Artificial Intelligence to Assist in the Screening Fetal Anomaly Ultrasound Scan (PROMETHEUS): A Randomised Controlled Trial
Thomas George Day,Jacqueline Matthew,Samuel F Budd, Alfonso Farruggia, Lorenzo Venturini,Robert Wright,Babak Jamshidi,Meekai To, Huazen Ling, Jonathon Lai,Min Yi Tan, Matthew Brown,Gavin Guy,Davide Casagrandi,Anastasija Arechvo,Argyro Syngelaki,David Lloyd,Vita Zidere,Trisha Vigneswaran,Owen Miller,Ranjit Akolekar,Surabhi Nanda,Kypros Nicolaides,Bernhard Kainz,John M Simpson,Jo V Hajnal,Reza Razavi Ultrasound in Obstetrics and Gynecology(2024)
AI 理解论文
溯源树
样例
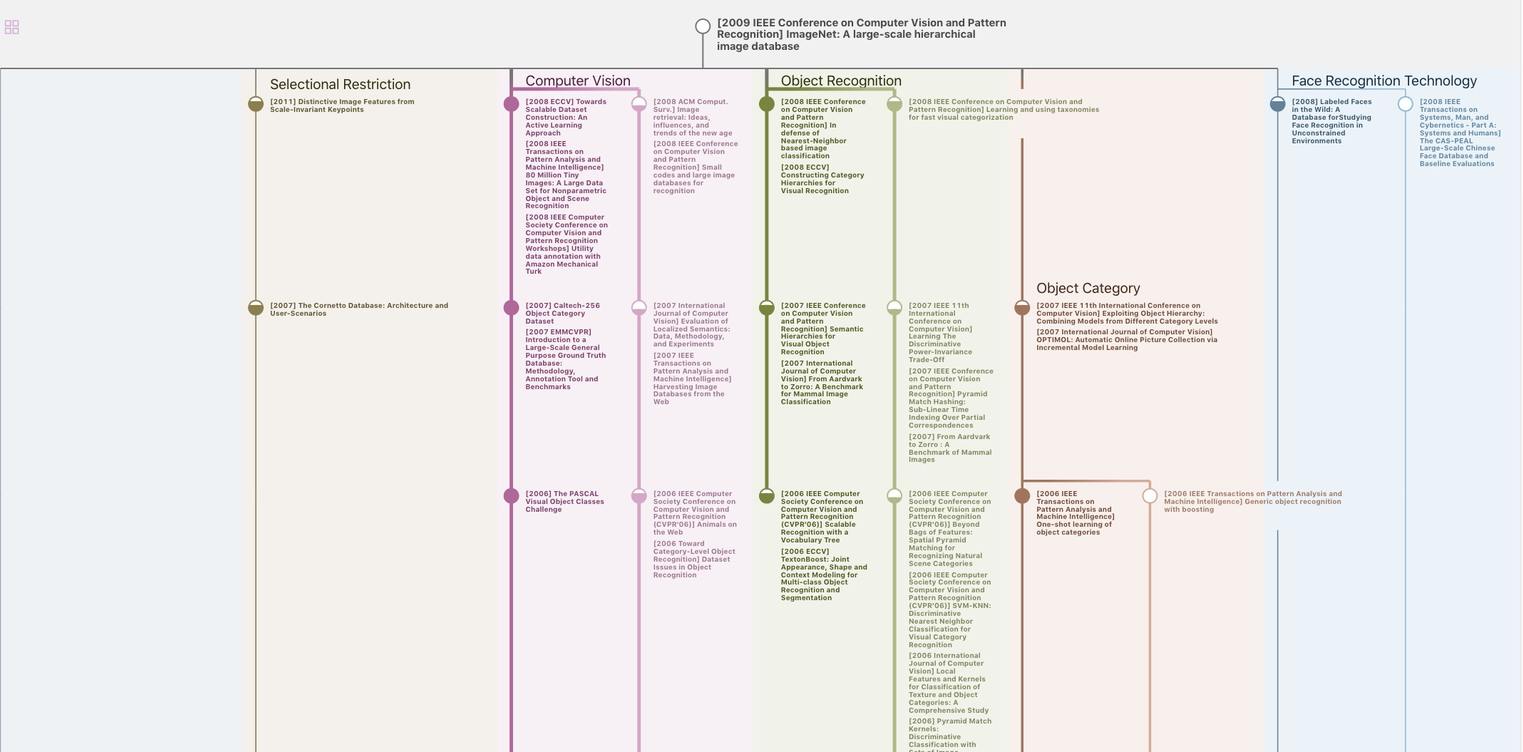