CAU-Net: A Convolutional Attention U-Network For Radar Signal Deinterleaving
IEEE Communications Letters(2024)
摘要
The deinterleaving of radar signals is designed to segregate distinct pulses within a composite signal, facilitating the detailed analysis of individual components. This process is pivotal in situational awareness applications, including target signal interception and cognitive jamming antagonism. In the existing electromagnetic environment, the radar emitter pulse modulation parameters will be jitter modulated to improve its anti-identification ability, leading to the lack of integrity of the pulse features obtained from the measurements and the degradation of the deinterleaving performance of the existing methods. In this paper, a Convolutional Attention U-Network (CAU-Net) is proposed to deinterleave the original signal. Guided by the attentional mechanism, the feature of the original signal is extracted by the Attention Down-Sampling (ADS) block without necessitating pulse measurement. Then, it is used to classify the predicted pulses by the Feature Fusion Up-Sampling (FFUS) block, with the prior maximum number of radar emitters in each sample. The experimental results demonstrate the superior performance of the proposed CAU-Net in terms of deinterleaving and complexity, and its robustness is confirmed under various noise conditions.
更多查看译文
关键词
Radar signal deinterleaving,Deep convolutional network,Convolutional attention mechanism,Parameter estimation
AI 理解论文
溯源树
样例
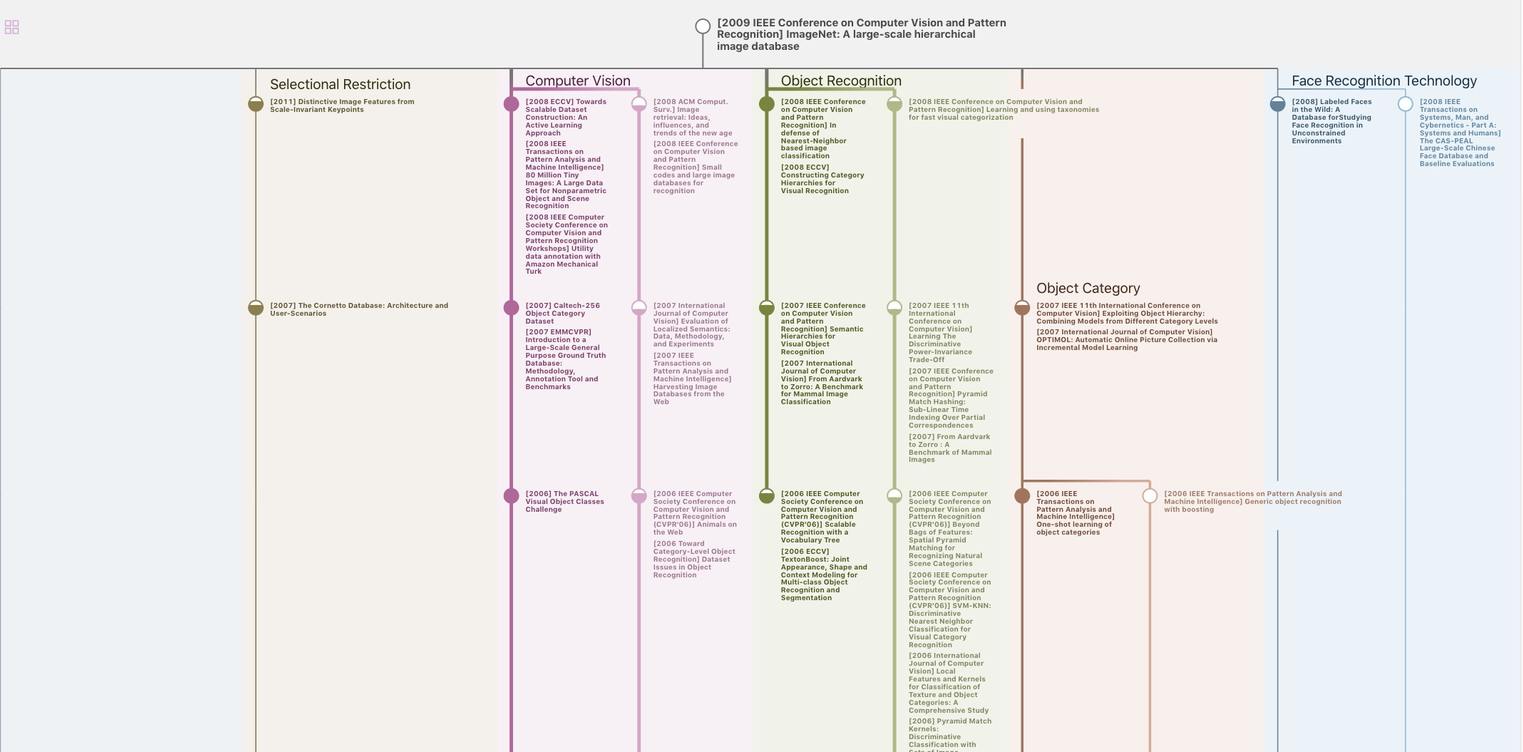
生成溯源树,研究论文发展脉络
Chat Paper
正在生成论文摘要