Integrated machine learning, computational, and experimental investigation of compatibility in oil-modified silicone elastomer coatings
PROGRESS IN ORGANIC COATINGS(2024)
摘要
In this work, we present a novel computational design strategy for coatings, specifically focusing on the compatibility of silicone elastomer coatings modified with silicone oils. We present a novel modeling framework that integrates machine learning (ML), statistical thermodynamics, molecular dynamics simulation, and surface characterization techniques to gain deeper insights on the parameters governing the compatibility of the oil with the elastomer. Our developed machine-learning models identified the key features that influence overall surface energy. Informed by the ML modeling, the in silico miscibility behavior of phenylmethyl silicone oil in a model PDMS matrix was investigated, applying the COnductor-like Screening MOdel for Real Solvents (COSMO-RS). The role of solvents, molecular weight, and temperature on the free energy of mixing Delta Gmix of different siliconeoil and PDMS mixtures is discussed. In addition, computational methods and high-resolution imaging techniques are employed to study the phase-separation behavior of coating surfaces. Through this integrated framework, it was possible to gain molecular insights into the thermodynamic and kinetic attributes of silicone elastomers, which play a crucial role in determining the characteristics of the PDMS surface. The findings can be used as a tool to relate the silicon oil and elastomer compatibility with fouling-release performance and further as a guiding tool to enhance the fouling release performance of this specific class of coatings in marine environments. Ultimately, we believe our approach provides a foundation for advanced design strategies of polymeric coating materials.
更多查看译文
关键词
Silicone elastomer coatings,Surface energy,Machine learning,Structure-property relationship,Silicon oil-modified,Modeling
AI 理解论文
溯源树
样例
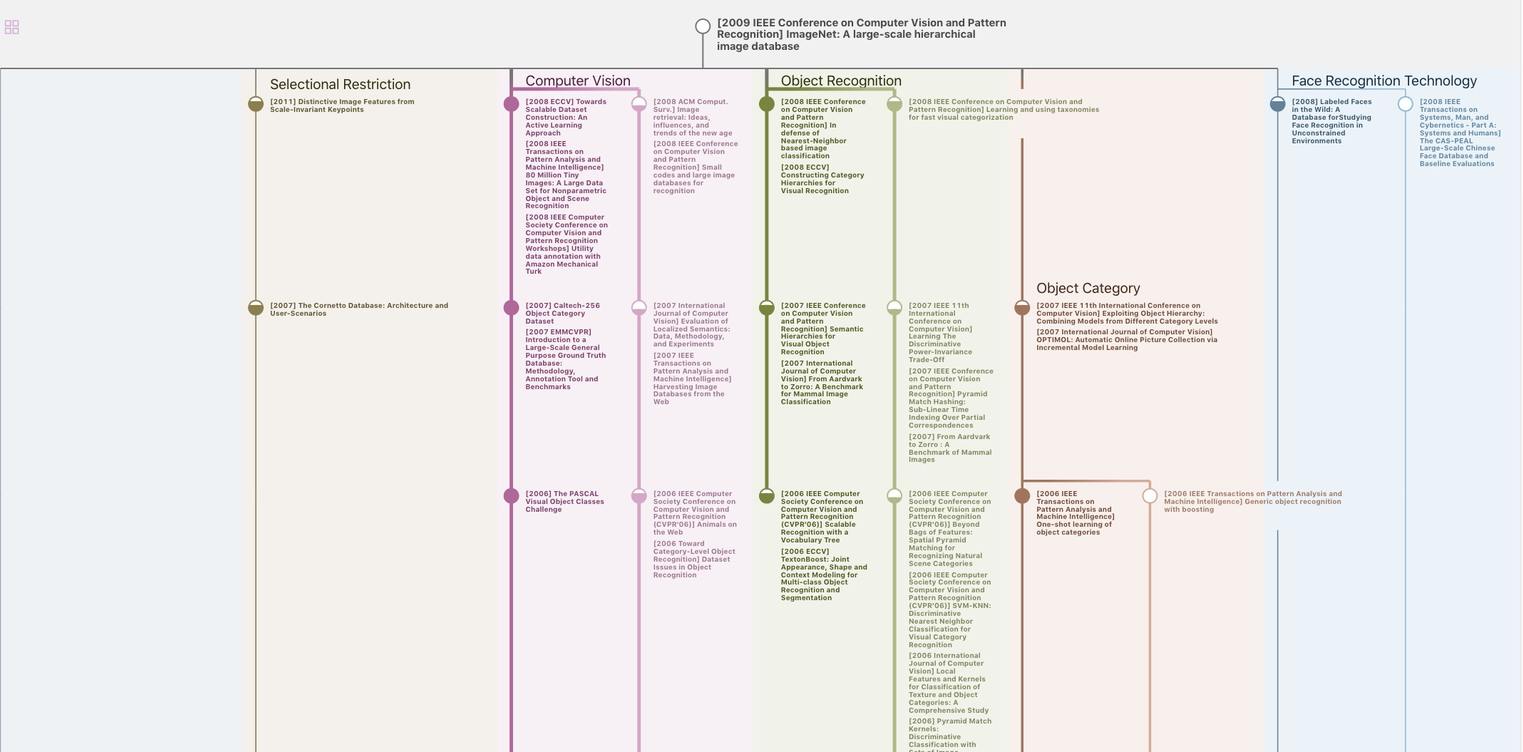
生成溯源树,研究论文发展脉络
Chat Paper
正在生成论文摘要