Rethinking Class-Incremental Learning from a Dynamic Imbalanced Learning Perspective
CoRR(2024)
Abstract
Deep neural networks suffer from catastrophic forgetting when continually
learning new concepts. In this paper, we analyze this problem from a data
imbalance point of view. We argue that the imbalance between old task and new
task data contributes to forgetting of the old tasks. Moreover, the increasing
imbalance ratio during incremental learning further aggravates the problem. To
address the dynamic imbalance issue, we propose Uniform Prototype Contrastive
Learning (UPCL), where uniform and compact features are learned. Specifically,
we generate a set of non-learnable uniform prototypes before each task starts.
Then we assign these uniform prototypes to each class and guide the feature
learning through prototype contrastive learning. We also dynamically adjust the
relative margin between old and new classes so that the feature distribution
will be maintained balanced and compact. Finally, we demonstrate through
extensive experiments that the proposed method achieves state-of-the-art
performance on several benchmark datasets including CIFAR100, ImageNet100 and
TinyImageNet.
MoreTranslated text
AI Read Science
Must-Reading Tree
Example
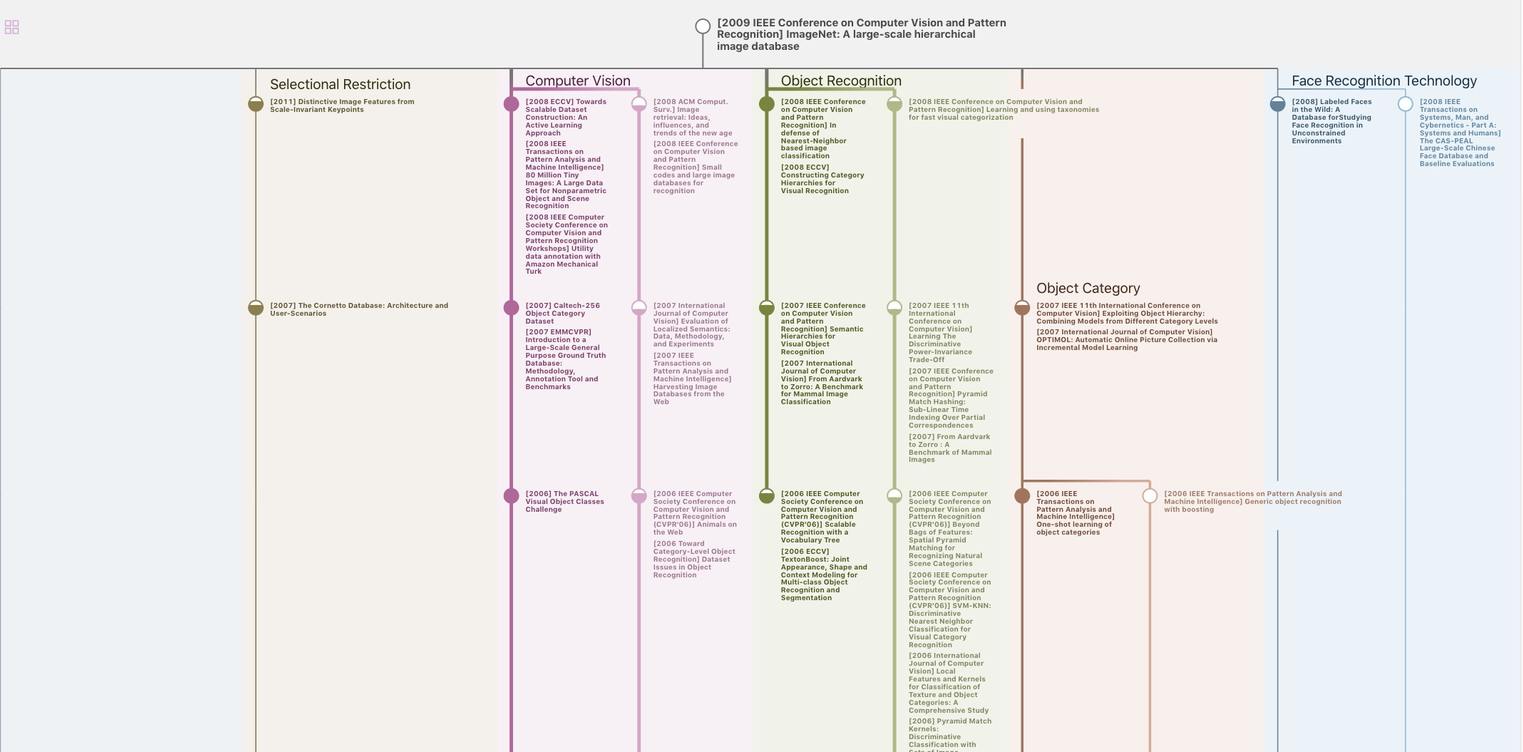
Generate MRT to find the research sequence of this paper
Chat Paper
Summary is being generated by the instructions you defined