A predictive framework for PEMFC dynamic load performance degradation based on feature parameter analysis
International Journal of Hydrogen Energy(2024)
Abstract
Accurate and swift estimation of the future performance degradation of Proton Exchange Membrane Fuel Cells (PEMFCs) is crucial for the health management of PEMFC. However, most existing studies primarily concentrate on uncovering the inherent patterns of performance degradation parameters, neglecting the fundamental relationships and pattern variations between performance degradation and other characteristic parameters (such as flow rate, temperature, and pressure). This paper seeks to address this research gap. To that end, we delve into feature parameters, extracting underlying patterns of feature evolution to construct a feature exploration-based framework for predicting PEMFC performance degradation. Initially, Time-Varying Filtered Empirical Mode Decomposition (TVF-EMD) is employed to decompose each feature variable, isolating the core information of the features. Furthermore, to reduce the computational burden caused by redundant features, Uniform Manifold Approximation and Projection (UMAP) is used to perform dimensionality reduction on the decomposed feature subsequences, ensuring the efficiency and representativeness of the extracted features. Finally, to overcome the shortcomings of long short-term memory networks (LSTM) in terms of convergence and the effective use of time series information, we introduce an innovative Mogrifier LSTM (M-LSTM) model to enhance the accuracy of PEMFC performance degradation predictions. The experimental and validation results demonstrate that the extraction of feature parameters significantly enhances the predictive precision of the baseline model. Relative to the compared models, there was an improvement of at least 16.859% in Root Mean Square Error (RMSE), a minimum increase of 23.333% in Mean Absolute Error (MAE), and a boost of no less than 0.913% in the coefficient of determination (R2). Simultaneously, the proposed predictive framework demonstrates good stability and accuracy in predicting PEMFC performance degradation.
MoreTranslated text
Key words
Proton exchange membrane fuel cell,Performance degradation trend,Deep learning,Feature engineering
AI Read Science
Must-Reading Tree
Example
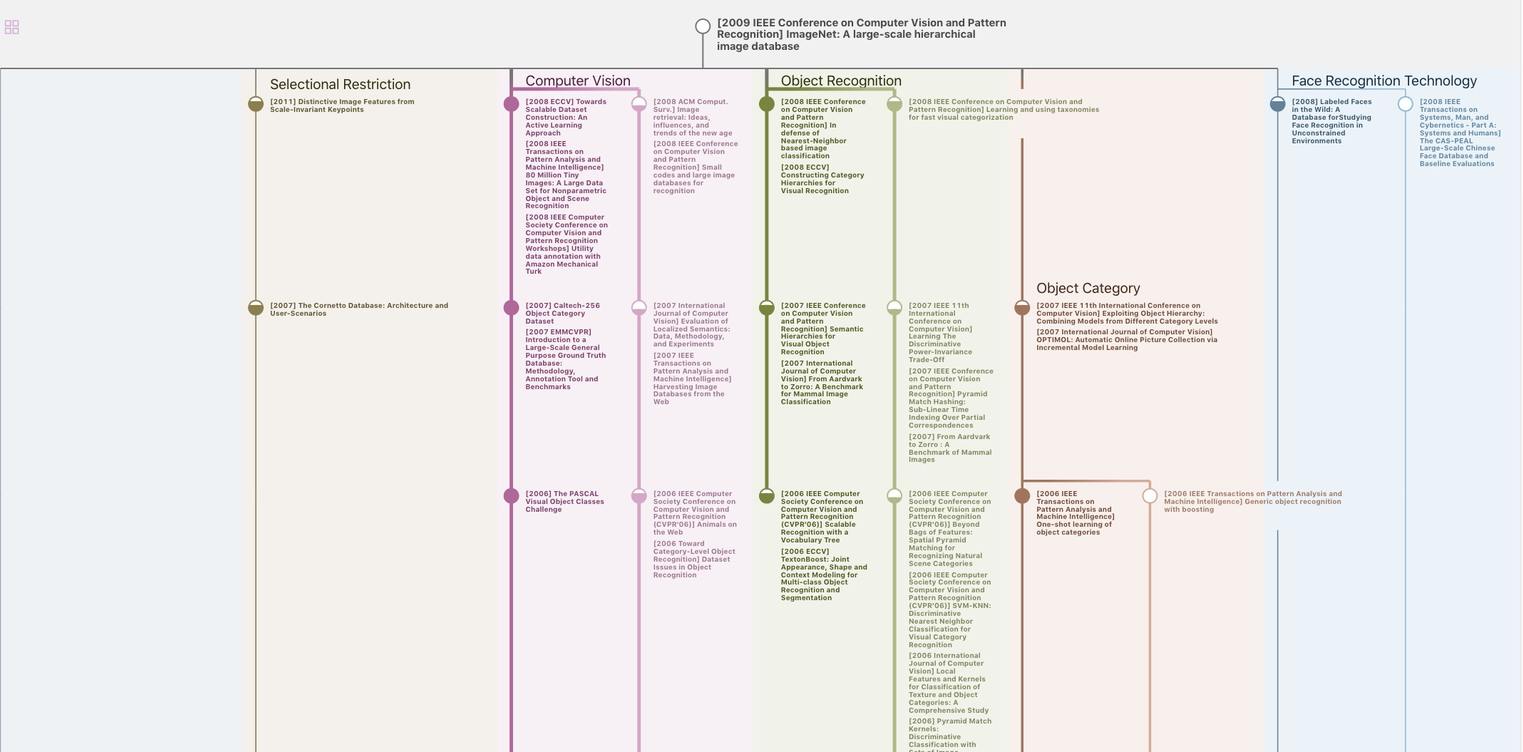
Generate MRT to find the research sequence of this paper
Chat Paper
Summary is being generated by the instructions you defined