Distinguish Any Fake Videos: Unleashing the Power of Large-scale Data and Motion Features
CoRR(2024)
Abstract
The development of AI-Generated Content (AIGC) has empowered the creation of
remarkably realistic AI-generated videos, such as those involving Sora.
However, the widespread adoption of these models raises concerns regarding
potential misuse, including face video scams and copyright disputes. Addressing
these concerns requires the development of robust tools capable of accurately
determining video authenticity. The main challenges lie in the dataset and
neural classifier for training. Current datasets lack a varied and
comprehensive repository of real and generated content for effective
discrimination. In this paper, we first introduce an extensive video dataset
designed specifically for AI-Generated Video Detection (GenVidDet). It includes
over 2.66 M instances of both real and generated videos, varying in categories,
frames per second, resolutions, and lengths. The comprehensiveness of GenVidDet
enables the training of a generalizable video detector. We also present the
Dual-Branch 3D Transformer (DuB3D), an innovative and effective method for
distinguishing between real and generated videos, enhanced by incorporating
motion information alongside visual appearance. DuB3D utilizes a dual-branch
architecture that adaptively leverages and fuses raw spatio-temporal data and
optical flow. We systematically explore the critical factors affecting
detection performance, achieving the optimal configuration for DuB3D. Trained
on GenVidDet, DuB3D can distinguish between real and generated video content
with 96.77
types.
MoreTranslated text
AI Read Science
Must-Reading Tree
Example
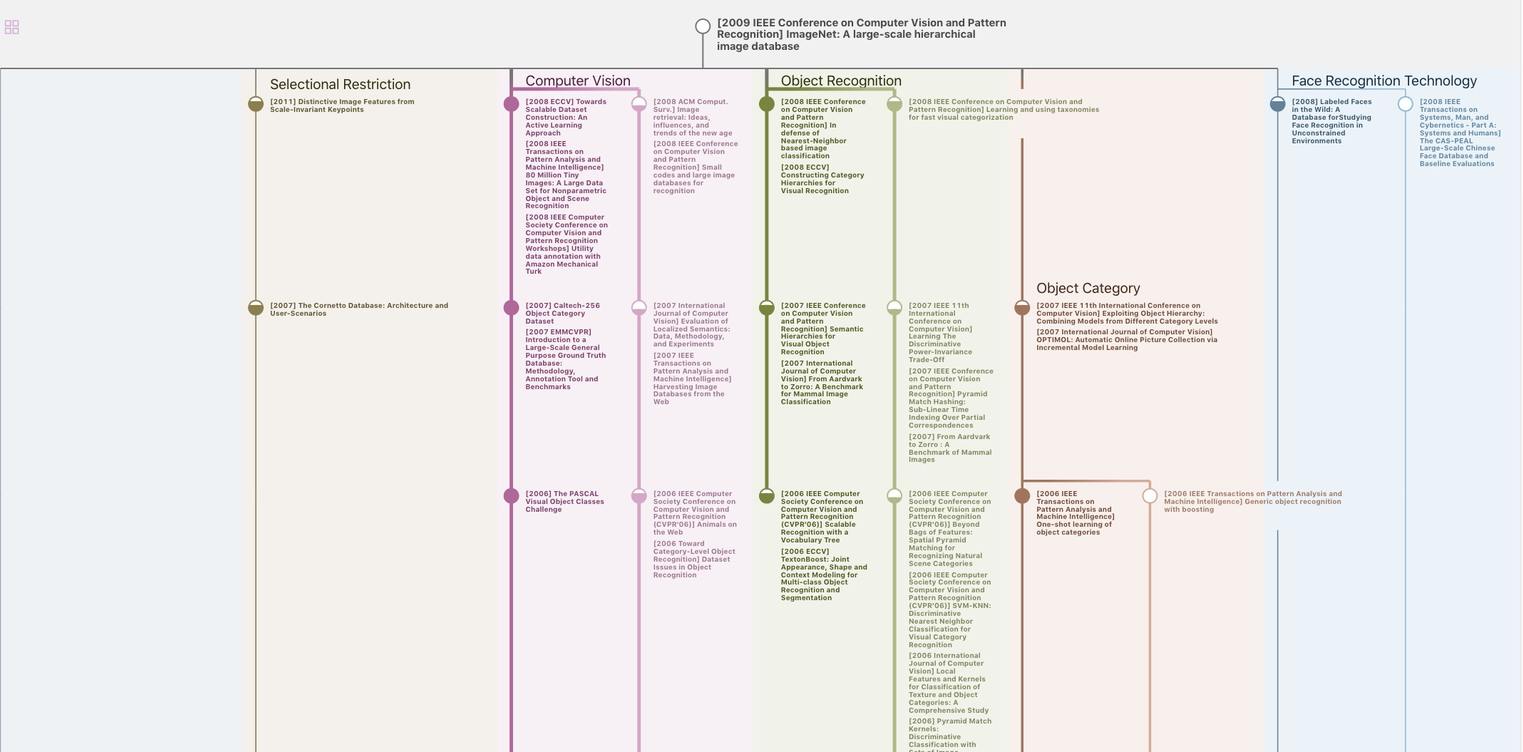
Generate MRT to find the research sequence of this paper
Chat Paper
Summary is being generated by the instructions you defined