Predictive Modelling of Base Station Energy Consumption:Insights and Application of STL-LSTM Networks
2024 9th Asia Conference on Power and Electrical Engineering (ACPEE)(2024)
摘要
The increasing demand for wireless communication services has led to a significant growth in the number of base stations, resulting in a substantial increase in energy consumption. Understanding and predicting base station energy consumption is important for optimizing energy usage and developing sustainable communication networks. This study introduces a predictive modeling approach for base station energy consumption by combining Seasonal and Trend decomposition using Loess (STL) and Long Short-Term Memory (LSTM) networks. The methodology involves decomposing the historical data into seasonal, trend, and residual components using STL. LSTM is then utilized to model the relationships between these components, capturing complex temporal dependencies and enabling accurate prediction of future energy consumption based on traffic and network load patterns. Experimental results demonstrate the effectiveness of the proposed STL-LSTM approach, outperforming traditional forecasting models and providing valuable insights into base station energy consumption dynamics.
更多查看译文
关键词
Base Station,STL,LSTM,Energy Prediction
AI 理解论文
溯源树
样例
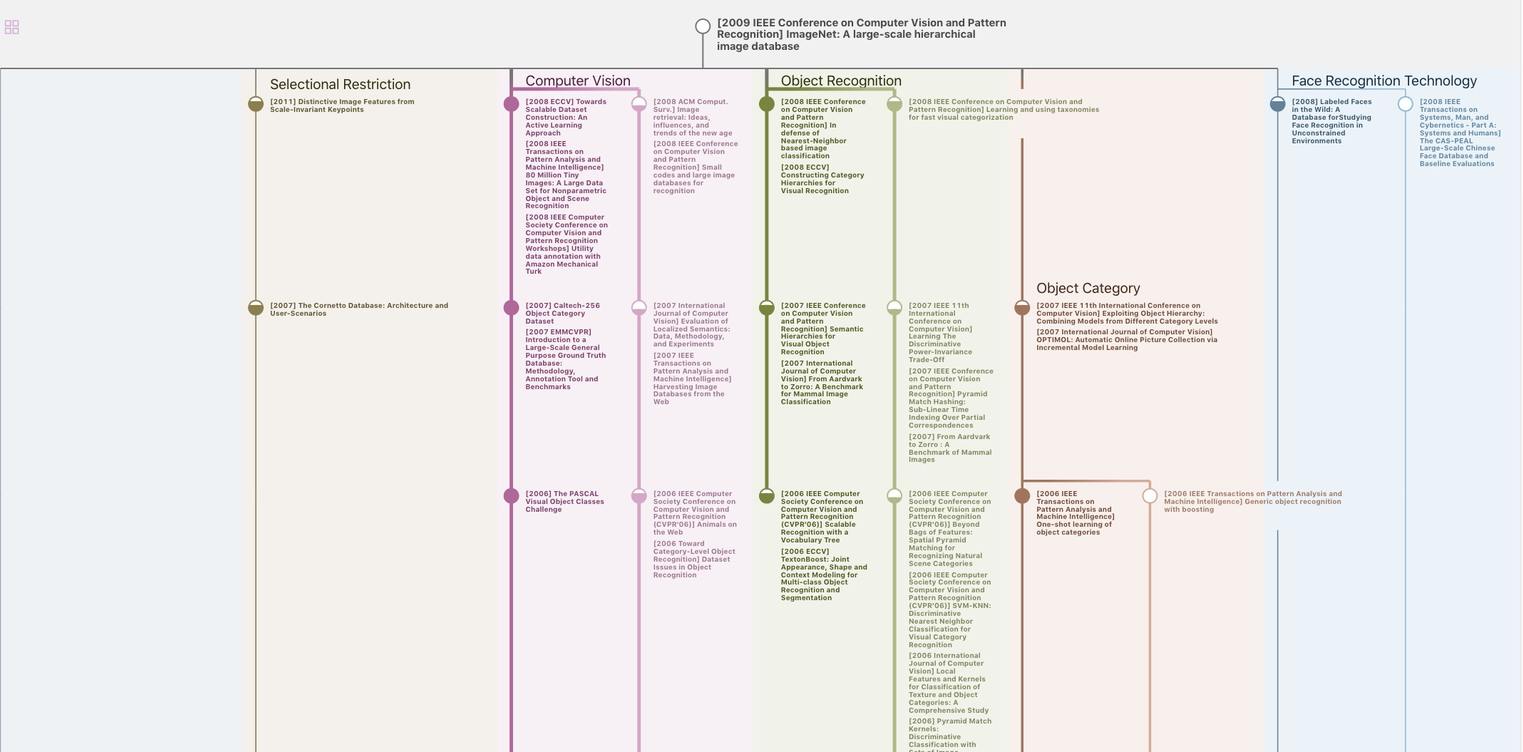
生成溯源树,研究论文发展脉络
Chat Paper
正在生成论文摘要