Partial Discharge Type Identification Based on PSO Optimised BP-RF Model and Multi-Type Sensors
2024 9th Asia Conference on Power and Electrical Engineering (ACPEE)(2024)
Abstract
For the partial discharge phenomenon occurring during the use of power equipment, the partial discharge data acquired by multiple sensors (HFCT, AE, TEV) are used to correct the PRPD map by image processing techniques, so as to improve the accuracy of partial discharge type identification. The partial discharge signals under different experimental conditions were acquired using HFCT, AE and TEV sensors, and the alignment of PRPD images acquired by different sensors was achieved by image processing. On this basis, the data from individual sensors were preliminary classified using BP neural networks to identify different types of partial discharge patterns; the per-classification results of these individual sensing were input into the Random Forest algorithm model as features, while the Particle Swarm Optimization algorithm was used to search for the optimal hyper-parameters of the Random Forest, and finally, the Random Forest algorithm model of the Particle Swarm Optimization was used to make the final decision and classification. The experimental results show that the method can effectively identify and classify different types of partial discharges, providing a new technical way for power system maintenance and fault prevention.
MoreTranslated text
AI Read Science
Must-Reading Tree
Example
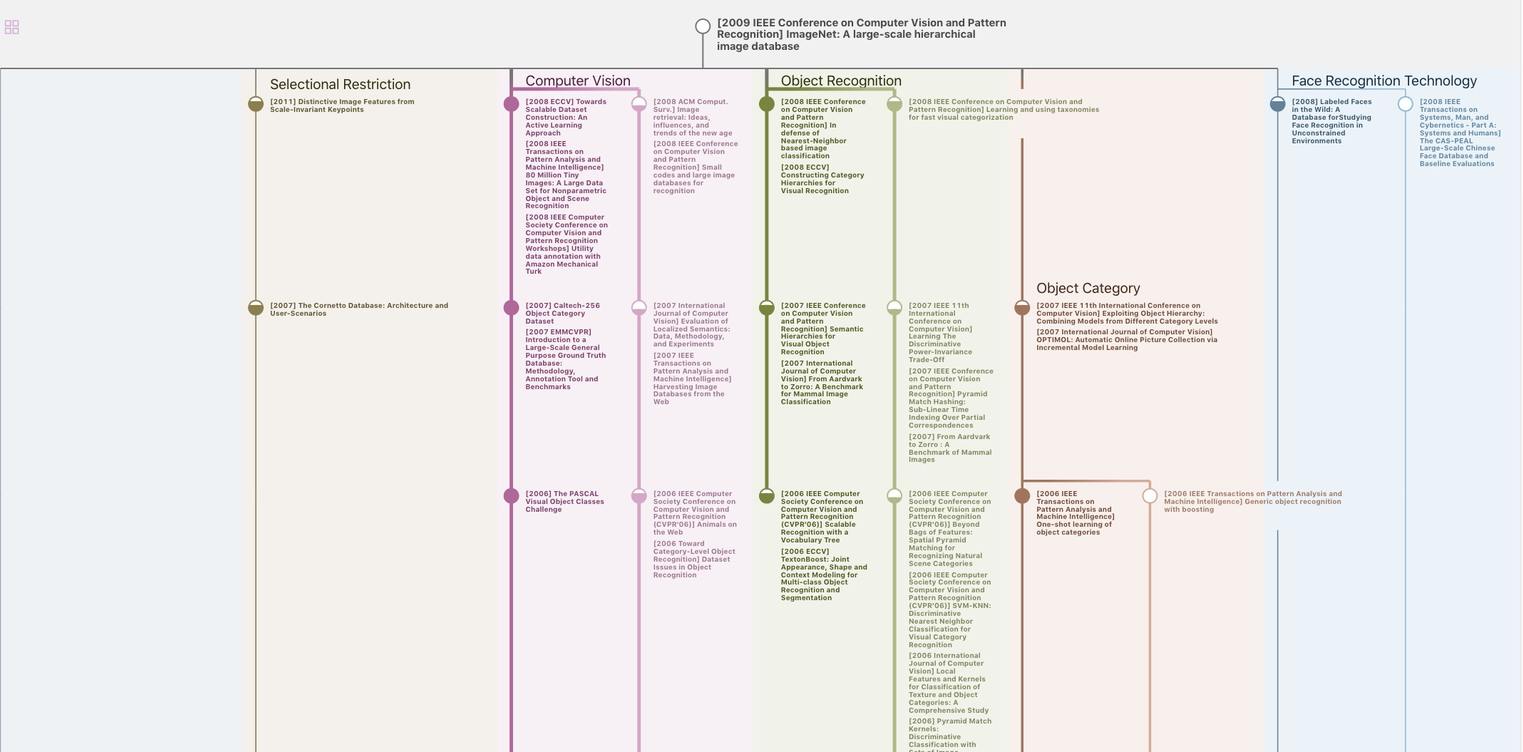
Generate MRT to find the research sequence of this paper
Chat Paper
Summary is being generated by the instructions you defined