A predictive analytics framework for rolling bearing vibration signal using deep learning and time series techniques
COMPUTERS & ELECTRICAL ENGINEERING(2024)
摘要
Degradation trend prognostics plays an important role in industrial prognostics and health management (PHM), requiring data-driven models with higher predictive capability for accurate long time series prediction. Nevertheless, typical convolutional neural network (CNN), recurrent neural network (RNN) and their variants still fall short in feature extraction and temporal complexity analysis, which makes them difficult to capture the overall characteristics/distribution of the time series. Recent studies have revealed the significant impact of Transformer-based frameworks in sequence processing, demonstrating their potential to enhance predictive capabilities. Inspired by the above, a new Frequency Enhanced Crossformer (FEC) framework is proposed for accurately predicting vibrations during the bearing degradation stage. The proposed framework contains two important feature extraction mechanisms, which are temporal and dimensional attention mechanisms and frequency enhanced block. These mechanisms are designed to capture the temporal dependence, dimensional dependence and frequency domain features of the bearing vibration data, respectively. Then, bearing vibration prediction is performed on two publicly available bearing datasets to verify the effectiveness and robustness of the proposed framework. The results show that the Frequency Enhanced Crossformer can accurately predict the future time series of bearing vibration under different operating conditions compared to other commonly used algorithms. In addition, the significance and necessity of the two blocks are demonstrated by ablation experiments.
更多查看译文
关键词
Bearing vibration,Sensor data,Crossformer,Transformer,Time series forecasting
AI 理解论文
溯源树
样例
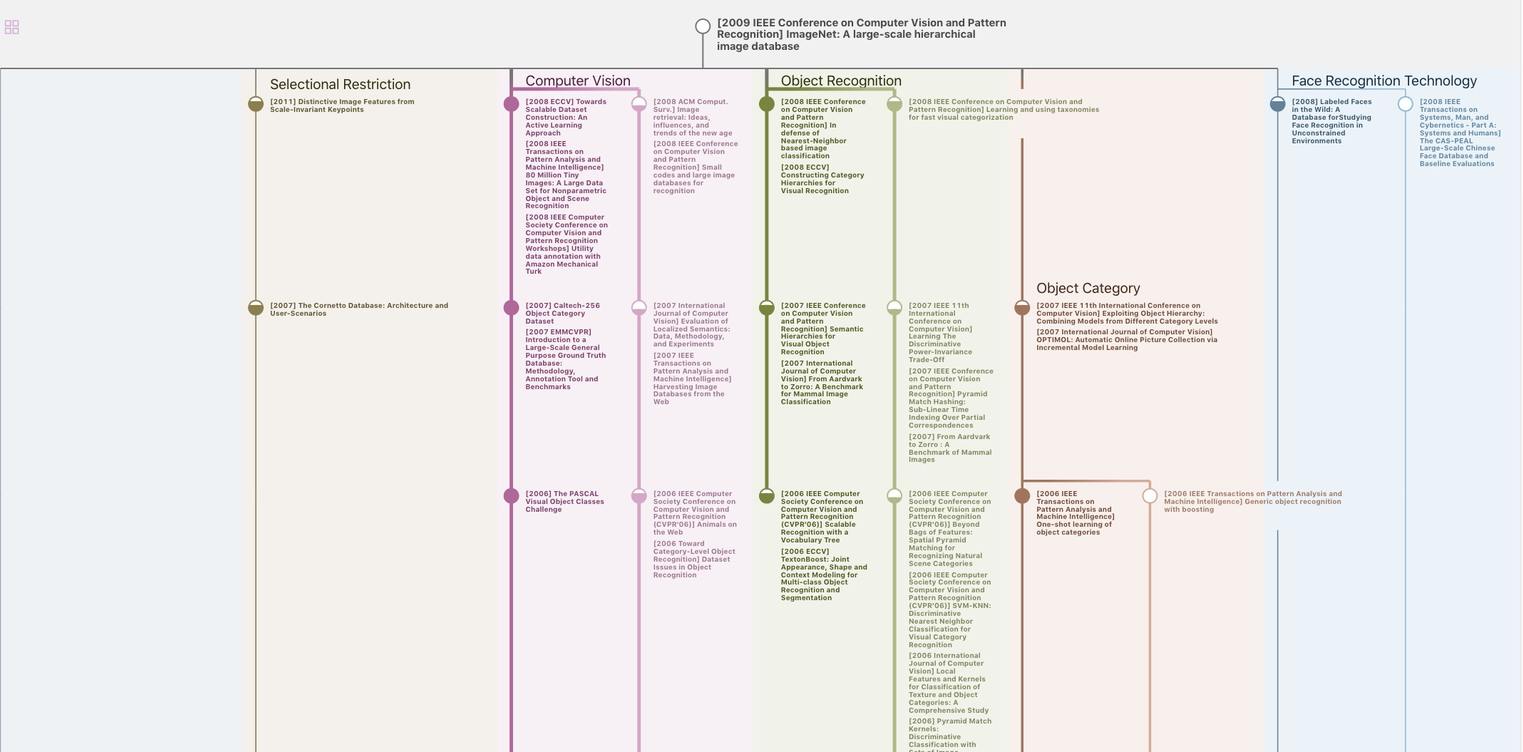
生成溯源树,研究论文发展脉络
Chat Paper
正在生成论文摘要