NetHD: Neurally Inspired Integration of Communication and Learning in Hyperspace
ADVANCED INTELLIGENT SYSTEMS(2024)
摘要
The 6G network, the next-generation communication system, is envisaged to provide unprecedented experience through hyperconnectivity involving everything. The communication should hold artificial intelligence-centric network infrastructures as interconnecting a swarm of machines. However, existing network systems use orthogonal modulation and costly error correction code; they are very sensitive to noise and rely on many processing layers. These schemes impose significant overhead on low-power internet of things devices connected to noisy networks. Herein, a hyperdimensional network-based system, called NetHD$N e t H D$, is proposed, which enables robust and efficient data communication/learning. NetHD$N e t H D$ exploits a redundant and holographic representation of hyperdimensional computing (HDC) to design highly robust data modulation, enabling two functionalities on transmitted data: 1) an iterative decoding method that translates the vector back to the original data without error correction mechanisms, or 2) a native hyperdimensional learning technique on transmitted data with no need for costly data decoding. A hardware accelerator that supports both data decoding and hyperdimensional learning using a unified accelerator is also developed. The evaluation shows that NetHD$N e t H D$ provides a bit error rate comparable to that of state-of-the-art modulation schemes while achieving 9.4 x$\times$ faster and 27.8 x$\times$ higher energy efficiency compared to state-of-the-art deep learning systems. The 6G network, the next-generation communication system, is envisaged to provide unprecedented experience through hyperconnectivity involving everything. The communication should hold artificial intelligence-centric network infrastructures as interconnecting a swarm of machines. However, existing network systems use orthogonal modulation and costly error correction code; they are very sensitive to noise and rely on many processing layers. These schemes impose significant overhead on low-power internet of things devices connected to noisy networks. Herein, a hyperdimensional network-based system, called NetHD, is proposed, which enables robust and efficient data communication/learning. Unlike existing communication protocols that use layered processing for data communication, NetHD fundamentally integrates communication with machine learning. NetHD exploits a redundant and holographic representation of hyperdimensional computing (HDC) to design highly robust data modulation. As HDC encoding spreads the data over a high-dimensional vector, a substantial number of bits can be corrupted while preserving sufficient information, resulting in high noise robustness. NetHD enables two functionalities on transmitted data: 1) an iterative decoding method that translates the vector back to the original data without error correction mechanisms, or 2) a native hyperdimensional learning technique on transmitted data with no need for costly data decoding. A hardware accelerator that supports both data decoding and hyperdimensional learning using a unified accelerator is also developed. The evaluation shows that NetHD provides a bit error rate comparable to that of state-of-the-art modulation schemes while achieving 9.4x faster and 27.8x higher energy efficiency compared to state-of-the-art deep learning systems.image (c) 2024 WILEY-VCH GmbH
更多查看译文
关键词
artificial intelligent,channel computing,energy efficiency,hyperdimensional computing
AI 理解论文
溯源树
样例
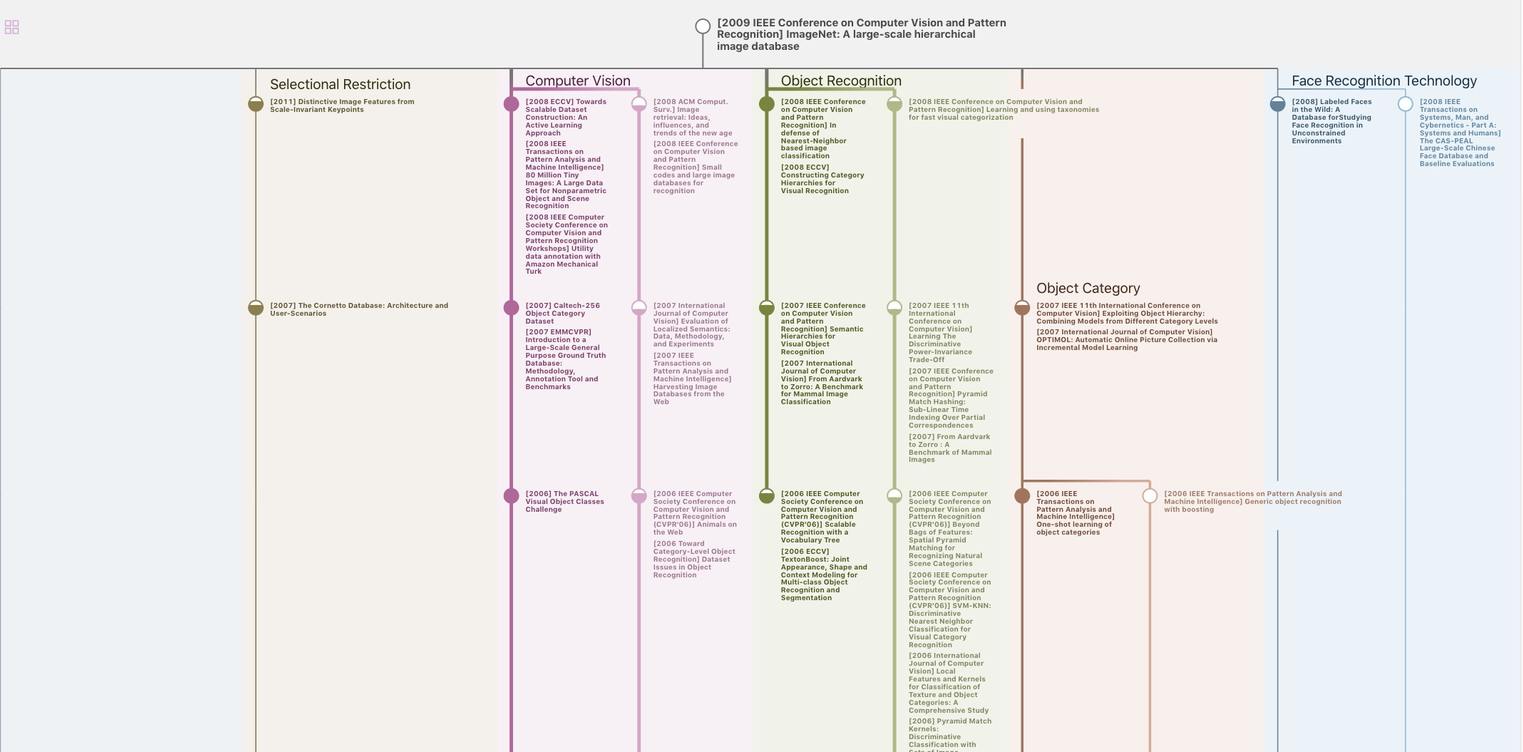
生成溯源树,研究论文发展脉络
Chat Paper
正在生成论文摘要