems(SLM-OPs)where most decision variables are zero.As a result,many algorithms use a two-layer encoding approach to optimize binary variable Mask and real variable Dec separately.Nevertheless,existing optimizers often focus on locating non-zero variable posi-tions to optimize the binary variables Mask.However,approxi-mating the sparse distribution of real Pareto optimal solutions does not necessarily mean that the objective function is optimized.In data mining,it is common to mine frequent itemsets appear-ing together in a dataset to reveal the correlation between data.Inspired by this,we propose a novel two-layer encoding learning swarm optimizer based on frequent itemsets(TELSO)to address these SLMOPs.TELSO mined the frequent terms of multiple particles with better target values to find mask combinations that can obtain better objective values for fast convergence.Experi-mental results on five real-world problems and eight benchmark sets demonstrate that TELSO outperforms existing state-of-the-art sparse large-scale multi-objective evolutionary algorithms(SLMOEAs)in terms of performance and convergence speed.
MoreTranslated text
Key words
Evolutionary algorithms,learning swarm optimiza-tion,sparse large-scale optimization,sparse large-scale multi-objec-tive problems,two-layer encoding
AI Read Science
Must-Reading Tree
Example
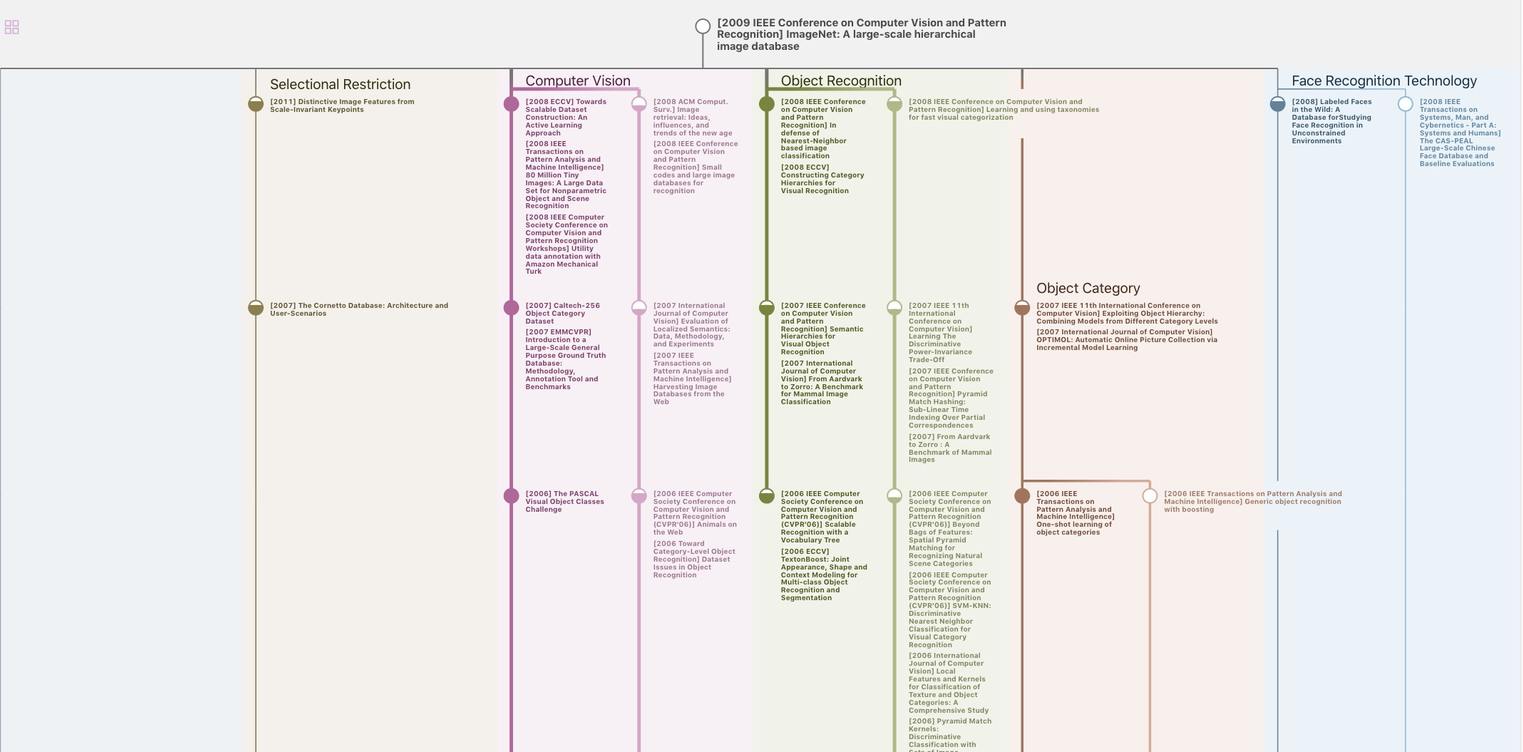
Generate MRT to find the research sequence of this paper
Chat Paper
Summary is being generated by the instructions you defined