Cross-dataset evaluation of wearable fall detection systems using data from real falls and long-term monitoring of daily life
Measurement(2024)
Abstract
The evaluation of fall detection systems based on wearables is controversial as most studies in the literature benchmark their proposals against falls that are simulated by experimental subjects under unrealistic laboratory conditions. In order to systematically investigate the suitability of this procedure, this paper evaluates a wide set of artificial intelligence algorithms used for fall detection, when trained with a large number of datasets containing acceleration samples captured during the emulation of falls and ordinary movements and then tested with the signals of both actual falls and long-term traces collected from the constant monitoring of users during their daily routines. The results, based on a large number of repositories, show a remarkable degradation in all performance metrics (sensitivity, specificity and false alarm hourly rate) with respect to the typical case in which the detectors are tested with the same types of laboratory movements for which they were trained.
MoreTranslated text
Key words
Fall Detection Systems,Inertial Sensors,Wearables,Accelerometer,Dataset,Machine learning,Deep Learning.
AI Read Science
Must-Reading Tree
Example
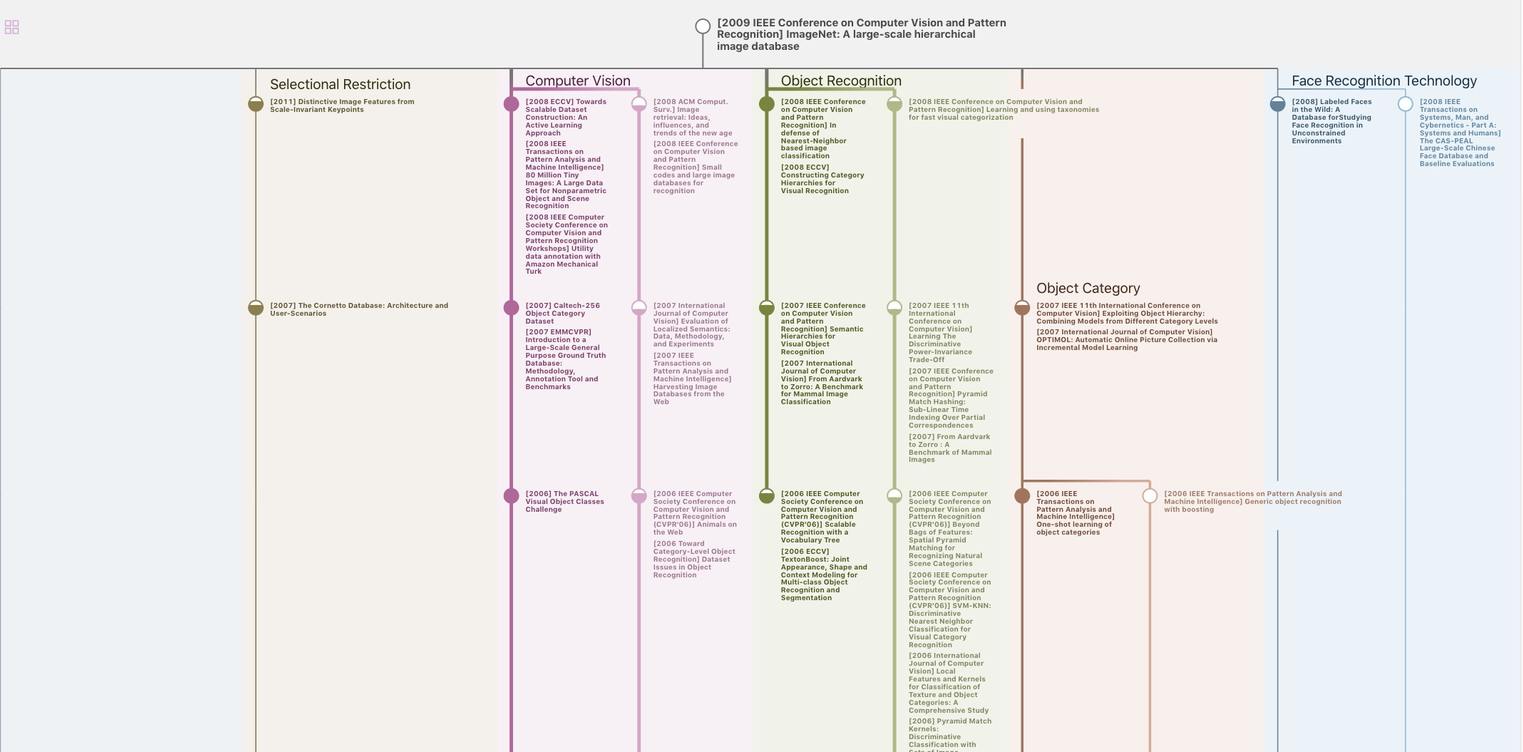
Generate MRT to find the research sequence of this paper
Chat Paper
Summary is being generated by the instructions you defined