Intelligent Approaches for Predicting the Intact Rock Mechanical Parameters and Crack Stress Thresholds
Rock Mechanics and Rock Engineering(2024)
摘要
This study aims to make a unique contribution to the existing body of knowledge about rock strength and deformation parameters and crack stress thresholds through intelligent and statistical approaches applied to a database comprising various rock types (i.e., sedimentary, igneous, and metamorphic rocks). The database contains physical–mechanical and ultrasonic parameters. Six distinct machine learning (ML) algorithms— artificial neural network (ANN), random forest (RF), decision tree (DT), K-nearest neighbor (KNN), support vector regression (SVR), and bagging regressor (BR)— along with the conventional linear regression techniques, were employed to develop predictive models. These models estimate uniaxial compressive strength ( σ_c ) and Tangent Young’s modulus ( E_t ) based on bulk density ( ρ ) and P-wave ultrasonic velocity ( V_p ). Furthermore, they predict crack stress thresholds (i.e., crack closure stress σ_cc , crack initiation stress σ_ci , and crack damage stress σ_cd ) as a function of σ_c , E_t , ρ , V_p , axial strain at failure ( ε_1f ), and lateral strain at failure ( ε_3f ). Various performance indices were utilized to evaluate and compare the performance of these models. The results indicated that the RF method outperformed other ML-based and linear regression-based approaches in predicting the output parameters. Additionally, the multiple parametric sensitivity analysis (MPSA) was carried out to determine the significance of input parameters in predicting the output variables. This analysis revealed that V_p and ρ have the highest and lowest impact on predicting σ_c and E_t , respectively. On the other hand, σ_c was identified as the most influential parameter in predicting σ_ci and σ_cd , while parameters ε_3f and V_p showed the least impact on the foregoing outputs, respectively. This is while ε_1f and ρ were, respectively, found as the most important and least important factors in predicting σ_cc . Finally, to facilitate easy access to the prediction results and enhance the practicality of the proposed RF model, a graphical user interface (GUI) was developed, which enables the practical application of the most performing developed prediction model.
更多查看译文
关键词
Crack threshold stresses,Intact rock,Rock strength,Young’s modulus,Machine learning,Random forest
AI 理解论文
溯源树
样例
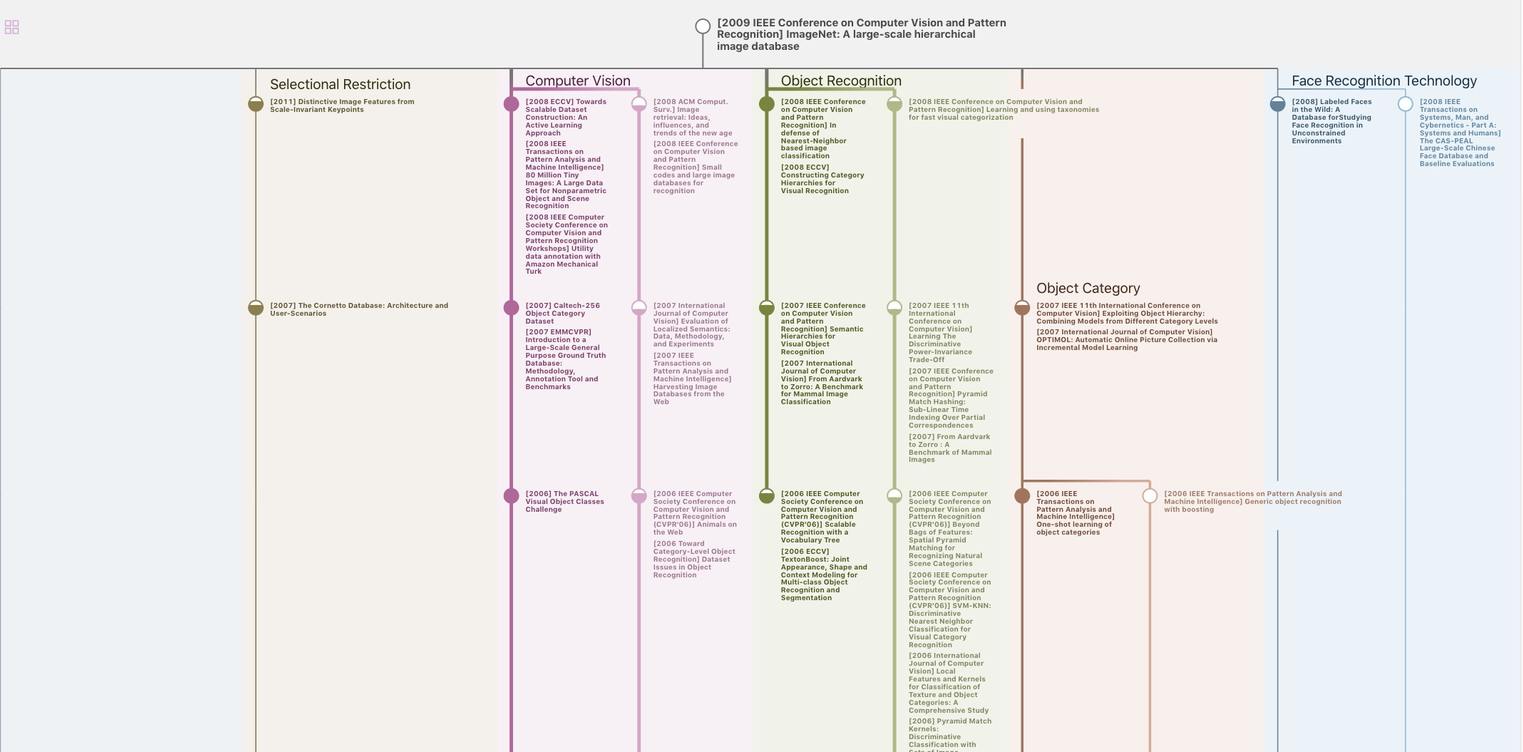
生成溯源树,研究论文发展脉络
Chat Paper
正在生成论文摘要