Bridging the simulation-to-real gap of depth images for deep reinforcement learning
Expert Systems with Applications(2024)
摘要
While deep reinforcement learning (DRL) models are effective at learning appropriate actions from high-dimensional data, they require large amounts of costly and time-consuming training data to be collected in real-world settings. For this reason, collecting data in simulations offers a promising alternative, but transferring policy networks from simulation to reality can be challenging due to differences in perception between the virtual and real worlds. This paper proposes a two-level method to bridge the simulation-to-reality (sim-to-real) gap for depth images, specifically for autonomous environmental navigation that uses DRL. Simulated depth images are first translated at a perception level through generative adversarial network (GAN) to make them look like real data from a depth sensor. Simulated and GAN-generated depth images are encoded into latent representations, and the encoder is trained in the latent space to make the two images paired. This encoder is trained simultaneously with a reinforcement learning network model to extract domain-invariant and task-relevant features from depth images and map the behavioral similarity of states to the latent space. Our experimental results demonstrate that our approach can effectively bridge the sim-to-real gap, enabling policies learned in simulation to maintain their control performance in the real world.
更多查看译文
关键词
Autonomous navigation,Depth image,Mobile robot,Sim-to-real
AI 理解论文
溯源树
样例
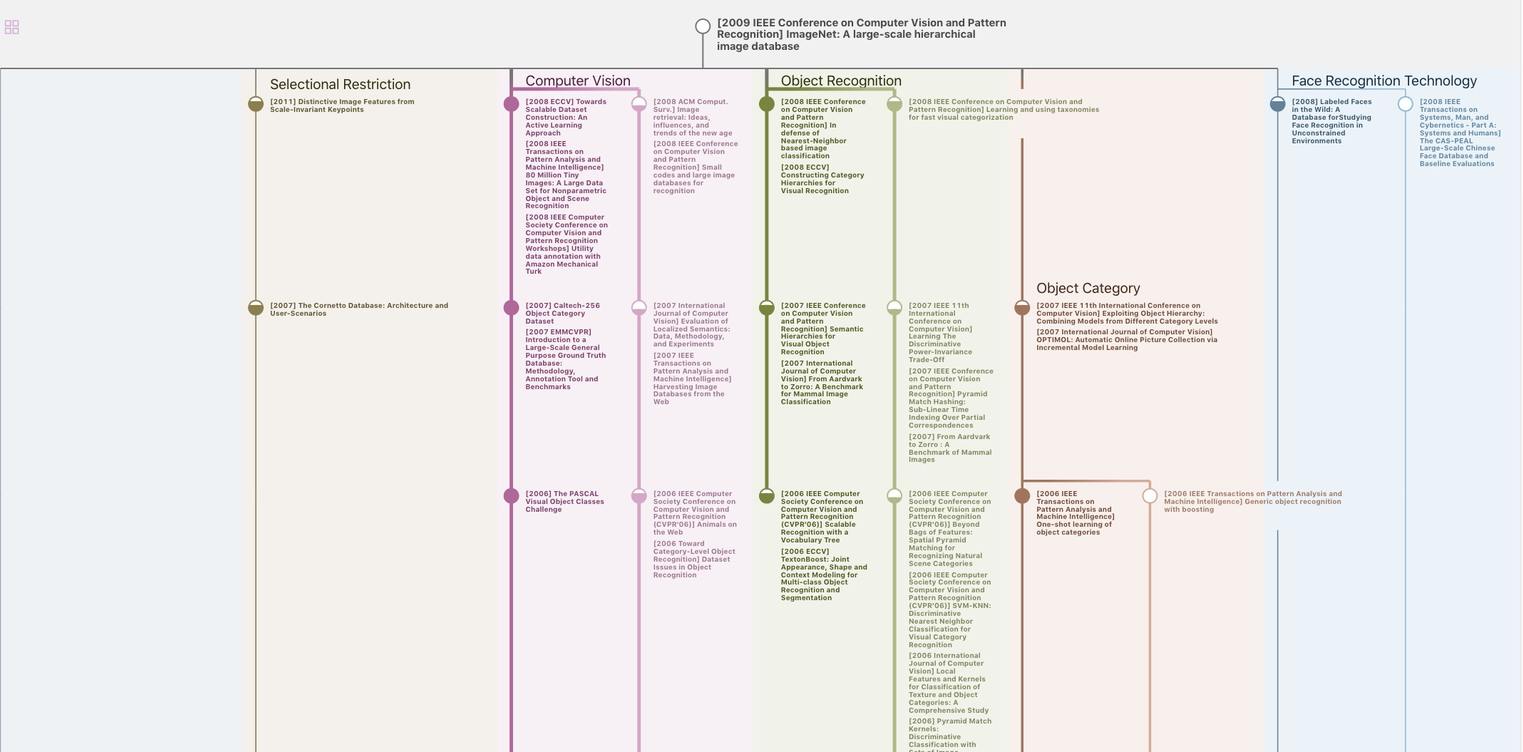
生成溯源树,研究论文发展脉络
Chat Paper
正在生成论文摘要